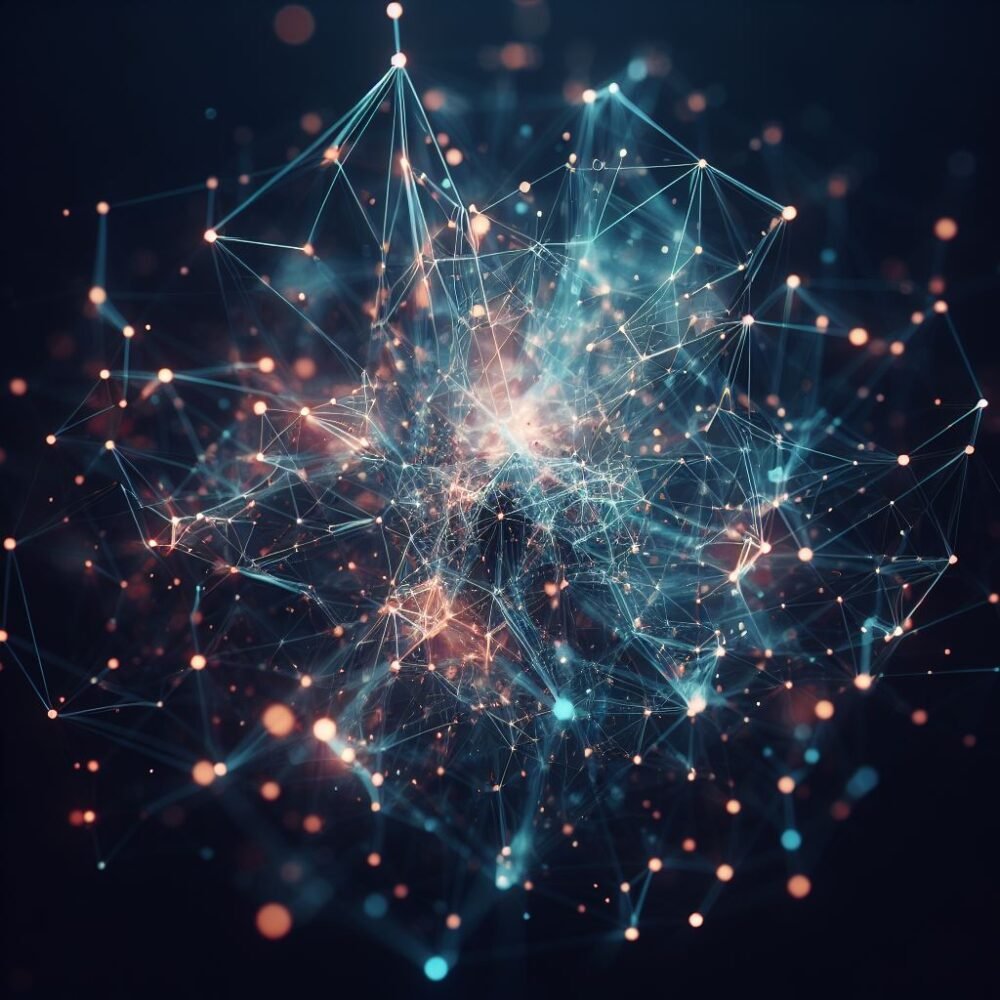
AI, or artificial intelligence, is the science and technology of creating machines and systems that can perform tasks that normally require human intelligence, such as learning, reasoning, decision-making, and problem-solving. AI is changing the world in many ways, from improving healthcare and education to enhancing security and entertainment to transforming business and industry. In this article, we will explore over 100 amazing AI applications that you need to know, with images and examples. We will also discuss the benefits and challenges of AI and the future of AI.
Introduction
AI is not a new concept. It has been around since the 1950s when the term was coined by John McCarthy, a computer scientist who defined it as “the science and engineering of making intelligent machines”. However, AI has gained a lot of momentum and popularity in recent years, thanks to advances in computing power, data availability, and algorithm development. AI is now everywhere, from our smartphones and laptops to our cars and homes, to our workplaces and schools. AI is also behind many of the products and services we use every day, such as search engines, social media, online shopping, music streaming, and video games.
What is AI
AI is not a single technology, but a broad field that encompasses many subfields. Some of the main subfields are:
- Machine learning: The study of algorithms and systems that can learn from data and improve their performance.
- Computer vision: The study that can understand and manipulate visual information, such as images and videos.
- Natural language processing: The study of algorithms and systems that can understand and generate natural language, such as speech and text.
- Speech recognition: The study of algorithms and systems that can recognize and transcribe spoken language.
- Natural language generation: The study of algorithms and systems that can generate natural language, such as text and speech.
- Natural language understanding: The study of algorithms and systems that can comprehend the meaning and intent of natural language, such as text and speech.
- Machine translation: The study of algorithms and systems that can translate natural language from one language to another.
- Computer graphics: The study of algorithms and systems that can create and manipulate visual content, such as images and videos.
- Robotics: The study of algorithms and systems that can control and interact with physical machines, such as robots and drones.
- Artificial neural networks: The study of algorithms and systems that are inspired by the structure and function of biological neural networks, such as the brain.
- Deep learning: The study of algorithms and systems that use artificial neural networks to perform complex tasks, such as image recognition, natural language processing, and speech recognition.
- Reinforcement learning: The study of algorithms and systems that can learn from their own actions and rewards, and optimize their behavior accordingly.
- Generative adversarial networks: The study of algorithms and systems that use two competing neural networks, one that generates data and one that evaluates data, to create realistic and novel data, such as images and videos.
Benefits of AI
AI has many benefits for humanity and society, such as:
- Improving efficiency and productivity
- Enhancing quality and accuracy
- Reducing costs and errors
- Saving time and resources
- Increasing safety and security
- Expanding knowledge and innovation
- Creating new opportunities and jobs
- Improving health and well-being
- Enriching entertainment and culture
- Solving global challenges and issues
AI Applications Challanges
However, AI also has some challenges and risks, such as:
- Ethical and moral dilemmas
- Social and economic impacts
- Privacy and security threats
- Bias and discrimination
- Accountability and transparency
- Human and machine interaction
- Regulation and governance
- Trust and reliability
- Education and skills
- Sustainability and environment
Therefore, it is important to develop and use AI responsibly, ethically, and sustainably, and to ensure that AI serves the common good and respects human values and rights.
AI has many applications in different industries and domains, such as healthcare, finance, manufacturing, retail, transportation, education, entertainment, and more. In the following sections, we will explore some of the most amazing and impactful AI applications that you need to know, with images and examples. We will also provide some links to free AI tools that you can use to experience AI yourself.
First AI Applications: AI in Healthcare
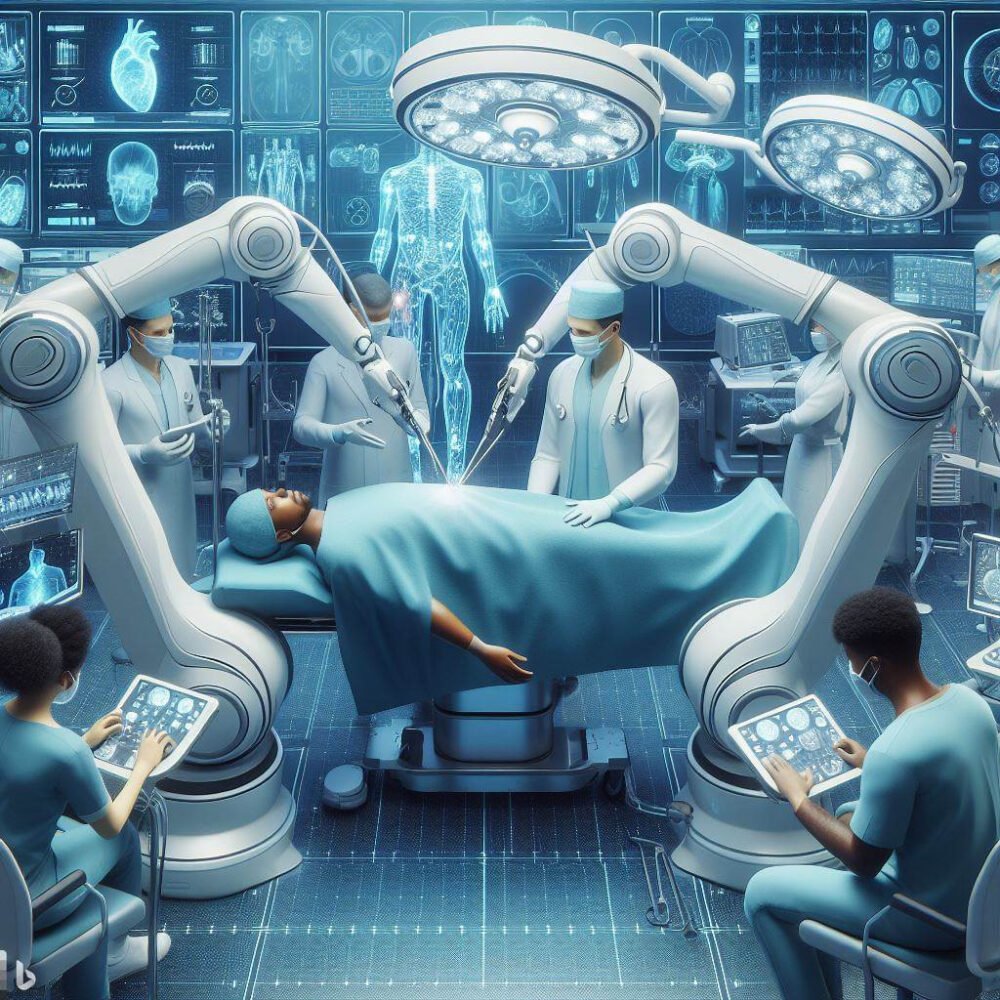
Healthcare is one of the most important and promising domains for AI applications. AI can help improve the quality and accessibility of healthcare, and save lives and costs. Some of the AI applications in healthcare are:
AI-Powered Diagnosis
AI can help identify diseases and medical conditions, such as cancer, diabetes, Alzheimer’s, and COVID-19, by analyzing various types of data, such as medical records, symptoms, tests, images, and genetics. For example, IBM Watson Health is an AI platform that can help diagnose and treat various diseases, such as cancer, diabetes, and heart disease, by using natural language processing and machine learning to analyze large amounts of data and provide evidence-based insights and recommendations.
AI-Powered Treatment Planning
AI can help develop personalized treatment plans for patients, based on their individual characteristics, preferences, and outcomes. For example, DeepMind is an AI company that has developed an AI system called AlphaFold, which can predict the 3D structure of proteins, which are essential for many biological processes and diseases. This can help design new drugs and treatments for various diseases, such as Alzheimer’s, Parkinson’s, and cystic fibrosis.
AI-Powered Drug Discovery
AI can help discover new drugs and treatments, by screening and synthesizing millions of potential molecules and finding the most effective and safe ones. For example, Insilico Medicine is an AI company that uses deep learning and generative adversarial networks to design new molecules for drug discovery and development and to test their efficacy and toxicity.
AI-Powered Medical Imaging
AI can help analyze medical images, such as X-rays, CT scans, MRI scans, and ultrasound, to identify diseases and medical conditions, such as tumors, fractures, and infections. For example, Arterys is an AI company that uses deep learning and cloud computing to provide fast and accurate analysis of medical images, such as cardiac MRI, lung CT, and liver MRI, and to provide insights and guidance for diagnosis and treatment.
AI-Powered Virtual Surgery
AI can help perform surgery remotely, using robotic devices that can be controlled by surgeons from anywhere in the world, and that can provide real-time feedback and assistance. For example, Intuitive Surgical is an AI company that develops and operates robotic surgical systems, such as the da Vinci system, which can perform minimally invasive surgery for various procedures, such as prostatectomy, hysterectomy, and cholecystectomy, and that can provide enhanced vision, precision, and control for surgeons.
AI-Powered Robotic Surgery
AI can help perform surgery using robotic devices that can operate autonomously or semi-autonomously, and that can adapt to the patient’s anatomy and physiology, and to the surgical environment. For example, Medtronic is an AI company that develops and operates robotic surgical systems, such as the Mazor X system, which can perform spine surgery, such as spinal fusion, and that can provide planning, guidance, and verification for surgeons.
AI-Powered Personalized Medicine
AI can help develop treatments tailored to the individual patient, based on their genetic, molecular, and environmental factors, and their response to previous treatments. For example, Tempus is an AI company that uses machine learning and natural language processing to collect and analyze large amounts of clinical and molecular data, such as genomic sequencing, and to provide personalized insights and recommendations for
AI-Powered Patient Monitoring
AI can help monitor patients’ health remotely, using sensors, wearables, and mobile devices, and alert them and their caregivers of any changes or issues. For example, Livongo is an AI company that uses machine learning and cloud computing to provide remote monitoring and coaching for patients with chronic conditions, such as diabetes, hypertension, and obesity, and to help them manage their health and lifestyle.
Healthcare Analytics
AI can help improve healthcare delivery and outcomes, by analyzing large amounts of data from various sources, such as electronic health records, claims, clinical trials, and research, and providing insights and predictions for healthcare providers, payers, researchers, and policymakers. For example, Flatiron Health is an AI company that uses natural language processing and machine learning to collect and analyze real-world data from cancer patients, and to provide insights and solutions for oncology research and care.
AI in healthcare is a rapidly growing and evolving field, with many opportunities and challenges. AI can help improve the quality and accessibility of healthcare, and save lives and costs. However, AI also poses some ethical and social issues, such as privacy, consent, accountability, bias, and trust. Therefore, it is important to develop and use AI in healthcare responsibly, ethically, and sustainably, and to ensure that AI respects human dignity and rights.
Second AI Applications: AI in Finance
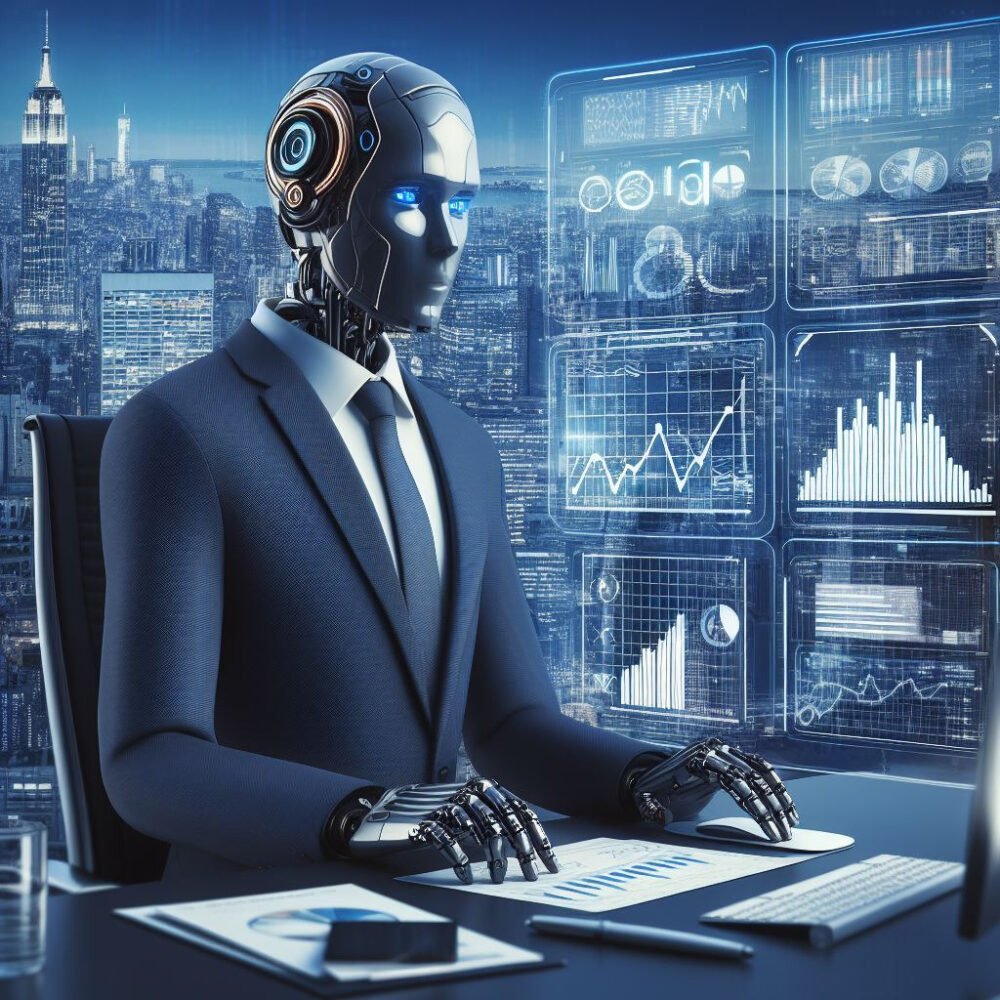
Finance is one of the most lucrative and competitive domains for AI applications. AI can help improve the efficiency and profitability of financial services, and reduce risks and costs. Some of the AI applications in finance are:
AI-Powered Fraud Detection
AI can help identify fraudulent transactions and activities, by analyzing various types of data, such as transaction history, customer behavior, location, device, and biometrics. For example, PayPal is an online payment service that uses machine learning and deep learning to detect and prevent fraud, by using neural networks to classify transactions as fraudulent or legitimate, and by using reinforcement learning to adapt to changing fraud patterns.
AI-Powered Risk Assessment
AI can help assess the risk of financial transactions and investments, by using various types of data, such as credit history, market trends, social media, and news. For example, Zest AI is an AI company that uses machine learning and natural language processing to provide risk assessment and credit scoring for lenders, by using explainable AI models that can provide transparent and fair decisions, and by using alternative data sources that can capture the creditworthiness of underserved customers.
AI-Powered Trading
AI can help make financial trades on behalf of clients, by using various types of data, such as market data, historical data, news, and sentiment. For example, Sentient is an AI company that uses evolutionary algorithms and deep learning to provide automated trading for investors, by using distributed AI systems that can evolve and optimize trading strategies, and by using natural language processing and sentiment analysis to capture market signals and trends.
AI-Powered Portfolio Management
AI can help manage clients’ investment portfolios, by using various types of data, such as client profile, risk appetite, financial goals, and market conditions. For example, Wealthfront is an online investment service that uses machine learning and robo-advisors to provide portfolio management for clients, by using automated algorithms that can allocate and rebalance funds, and by using personalized advice that can help clients achieve their financial objectives.
AI-Powered Customer Service
AI can help provide customer support for financial products and services, by using natural language processing and speech recognition to understand and respond to customer queries, and by using natural language generation and speech synthesis to generate natural and engaging responses. For example, Bank of America is a financial institution that uses natural language processing and machine learning to provide customer service through its virtual assistant, Erica, which can help customers with various tasks, such as checking balances, paying bills, transferring money, and getting financial advice.
AI-Powered Financial Planning
AI can help clients develop financial plans, by using various types of data, such as income, expenses, assets, liabilities, and goals. For example, Mint is an online financial service that uses machine learning and natural language processing to provide financial planning for clients, by using automated algorithms that can track and categorize transactions, and by using personalized recommendations that can help clients budget, save, and invest.
AI-Powered Compliance
AI can help ensure compliance with financial regulations, by using natural language processing and machine learning to analyze and interpret complex and dynamic rules, and by using natural language generation and machine learning to generate and verify reports and documents. For example, Ayasdi is an AI company that uses machine learning and natural language processing to provide compliance solutions for financial institutions, by using explainable AI models that can detect and prevent money laundering, fraud, and other financial crimes, and by using natural language generation and machine learning to generate and validate regulatory reports and filings.
AI-Powered Market Research
AI can help analyze market data to make informed financial decisions, by using natural language processing and machine learning to extract and synthesize relevant information from various sources, such as news, social media, and research papers. For example, AlphaSense is an AI company that uses natural language processing and machine learning to provide market research for financial professionals, by using semantic search and natural language understanding to find and analyze relevant data, and by using natural language generation and machine learning to summarize and visualize key insights and trends.
AI in finance is a rapidly growing and evolving field, with many opportunities and challenges. AI can help improve the efficiency and profitability of financial services, and reduce risks and costs. However, AI also poses some ethical and social issues, such as privacy, security, accountability, bias, and trust. Therefore, it is important to develop and use AI in finance responsibly, ethically, and sustainably, and to ensure that AI respects human dignity and rights.
Third AI Applications: AI in Manufacturing
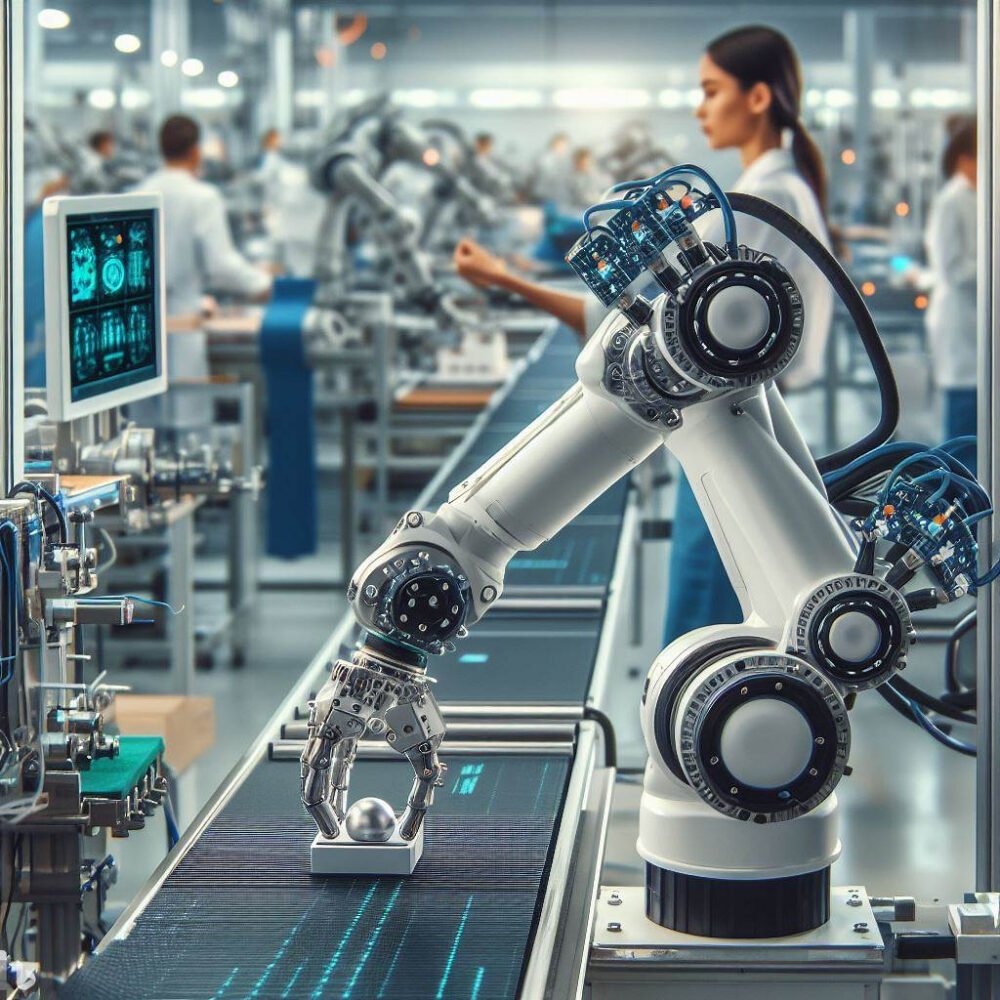
Manufacturing is one of the most traditional and vital domains for AI applications. AI can help improve the quality and efficiency of manufacturing processes, and reduce waste and costs. Some of the AI applications in manufacturing are:
AI-Powered Quality control
AI can help inspect products for defects, by using computer vision and machine learning to analyze images and videos of products, and by using natural language generation and machine learning to generate reports and feedback. For example, Instrumental is an AI company that uses computer vision and machine learning to provide quality control for manufacturers, by using cameras and sensors to capture and analyze images of products, and by using natural language generation and machine learning to provide insights and recommendations for improvement.
Predictive Maintenance
AI can help predict and prevent machine failures, by using machine learning and deep learning to analyze data from various sources, such as sensors, logs, and maintenance records, and by using natural language generation and machine learning to generate alerts and actions. For example, Uptake is an AI company that uses machine learning and deep learning to provide predictive maintenance for manufacturers, by using cloud computing and edge computing to collect and analyze data from machines, and uses natural language generation and machine learning to provide insights and solutions for maintenance and optimization.
AI-Powered Supply Chain Optimization
AI can help optimize the supply chain to reduce costs and improve efficiency, by using machine learning and deep learning to analyze data from various sources, such as inventory, demand, production, and delivery, and by using natural language generation and machine learning to generate plans and schedules. For example, ClearMetal is an AI company that uses machine learning and deep learning to provide supply chain optimization for manufacturers, by using cloud computing and blockchain to collect and analyze data from the supply chain, and by using natural language generation and machine learning to provide insights and predictions for supply chain management.
AI-Powered Product Design
AI can help design new products using AI-powered tools, such as generative design, which can generate multiple design options based on the user’s specifications and constraints, and allow the user to select and refine the best ones. For example, Autodesk is an AI company that develops and operates AI-powered tools for product design, such as Fusion 360, which can use generative design to create and optimize products, such as cars, bikes, and furniture, and that can use cloud computing and 3D printing to prototype and test products.
AI-Powered Production Planning
AI can help plan production schedules to meet demand and minimize costs, by using machine learning and deep learning to analyze data from various sources, such as orders, forecasts, resources, and constraints, and by using natural language generation and machine learning to generate plans and schedules. For example, LeanDNA is an AI company that uses machine learning and deep learning to provide production planning for manufacturers, by using cloud computing and analytics to collect and analyze data from the production process, and by using natural language generation and machine learning to provide insights and recommendations for production planning and execution.
Robotics
AI can help automate tasks using robots, by using computer vision and machine learning to enable robots to perceive and manipulate their environment, and by using reinforcement learning and deep learning to enable robots to learn and improve their skills. For example, Boston Dynamics is an AI company that develops and operates robots, such as Spot, Atlas, and Handle, which can perform various tasks, such as walking, running, jumping, lifting, and carrying, and that can use computer vision and machine learning to navigate and interact with their environment and use reinforcement learning and deep learning to learn and improve their behavior.
Process Automation
AI can help automate business processes, by using natural language processing and machine learning to understand and execute tasks, and by using natural language generation and machine learning to generate outputs and feedback. For example, UiPath is an AI company that uses natural language processing and machine learning to provide process automation for manufacturers, by using robotic process automation (RPA) to automate repetitive and rule-based tasks, such as data entry, invoice processing, and order fulfillment, and by using natural language generation and machine learning to provide insights and reports for process improvement.
AI-Powered Safety
AI can help improve safety in the workplace, by using computer vision and machine learning to monitor and detect hazards, and by using natural language generation and machine learning to generate alerts and actions. For example, Soter Analytics is an AI company that uses computer vision and machine learning to provide safety solutions for manufacturers, by using wearables and sensors to monitor and analyze workers’ movements and postures, and by using natural language generation and machine learning to provide feedback and coaching for injury prevention and reduction.
AI in manufacturing is a rapidly growing and evolving field, with many opportunities and challenges. AI can help improve the quality and efficiency of manufacturing processes, and reduce waste and costs. However, AI poses some ethical and social issues, such as privacy, security, accountability, bias, and trust. Therefore, it is important to develop and use AI in manufacturing responsibly, ethically, and sustainably, and to ensure that AI respects human dignity and rights.
Fourth AI Applications: AI in Retail
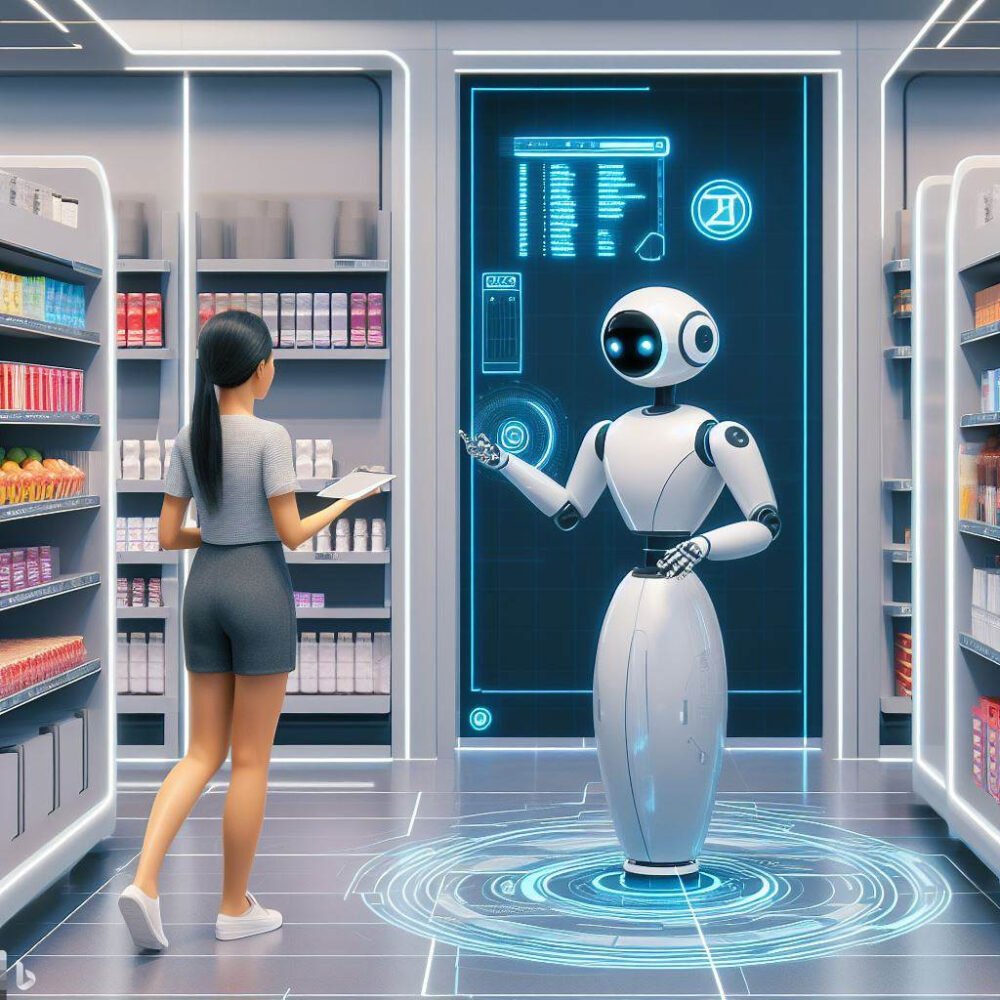
Retail is one of the most dynamic and customer-oriented domains for AI applications. AI can help improve the customer experience and satisfaction, and increase sales and revenue. Some of the AI applications in retail are:
AI-Powered Personalization
AI can help recommend products to customers based on their interests and purchase history, by using machine learning and deep learning to analyze data from various sources, such as browsing behavior, shopping cart, feedback, and social media. For example, Amazon is an online retailer that uses machine learning and deep learning to provide personalization for customers, by using recommender systems that can suggest products, books, movies, and music, and by using natural language processing and machine learning to provide voice-based shopping through its virtual assistant, Alexa.
Recommendation Engines
AI can help recommend products to customers based on their interests and purchase history, by using machine learning and deep learning to analyze data from various sources, such as browsing behavior, shopping cart, feedback, and social media. For example, Netflix is an online streaming service that uses machine learning and deep learning to provide recommendation engines for customers, by using collaborative filtering and content-based filtering to suggest movies and TV shows, and by using natural language processing and machine learning to provide personalized summaries and ratings for each title.
AI-Powered Inventory Management
AI can help track inventory levels and optimize restocking, by using machine learning and deep learning to analyze data from various sources, such as sales, demand, supply, and delivery. For example, Walmart is a retail giant that uses machine learning and deep learning to provide inventory management for its stores, by using cloud computing and IoT to collect and analyze data from its shelves, warehouses, and trucks, and by using natural language generation and machine learning to provide insights and actions for inventory optimization.
Demand forecasting
AI can help predict customer demand for products, by using machine learning and deep learning to analyze data from various sources, such as sales, trends, seasonality, and events. For example, Starbucks is a coffee chain that uses machine learning and deep learning to provide demand forecasting for its stores, by using cloud computing and analytics to collect and analyze data from its point-of-sale systems, mobile apps, and loyalty programs, and by using natural language generation and machine learning to provide insights and predictions for demand planning and allocation.
Customer Service
AI can help provide customer support for retail products and services, by using natural language processing and speech recognition to understand and respond to customer queries, and by using natural language generation and speech synthesis to generate natural and engaging responses. For example, Sephora is a beauty retailer that uses natural language processing and machine learning to provide customer service through its chatbot, which can help customers with various tasks, such as finding products, booking appointments, and getting beauty tips.
Fraud Detection
AI can help identify fraudulent transactions and activities, by using machine learning and deep learning to analyze data from various sources, such as transaction history, customer behavior, location, device, and biometrics. For example, Shopify is an online commerce platform that uses machine learning and deep learning to provide fraud detection for its merchants, by using cloud computing and analytics to collect and analyze data from its transactions, and by using natural language generation and machine learning to provide insights and recommendations for fraud prevention and mitigation.
Pricing Optimization
AI can help set prices to maximize profits, by using machine learning and deep learning to analyze data from various sources, such as demand, supply, competition, and customer behavior. For example, Uber is an online transportation service that uses machine learning and deep learning to provide pricing optimization for its drivers and riders, by using cloud computing and analytics to collect and analyze data from its trips, and by using natural language generation and machine learning to provide insights and predictions for dynamic pricing and surge pricing.
AI in retail is a rapidly growing and evolving field, with many opportunities and challenges. AI can help improve the customer experience and satisfaction, and increase sales and revenue. However, AI also poses some ethical and social issues, such as privacy, security, accountability, bias, and trust. Therefore, it is important to develop and use AI in retail responsibly, ethically, and sustainably, and to ensure that AI respects human dignity and rights.
Fifth AI Applications: AI in Education
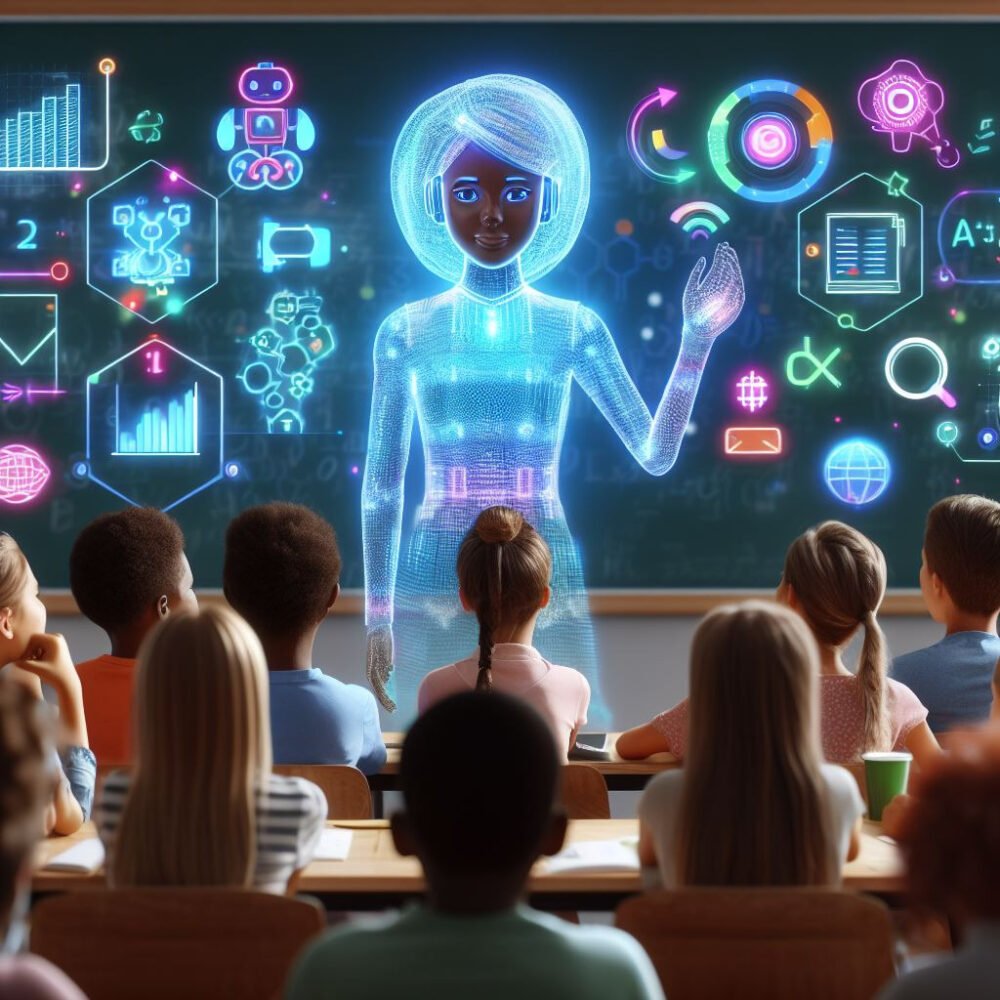
Education is one of the most fundamental and impactful domains for AI applications. AI can help improve the quality and accessibility of education, and enhance learning and teaching. Some of the AI applications in education are:
Personalized Learning
AI can help develop personalized learning plans for students, by using machine learning and deep learning to analyze data from various sources, such as learning styles, preferences, goals, and progress, and by using natural language generation and machine learning to generate adaptive and customized content and feedback. For example, Knewton is an AI company that uses machine learning and deep learning to provide personalized learning for students, by using cloud computing and analytics to collect and analyze data from students’ interactions with online courses, and by using natural language generation and machine learning to provide tailored and engaging content and feedback for each student.
AI-Powered Tutoring
AI can help provide tutoring assistance to students, by using natural language processing and speech recognition to understand and respond to student queries, and by using natural language generation and speech synthesis to generate natural and engaging responses. For example, Duolingo is an online language learning service that uses natural language processing and speech recognition to provide tutoring for students, by using chatbots and voice assistants that can converse with students in different languages, and by using natural language generation and speech synthesis to provide feedback and encouragement for students.
AI-Powered Assessment
AI can help develop and administer assessments to measure student learning, by using machine learning and deep learning to analyze data from various sources, such as quizzes, tests, essays, and projects, and by using natural language generation and machine learning to generate scores and feedback. For example, Pearson is an education company that uses machine learning and deep learning to provide assessment for students, by using cloud computing and analytics to collect and analyze data from students’ responses to online assessments, and by using natural language generation and machine learning to provide accurate and timely scores and feedback for students and teachers.
AI-Powered Language Learning
AI can help students learn new languages, by using natural language processing and speech recognition to understand and transcribe spoken language, and by using natural language generation and speech synthesis to generate spoken language. For example, Rosetta Stone is an online language learning service that uses natural language processing and speech recognition to provide language learning for students, by using interactive and immersive courses that can teach students vocabulary, grammar, and pronunciation, and by using natural language generation and speech synthesis to provide feedback and practice for students.
AI-Powered Creative Learning
AI can help students develop their creativity, by using machine learning and deep learning to generate creative content, such as poems, stories, code, essays, songs, and art, and by using natural language generation and machine learning to provide feedback and guidance for students. For example, OpenAI is an AI research organization that develops and operates AI-powered tools for creative learning, such as GPT-3, which can generate natural language text for various purposes, such as writing, summarizing, and answering, and DALL-E, which can generate art images for various prompts, such as drawing a cat wearing a hat.
AI-Powered Accessibility
AI can help make education more accessible to students with disabilities, by using natural language processing and speech recognition to provide speech-to-text and text-to-speech services, and by using computer vision and machine learning to provide image-to-text and text-to-image services. For example, Microsoft is a technology company that uses natural language processing and speech recognition to provide accessibility for students, by using cloud computing and AI to provide services such as Immersive Reader, which can read text aloud and highlight words and sentences, and Translator, which can translate text and speech from one language to another.
AI-Powered Educational Research
AI can help improve educational practices through research, by using machine learning and deep learning to analyze data from various sources, such as student performance, teacher feedback, and curriculum design, and by using natural language generation and machine learning to generate insights and recommendations for educators and policymakers. For example, LearnPlatform is an AI company that uses machine learning and deep learning to provide educational research for educators and policymakers, by using cloud computing and analytics to collect and analyze data from various educational technologies, and by using natural language generation and machine learning to provide insights and solutions for improving student outcomes and equity.
AI in education is a rapidly growing and evolving field, with many opportunities and challenges. AI can help improve the quality and accessibility of education, and enhance learning and teaching. However, AI also poses some ethical and social issues, such as privacy, security, accountability, bias, and trust. Therefore, it is important to develop and use AI in education responsibly, ethically, and sustainably, and to ensure that AI respects human dignity and rights.
Sixth AI Applications: AI in Transportation
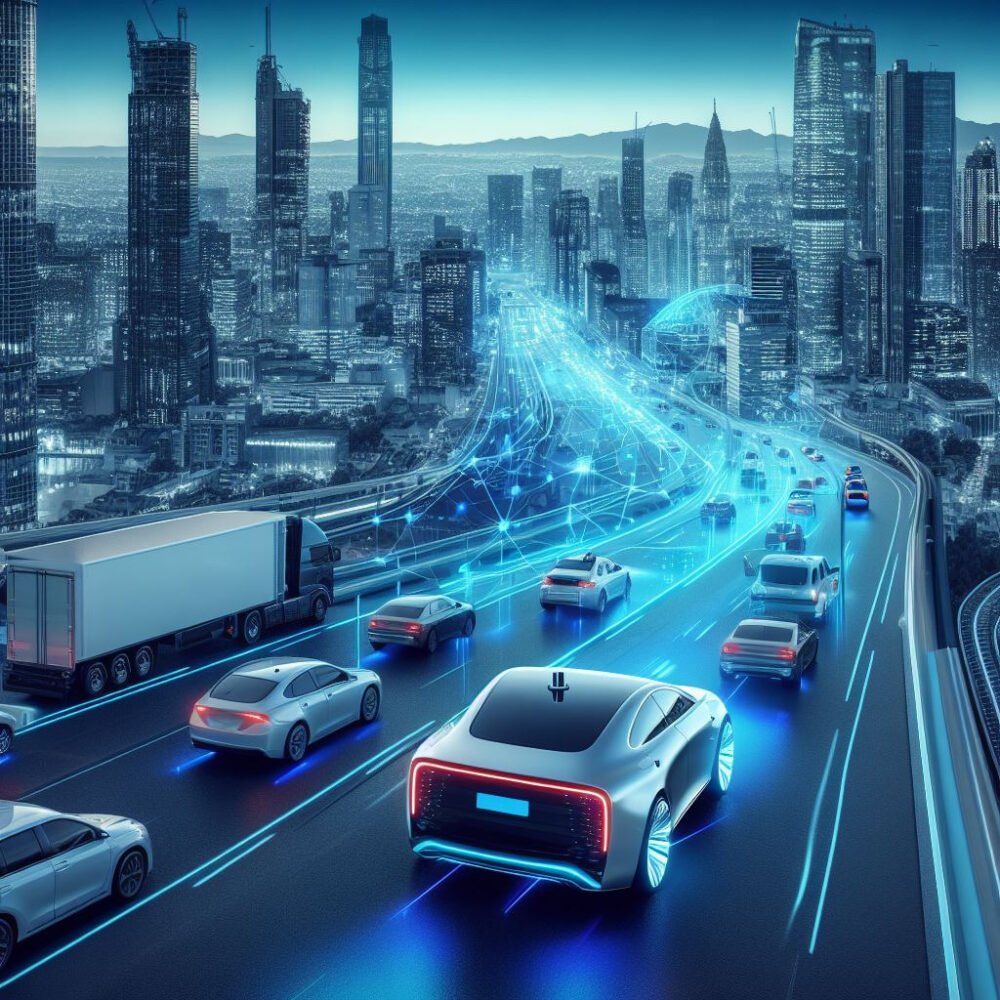
Transportation is one of the most essential and challenging domains for AI applications. AI can help improve the safety and efficiency of transportation systems, and reduce emissions and congestion. Some of the AI applications in transportation are:
Self-driving Cars
AI can help develop and operate self-driving cars, by using computer vision and machine learning to enable cars to perceive and navigate their environment, and by using reinforcement learning and deep learning to enable cars to learn and improve their driving skills. For example, Waymo is an AI company that develops and operates self-driving cars, by using lidar, radar, and cameras to collect and analyze data from the road, and by using neural networks and deep learning to control the car’s steering, acceleration, and braking.
Traffic Optimization
AI can help reduce traffic congestion and improve traffic flow, by using machine learning and deep learning to analyze data from various sources, such as traffic lights, sensors, cameras, and GPS, and by using natural language generation and machine learning to generate traffic signals and directions. For example, Rapid Flow Technologies is an AI company that uses machine learning and deep learning to provide traffic optimization for cities, by using cloud computing and IoT to collect and analyze data from traffic lights and sensors, and by using natural language generation and machine learning to provide adaptive traffic signals and real-time traffic information.
AI-Powered Fleet Management
AI can help manage fleets of vehicles more efficiently, by using machine learning and deep learning to analyze data from various sources, such as fuel consumption, maintenance, location, and driver behavior, and by using natural language generation and machine learning to generate reports and actions. For example, Samsara is an AI company that uses machine learning and deep learning to provide fleet management for businesses, by using cloud computing and IoT to collect and analyze data from vehicles and drivers, and by using natural language generation and machine learning to provide insights and solutions for fleet optimization and safety.
AI-Powered Public Transportation
AI can help improve the efficiency and effectiveness of public transportation systems, by using machine learning and deep learning to analyze data from various sources, such as ridership, demand, supply, and weather, and by using natural language generation and machine learning to generate schedules and routes. For example, Optibus is an AI company that uses machine learning and deep learning to provide public transportation for cities, by using cloud computing and analytics to collect and analyze data from buses and trains, and by using natural language generation and machine learning to provide optimal schedules and routes for public transportation.
AI-Powered Logistics
AI can help improve the efficiency of logistics operations, by using machine learning and deep learning to analyze data from various sources, such as orders, inventory, delivery, and customer feedback, and by using natural language generation and machine learning to generate plans and actions. For example, Flexport is an AI company that uses machine learning and deep learning to provide logistics for businesses, by using cloud computing and blockchain to collect and analyze data from the supply chain, and by using natural language generation and machine learning to provide insights and solutions for logistics management and optimization.
AI-Powered Aviation
AI can help improve the safety and efficiency of air travel, by using machine learning and deep learning to analyze data from various sources, such as flight data, weather, and air traffic, and by using natural language generation and machine learning to generate flight plans and actions. For example, Airbus is an aerospace company that uses machine learning and deep learning to provide aviation for airlines and passengers, by using cloud computing and IoT to collect and analyze data from aircraft and airports, and by using natural language generation and machine learning to provide insights and solutions for flight optimization and safety.
AI-Powered Maritime Transportation
AI can help improve the safety and efficiency of maritime transportation, by using machine learning and deep learning to analyze data from various sources, such as ship data, ocean data, and port data, and by using natural language generation and machine learning to generate ship plans and actions. For example, Wartsila is an AI company that uses machine learning and deep learning to provide maritime transportation for shipping companies and ports, by using cloud computing and IoT to collect and analyze data from ships and ports, and by using natural language generation and machine learning to provide insights and solutions for ship optimization and safety.
AI in transportation is a rapidly growing and evolving field, with many opportunities and challenges. AI can help improve the safety and efficiency of transportation systems, and reduce emissions and congestion. However, AI also poses some ethical and social issues, such as privacy, security, accountability, bias, and trust. Therefore, it is important to develop and use AI in transportation responsibly, ethically, and sustainably, and to ensure that AI respects human dignity and rights.
Seventh AI Applications: AI in Entertainment
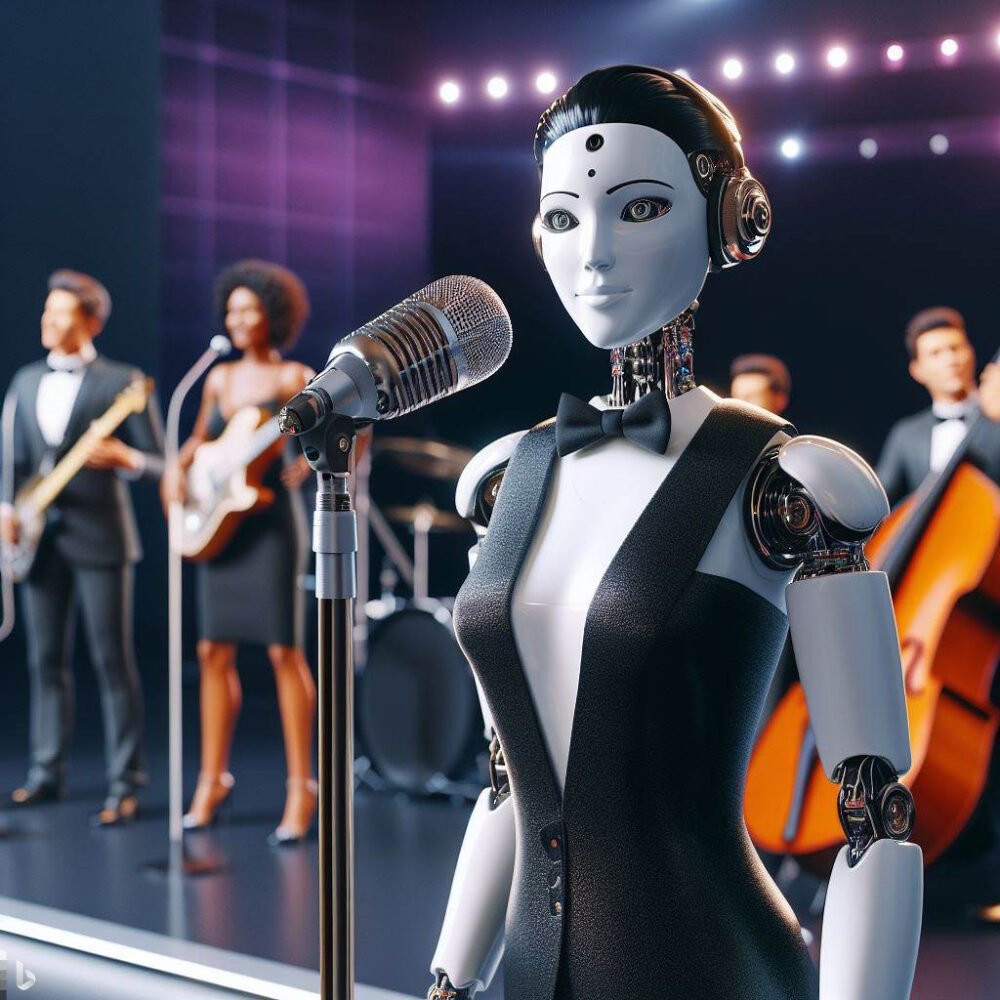
Entertainment is one of the most fun and creative domains for AI applications. AI can help create and distribute various forms of entertainment, such as games, music, movies, TV, and art. Some of the AI applications in entertainment are:
AI in Gaming
AI can help develop and operate video games, by using machine learning and deep learning to generate and optimize game content, such as levels, characters, and graphics, and by using reinforcement learning and deep learning to create and improve game agents, such as enemies, allies, and NPCs. For example, OpenAI is an AI research organization that develops and operates AI-powered games, such as OpenAI Five, which can play the popular game Dota 2, and OpenAI Codex, which can create and modify games using natural language commands.
AI in Music
AI can help create and distribute music, by using machine learning and deep learning to generate and synthesize music, such as melodies, harmonies, and lyrics, and by using natural language processing and machine learning to analyze and recommend music, such as genres, artists, and songs. For example, Spotify is an online music streaming service that uses machine learning and deep learning to provide music for listeners, by using recommender systems that can suggest music based on the listener’s preferences and behavior, and by using natural language processing and machine learning to provide personalized playlists and podcasts for each listener.
AI in Movies
AI can help produce and distribute movies, by using machine learning and deep learning to generate and edit movie content, such as scripts, dialogues, and scenes, and by using natural language processing and machine learning to analyze and recommend movies, such as genres, directors, and actors. For example, Netflix is an online streaming service that uses machine learning and deep learning to provide movies for viewers, by using collaborative filtering and content-based filtering to suggest movies based on the viewer’s preferences and behavior, and by using natural language processing and machine learning to provide personalized summaries and ratings for each movie.
AI in TV
AI can help produce and distribute TV shows, by using machine learning and deep learning to generate and edit TV content, such as scripts, dialogues, and scenes, and by using natural language processing and machine learning to analyze and recommend TV shows, such as genres, producers, and actors. For example, HBO is a TV network that uses machine learning and deep learning to provide TV shows for viewers, by using cloud computing and analytics to collect and analyze data from its shows and viewers, and by using natural language processing and machine learning to provide insights and predictions for TV production and distribution.
AI in Streaming
AI can help provide streaming services for movies, TV shows, music, and other content, by using machine learning and deep learning to optimize and personalize the streaming experience, such as quality, speed, and recommendations, and by using natural language processing and machine learning to provide interactive and engaging features, such as voice control, chat, and social media. For example, YouTube is an online video streaming service that uses machine learning and deep learning to provide streaming for viewers, by using cloud computing and edge computing to collect and analyze data from its videos and viewers, and by using natural language processing and machine learning to provide features such as captions, translations, comments, and likes.
AI in Social Media
AI can help develop and operate social media platforms, by using machine learning and deep learning to generate and optimize social media content, such as posts, stories, and filters, and by using natural language processing and machine learning to analyze and recommend social media content, such as friends, groups, and trends. For example, Facebook is a social media platform that uses machine learning and deep learning to provide social media for users, by using cloud computing and analytics to collect and analyze data from its users and content, and by using natural language processing and machine learning to provide features such as news feed, messenger, marketplace, and groups.
AI in Creative Writing
AI can help generate creative text formats, such as poems, stories, code, essays, songs, and more, by using machine learning and deep learning to generate and synthesize natural language text, based on the user’s input and preferences. For example, OpenAI is an AI research organization that develops and operates AI-powered tools for creative writing, such as GPT-3, which can generate natural language text for various purposes, such as writing, summarizing, and answering, and DALL-E, which can generate art images for various prompts, such as drawing a cat wearing a hat.
Art Generation
AI can help generate art images, such as paintings, drawings, and cartoons, by using machine learning and deep learning to generate and synthesize visual content, based on the user’s input and preferences. For example, Artbreeder is an online service that uses machine learning and deep learning to provide art generation for users, by using generative adversarial networks (GANs) that can create and modify images, such as portraits, landscapes, and animals, and by using natural language processing and machine learning to provide features such as tags, ratings, and comments.
AI in entertainment is a rapidly growing and evolving field, with many opportunities and challenges. AI can help create and distribute various forms of entertainment, such as games, music, movies, TV, and art. However, AI also poses some ethical and social issues, such as privacy, security, accountability, bias, and trust. Therefore, it is important to develop and use AI in entertainment responsibly, ethically, and sustainably, and to ensure that AI respects human dignity and rights.
Eighth AI Applications: AI in Legal
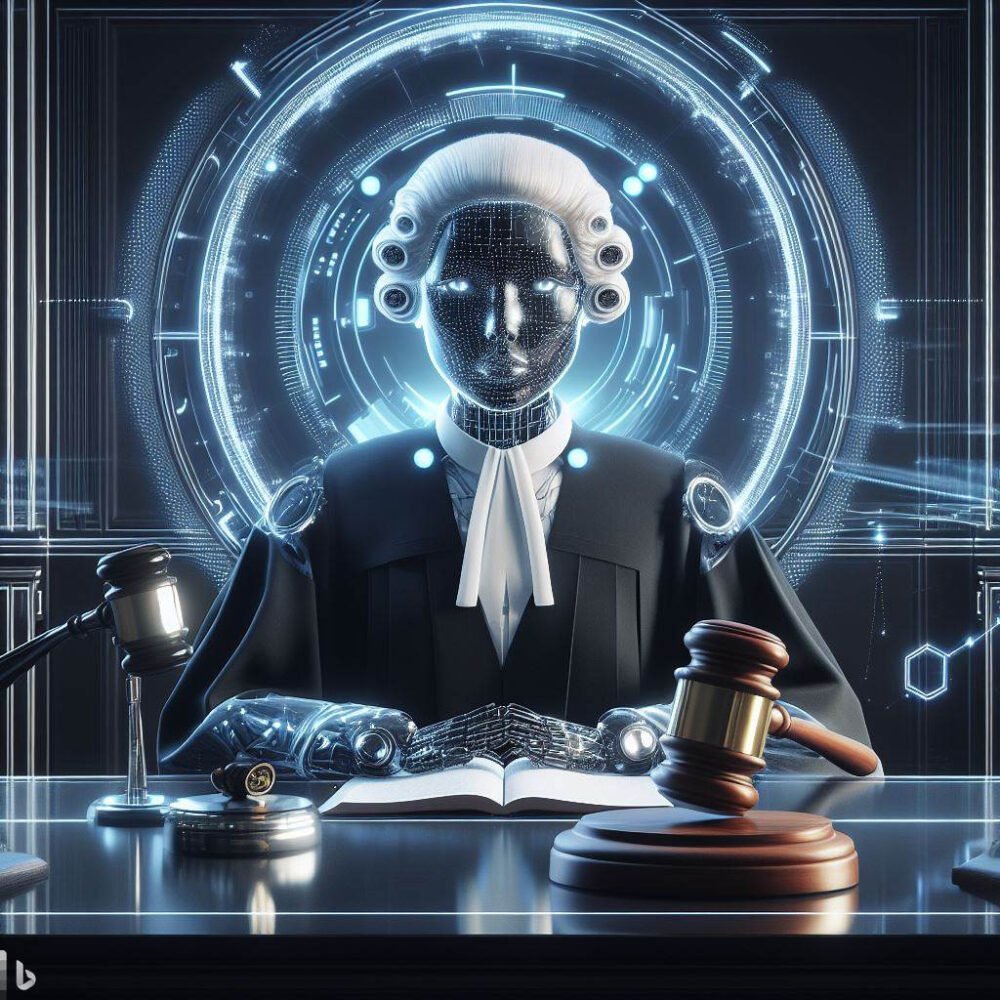
Legal is one of the most complex and critical domains for AI applications. AI can help improve the quality and efficiency of legal services, and reduce costs and errors. Some of the AI applications in legal are:
AI in Legal Research
AI can help conduct legal research, by using natural language processing and machine learning to analyze and interpret large amounts of legal data, such as statutes, cases, regulations, and contracts, and by using natural language generation and machine learning to generate summaries and insights for lawyers and clients. For example, ROSS Intelligence is an AI company that uses natural language processing and machine learning to provide legal research for lawyers and clients, by using cloud computing and analytics to collect and analyze data from various legal sources, and by using natural language generation and machine learning to provide answers and explanations for legal questions.
AI in Document Review
AI can help review documents for legal significance, by using natural language processing and machine learning to identify and extract relevant information, such as facts, issues, and clauses, and by using natural language generation and machine learning to generate reports and feedback for lawyers and clients. For example, Kira Systems is an AI company that uses natural language processing and machine learning to provide document review for lawyers and clients, by using cloud computing and analytics to collect and analyze data from various legal documents, such as contracts, leases, and agreements, and by using natural language generation and machine learning to provide insights and recommendations for document analysis and management.
AI in Contract Analysis
AI can help analyze contracts for legal risks and obligations, by using natural language processing and machine learning to identify and extract relevant information, such as terms, conditions, and deadlines, and by using natural language generation and machine learning to generate reports and feedback for lawyers and clients. For example, LawGeex is an AI company that uses natural language processing and machine learning to provide contract analysis for lawyers and clients, by using cloud computing and analytics to collect and analyze data from various contracts, such as employment contracts, sales contracts, and service contracts, and by using natural language generation and machine learning to provide insights and recommendations for contract review and approval.
AI in Due Diligence
AI can help conduct due diligence on businesses and individuals, by using natural language processing and machine learning to analyze and verify large amounts of data, such as financial records, legal records, and social media, and by using natural language generation and machine learning to generate reports and feedback for lawyers and clients. For example, Luminance is an AI company that uses natural language processing and machine learning to provide due diligence for lawyers and clients, by using cloud computing and analytics to collect and analyze data from various sources, such as corporate documents, regulatory filings, and news articles, and by using natural language generation and machine learning to provide insights and recommendations for due diligence and compliance.
Predictive Analytics
AI can help predict the outcome of legal cases, by using machine learning and deep learning to analyze and model large amounts of data, such as case history, evidence, and arguments, and by using natural language generation and machine learning to generate predictions and probabilities for lawyers and clients. For example, Lex Machina is an AI company that uses machine learning and deep learning to provide predictive analytics for lawyers and clients, by using cloud computing and analytics to collect and analyze data from various legal sources, such as court records, dockets, and opinions, and by using natural language generation and machine learning to provide insights and predictions for litigation strategy and outcomes.
AI in Legal Advice
AI can help provide legal advice to clients, by using natural language processing and speech recognition to understand and respond to client queries, and by using natural language generation and speech synthesis to generate natural and engaging responses. For example, DoNotPay is an online service that uses natural language processing and speech recognition to provide legal advice for clients, by using chatbots and voice assistants that can converse with clients in natural language, and use natural language generation and speech synthesis to provide feedback and guidance for various legal issues, such as parking tickets, flight delays, and consumer rights.
AI in Case Management
AI can help manage legal cases, by using machine learning and deep learning to organize and optimize large amounts of data, such as documents, tasks, and deadlines, and by using natural language generation and machine learning to generate reports and feedback for lawyers and clients. For example, Clio is an online service that uses machine learning and deep learning to provide case management for lawyers and clients, by using cloud computing and analytics to collect and analyze data from various legal sources, such as emails, calendars, and invoices, and by using natural language generation and machine learning to provide insights and recommendations for case management and collaboration.
AI in legal is a rapidly growing and evolving field, with many opportunities and challenges. AI can help improve the quality and efficiency of legal services, and reduce costs and errors. However, AI poses ethical and social issues, such as privacy, security, accountability, bias, and trust. Therefore, it is important to develop and use AI legally responsibly, ethically, and sustainably, and to ensure that AI respects human dignity and rights.
Nineth AI Applications: AI in Government
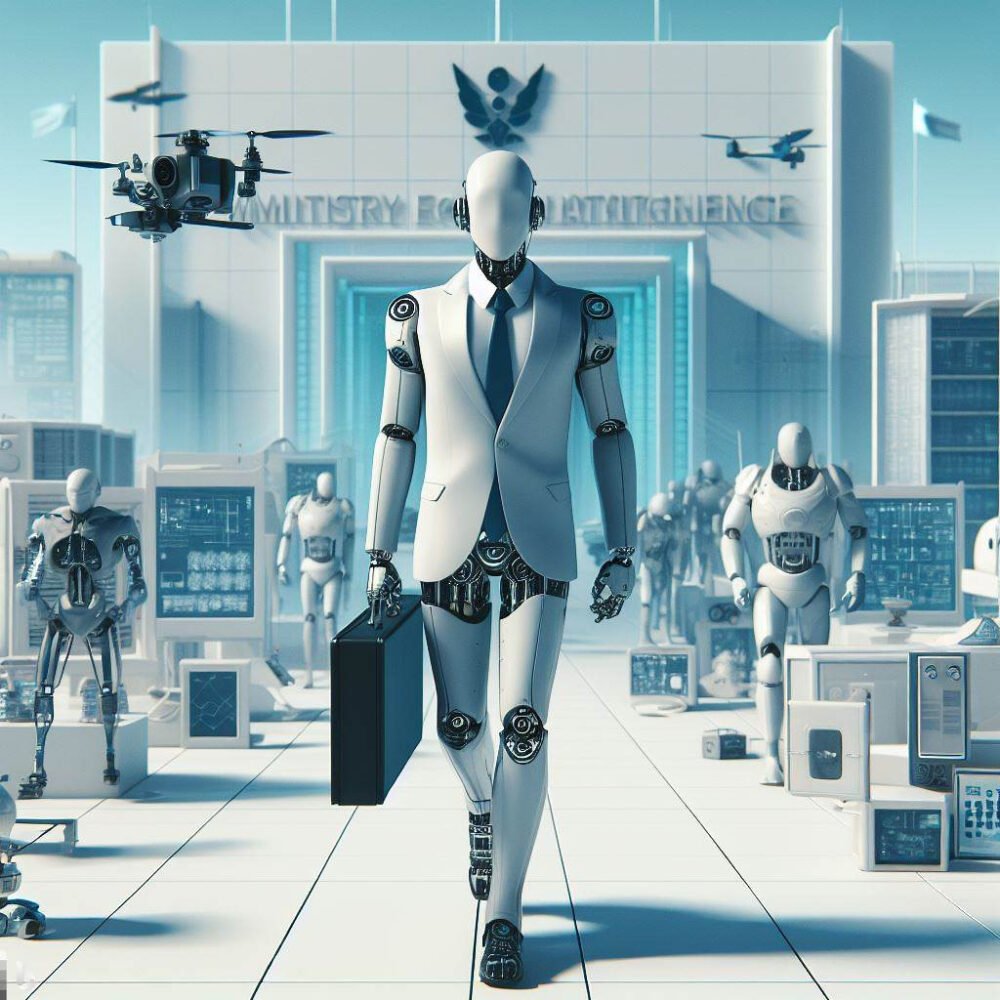
Government is one of the most essential and impactful domains for AI applications. AI can help improve the quality and accessibility of government services, and enhance public policy objectives. Some of the AI applications in government are:
AI in Public Safety
AI can help protect and serve the public, by using machine learning and deep learning to analyze data from various sources, such as cameras, sensors, and social media, and by using natural language generation and machine learning to generate alerts and actions for public safety officials and citizens. For example, SoundThinking is an AI company that uses machine learning and deep learning to provide public safety for public safety officials and citizens, by using cloud computing and IoT to collect and analyze data from acoustic sensors, and by using natural language generation and machine learning to provide insights and notifications for gunshot detection and location.
AI in Health and Welfare
AI can help provide health and welfare services, by using machine learning and deep learning to analyze data from various sources, such as medical records, tests, images, and symptoms, and by using natural language generation and machine learning to generate reports and feedback for health and welfare providers and beneficiaries. For example, Babylon Health is an online healthcare service that uses natural language processing and speech recognition to provide health and welfare services for health and welfare providers and beneficiaries, by using chatbots and voice assistants that can converse with users in natural language, and by using natural language generation and speech synthesis to provide feedback and guidance for various health issues, such as symptoms, diagnosis, and treatment.
AI in Education
AI can help provide education services, by using machine learning and deep learning to create and optimize educational content, such as courses, lessons, and feedback, and by using natural language processing and speech recognition to understand and respond to student queries, and by using natural language generation and speech synthesis to generate natural and engaging responses. For example, Duolingo is an online learning platform that uses machine learning and deep learning to provide education services for students and teachers, by using cloud computing and analytics to collect and analyze data from various sources, such as user preferences, progress, and performance, and by using natural language processing and speech recognition to provide features such as Duolingo Bots, which can converse with users in different languages, and Duolingo Stories, which can provide interactive and immersive stories for language learning.
AI in Transportation
AI can help provide transportation services, by using machine learning and deep learning to optimize and personalize the transportation experience, such as routes, schedules, and fares, and by using natural language processing and machine learning to provide interactive and engaging features, such as voice control, chat, and social media. For example, Uber is an online transportation service that uses machine learning and deep learning to provide transportation services for drivers and riders, by using cloud computing and edge computing to collect and analyze data from various sources, such as GPS, sensors, and maps, and by using natural language processing and machine learning to provide features such as Uber Pool, which can match riders with similar destinations, and Uber Eats, which can deliver food from local restaurants.
AI in Taxation
AI can help provide taxation services, by using machine learning and deep learning to analyze and verify data from various sources, such as income, expenses, and deductions, and by using natural language generation and machine learning to generate reports and feedback for taxpayers and tax authorities. For example, TurboTax is an online tax preparation service that uses machine learning and deep learning to provide taxation services for taxpayers and tax authorities, by using cloud computing and analytics to collect and analyze data from various sources, such as financial records, legal records, and social media, and by using natural language generation and machine learning to provide insights and recommendations for tax filing and refund.
AI in Legal
AI can help provide legal services, by using natural language processing and natural language generation to analyze and interpret large amounts of legal data, such as statutes, cases, regulations, and contracts, and by using natural language generation and machine learning to generate summaries and insights for lawyers and clients. For example, Kira Systems is an AI company that uses natural language processing and machine learning to provide legal services for lawyers and clients, by using cloud computing and analytics to collect and analyze data from various legal sources, such as court records, dockets, and opinions, and by using natural language generation and machine learning to provide insights and predictions for litigation strategy and outcomes.
AI in government is a rapidly growing and evolving field, with many opportunities and challenges. AI can help improve the quality and accessibility of government services, and enhance public policy objectives. However, AI also poses some ethical and social issues, such as privacy, security, accountability, bias, and trust. Therefore, it is important to develop and use AI in government responsibly, ethically, and sustainably, and to ensure that AI respects human dignity and rights.
Tenth AI Applications: AI in Agriculture
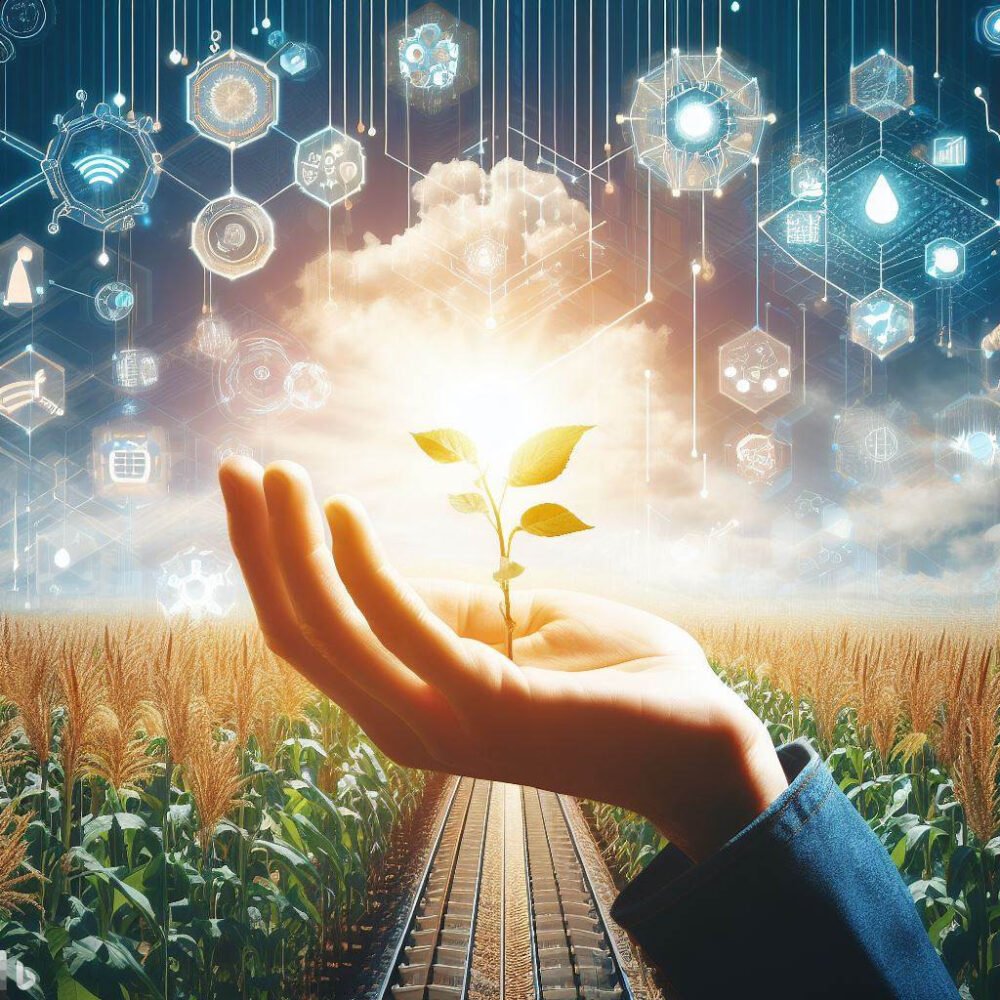
Agriculture is one of the most ancient and important domains for AI applications. AI can help improve the productivity and sustainability of agriculture, and reduce environmental impact and food waste. Some of the AI applications in agriculture are:
Crop Monitoring
AI can help monitor crop health and growth, by using machine learning and deep learning to analyze data from various sources, such as satellites, drones, sensors, and cameras, and by using natural language generation and machine learning to generate reports and feedback for farmers and agronomists. For example, Farmers Edge is an AI company that uses machine learning and deep learning to provide crop monitoring for farmers and agronomists, by using cloud computing and IoT to collect and analyze data from various sources, such as weather, soil, and crop, and by using natural language generation and machine learning to provide insights and recommendations for crop management and optimization.
Pest and Disease Detection
AI can help detect and prevent pest and disease outbreaks, by using computer vision and machine learning to analyze images and videos of crops and livestock, and by using natural language generation and machine learning to generate alerts and actions for farmers and veterinarians. For example, Plantix is an AI company that uses computer vision and machine learning to provide pest and disease detection for farmers and agronomists, by using cloud computing and edge computing to collect and analyze data from images of crops, and by using natural language generation and machine learning to provide diagnosis and treatment for crop diseases and pests.
Precision Farming
AI can help optimize farming inputs and outputs, by using machine learning and deep learning to analyze data from various sources, such as soil, water, fertilizer, and seeds, and by using natural language generation and machine learning to generate plans and actions for farmers and agronomists. For example, The Climate Corporation is an AI company that uses machine learning and deep learning to provide precision farming for farmers and agronomists, by using cloud computing and analytics to collect and analyze data from various sources, such as weather, soil, and crop, and by using natural language generation and machine learning to provide insights and recommendations for farming decisions and practices.
AI in Irrigation Management
AI can help manage irrigation systems more efficiently, by using machine learning and deep learning to analyze data from various sources, such as soil, water, and crop, and by using natural language generation and machine learning to generate plans and actions for farmers and agronomists. For example, CropX is an AI company that uses machine learning and deep learning to provide irrigation management for farmers and agronomists, by using cloud computing and IoT to collect and analyze data from soil sensors, and by using natural language generation and machine learning to provide insights and recommendations for irrigation scheduling and control.
AI-Powered Livestock Management
AI can help monitor and improve livestock health and welfare, by using machine learning and deep learning to analyze data from various sources, such as sensors, wearables, and cameras, and by using natural language generation and machine learning to generate reports and feedback for farmers and veterinarians. For example, Connecterra is an AI company that uses machine learning and deep learning to provide livestock management for farmers and veterinarians, by using cloud computing and IoT to collect and analyze data from sensors and wearables attached to cows, and by using natural language generation and machine learning to provide insights and recommendations for cow health and behavior.
AI in Harvesting
AI can help automate harvesting tasks, by using computer vision and machine learning to enable robots to perceive and manipulate crops, and by using reinforcement learning and deep learning to enable robots to learn and improve their skills. For example, Abundant Robotics is an AI company that develops and operates robots for harvesting, such as apple-picking robots, which can use computer vision and machine learning to locate and pick apples from trees and use reinforcement learning and deep learning to learn and improve their performance.
Food processing: AI can help improve the quality and safety of food processing, by using computer vision and machine learning to analyze images and videos of food products, such as fruits, vegetables, and meat, and by using natural language generation and machine learning to generate reports and feedback for food processors and inspectors. For example, Intello Labs is an AI company that uses computer vision and machine learning to provide food processing for food processors and inspectors, by using cloud computing and edge computing to collect and analyze data from images of food products, and by using natural language generation and machine learning to provide insights and recommendations for food quality and grading.
AI in agriculture is a rapidly growing and evolving field, with many opportunities and challenges. AI can help improve the productivity and sustainability of agriculture, and reduce environmental impact and food waste. However, AI also poses some ethical and social issues, such as privacy, security, accountability, bias, and trust. Therefore, it is important to develop and use AI in agriculture responsibly, ethically, and sustainably, and to ensure that AI respects human dignity and rights.
Eleventh AI Applications: AI in Energy
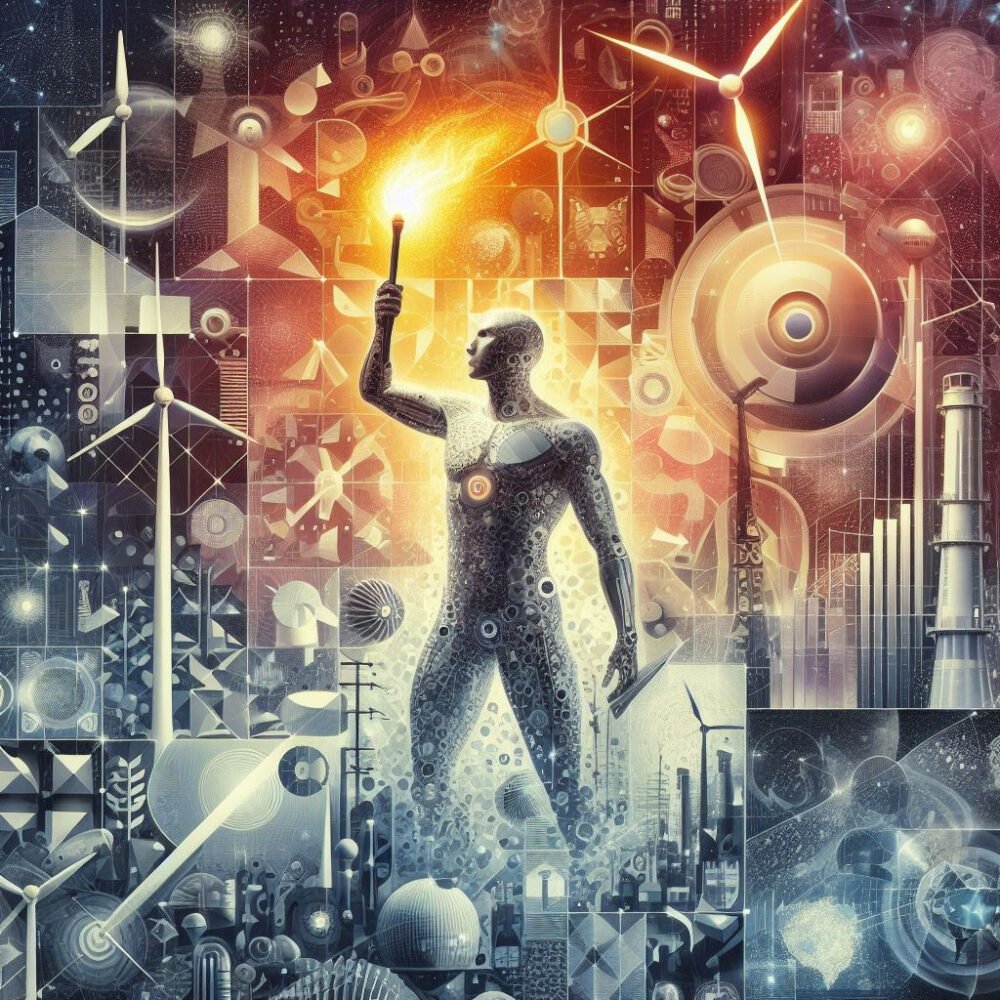
Energy is one of the most vital and challenging domains for AI applications. AI can help improve the efficiency and sustainability of energy systems, and reduce emissions and costs. Some of the AI applications in energy are:
Demand Forecasting
AI can help predict the demand for energy, by using machine learning and deep learning to analyze data from various sources, such as weather, time, season, and events, and by using natural language generation and machine learning to generate forecasts and insights for energy providers and consumers. For example, Google is a technology company that uses machine learning and deep learning to provide demand forecasting for its data centers, by using cloud computing and analytics to collect and analyze data from various sources, such as weather, traffic, and user behavior, and by using natural language generation and machine learning to provide insights and recommendations for energy optimization and management.
AI in Renewable Energy
AI can help integrate and optimize renewable energy sources, such as solar, wind, and hydro, by using machine learning and deep learning to analyze data from various sources, such as sensors, cameras, and satellites, and by using natural language generation and machine learning to generate plans and actions for energy providers and consumers. For example, DeepMind is an AI company that uses machine learning and deep learning to provide renewable energy for its partner, Google, by using cloud computing and analytics to collect and analyze data from various sources, such as wind farms, solar panels, and batteries, and by using natural language generation and machine learning to provide insights and predictions for renewable energy production and consumption.
Smart Grid
AI can help create and operate smart grids, which are networks of energy devices and systems that can communicate and coordinate with each other, by using machine learning and deep learning to analyze data from various sources, such as meters, switches, and transformers, and by using natural language generation and machine learning to generate signals and commands for energy devices and systems. For example, IBM is a technology company that uses machine learning and deep learning to provide smart grid for its clients, such as American Electric Power, by using cloud computing and IoT to collect and analyze data from various sources, such as smart meters, smart appliances, and smart vehicles, and by using natural language generation and machine learning to provide insights and solutions for smart grid management and control.
AI in Energy Efficiency
AI can help improve the energy efficiency of buildings and industries, by using machine learning and deep learning to analyze data from various sources, such as sensors, thermostats, and cameras, and by using natural language generation and machine learning to generate reports and feedback for building and industry owners and operators. For example, Carbon Lighthouse is an AI company that uses machine learning and deep learning to provide energy efficiency for buildings and industries, by using cloud computing and IoT to collect and analyze data from various sources, such as HVAC systems, lighting systems, and occupancy sensors, and by using natural language generation and machine learning to provide insights and recommendations for energy efficiency and savings.
AI in Electric Vehicles
AI can help develop and operate electric vehicles, which are vehicles that use electricity as their primary source of power, by using machine learning and deep learning to analyze data from various sources, such as batteries, motors, and chargers, and by using natural language generation and machine learning to generate reports and feedback for vehicle owners and drivers. For example, Tesla is an AI company that develops and operates electric vehicles, by using cloud computing and edge computing to collect and analyze data from various sources, such as cameras, radars, and ultrasonic sensors, and by using natural language generation and machine learning to provide features such as Autopilot, which can assist drivers with steering, acceleration, and braking.
AI in energy is a rapidly growing and evolving field, with many opportunities and challenges. AI can help improve the efficiency and sustainability of energy systems, and reduce emissions and costs. However, AI poses ethical and social issues, such as privacy, security, accountability, bias, and trust. Therefore, it is important to develop and use AI in energy responsibly, ethically, and sustainably, and to ensure that AI respects human dignity and rights.
Twelveth AI Applications: AI in Environment
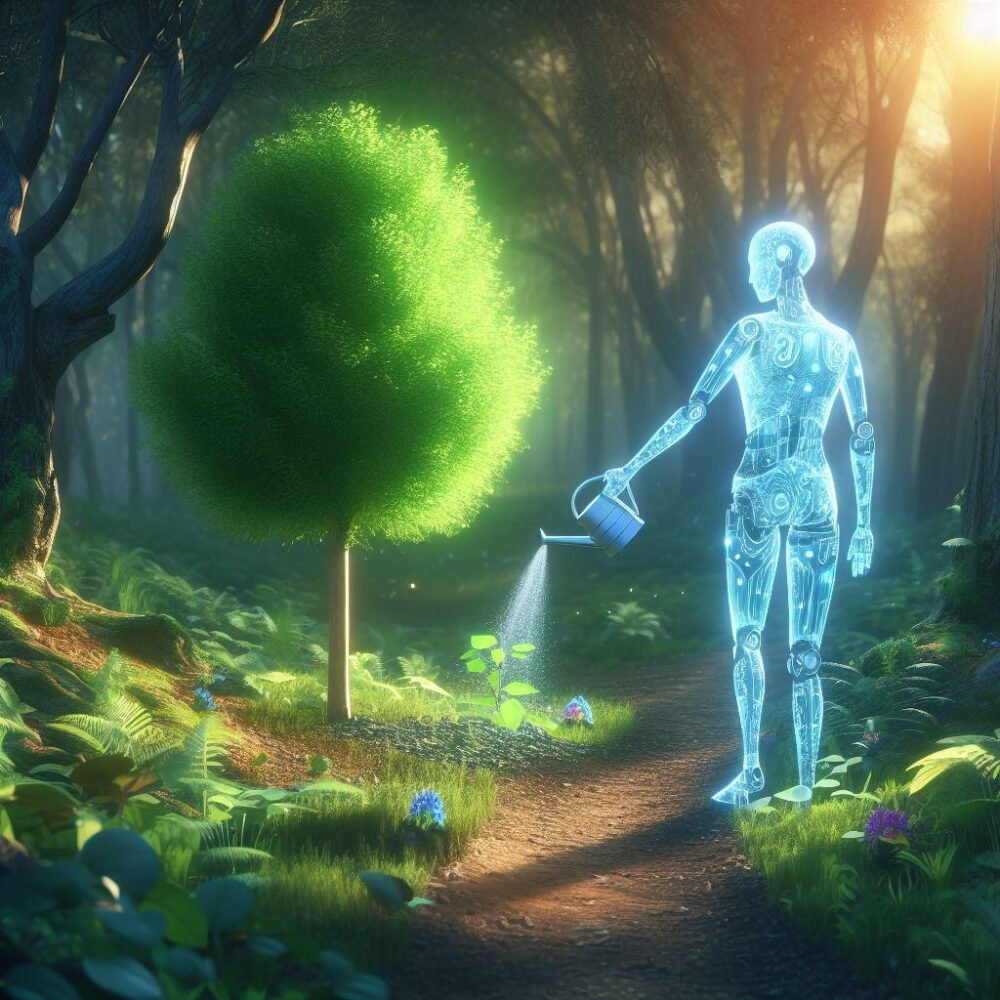
The environment is one of the most urgent and crucial domains for AI applications. AI can help protect and restore the environment, and mitigate the effects of climate change. Some of the AI applications in the environment are:
AI in Climate Change
AI can help monitor and model the causes and consequences of climate change, by using machine learning and deep learning to analyze data from various sources, such as satellites, sensors, and historical records, and by using natural language generation and machine learning to generate reports and insights for scientists and policymakers. For example, Microsoft AI for Earth is an AI initiative that uses machine learning and deep learning to provide climate change solutions for scientists and policymakers, by using cloud computing and analytics to collect and analyze data from various sources, such as weather, land use, and emissions, and by using natural language generation and machine learning to provide insights and predictions for climate change impacts and actions. You can read more about AI in Climate Change.
AI in Biodiversity
AI can help conserve and restore biodiversity, by using computer vision and machine learning to analyze images and videos of wildlife and habitats, and by using natural language generation and machine learning to generate reports and feedback for conservationists and ecologists. For example, WildMe is an AI platform that uses computer vision and machine learning to provide biodiversity conservation for conservationists and ecologists, by using cloud computing and edge computing to collect and analyze data from images and videos of wildlife, and by using natural language generation and machine learning to provide insights and recommendations for wildlife identification and tracking.
AI in Pollution
AI can help detect and reduce pollution, by using machine learning and deep learning to analyze data from various sources, such as sensors, cameras, and social media, and by using natural language generation and machine learning to generate alerts and actions for citizens and authorities. For example, BreezoMeter is an AI company that uses machine learning and deep learning to provide pollution detection and reduction for citizens and authorities, by using cloud computing and IoT to collect and analyze data from various sources, such as air quality sensors, traffic data, and weather data, and by using natural language generation and machine learning to provide insights and recommendations for pollution levels and exposure.
AI in Waste Management
AI can help manage waste more efficiently, by using computer vision and machine learning to identify and sort waste, and by using natural language generation and machine learning to generate reports and feedback for waste collectors and recyclers. For example, AMP Robotics is an AI company that uses computer vision and machine learning to provide waste management for waste collectors and recyclers, by using cloud computing and robotics to collect and analyze data from images of waste, and by using natural language generation and machine learning to provide insights and recommendations for waste classification and recycling.
AI in Forest Management
AI can help manage forests more sustainably, by using machine learning and deep learning to analyze data from various sources, such as satellites, drones, and sensors, and by using natural language generation and machine learning to generate reports and feedback for foresters and landowners. For example, Pachama is an AI company that uses machine learning and deep learning to provide forest management for foresters and landowners, by using cloud computing and analytics to collect and analyze data from various sources, such as satellite imagery, lidar, and carbon measurements, and by using natural language generation and machine learning to provide insights and recommendations for forest carbon sequestration and restoration.
AI in Water Management
AI can help manage water resources more effectively, by using machine learning and deep learning to analyze data from various sources, such as sensors, meters, and satellites, and by using natural language generation and machine learning to generate reports and feedback for water users and providers. For example, Fracta is an AI company that uses machine learning and deep learning to provide water management for water users and providers, by using cloud computing and analytics to collect and analyze data from various sources, such as water pipes, valves, and pumps, and by using natural language generation and machine learning to provide insights and recommendations for water leakage detection and prevention6.
AI in environment is a rapidly growing and evolving field, with many opportunities and challenges. AI can help protect and restore the environment, and mitigate the effects of climate change. However, AI poses ethical and social issues, such as privacy, security, accountability, bias, and trust. Therefore, it is important to develop and use AI in environment responsibly, ethically, and sustainably, and to ensure that AI respects human dignity and rights.
Thirteenth AI Applications: AI in Logistics
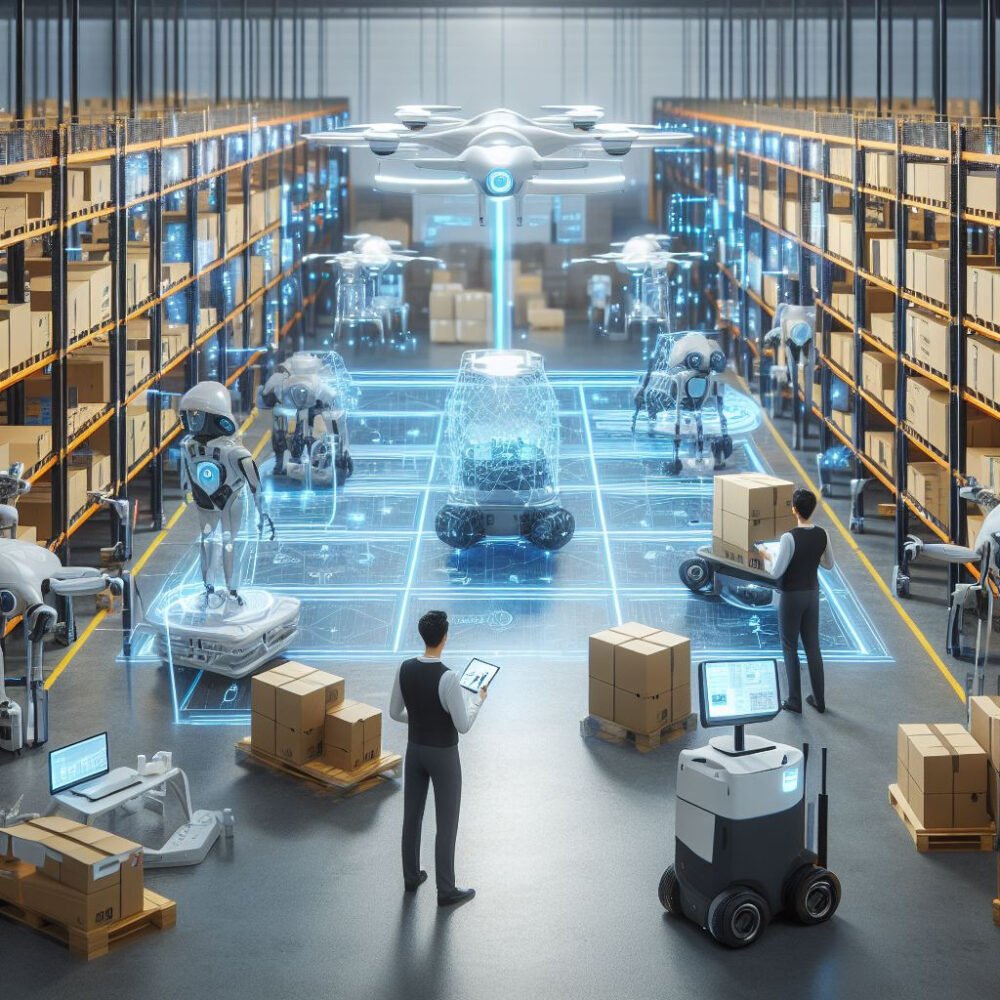
Logistics is one of the most complex and competitive domains for AI applications. AI can help improve the speed and accuracy of logistics operations, and reduce costs and errors. Some of the AI applications in logistics are:
Route Optimization
AI can help optimize the routes and schedules of vehicles and drivers, by using machine learning and deep learning to analyze data from various sources, such as traffic, weather, and customer demand, and by using natural language generation and machine learning to generate plans and actions for logistics providers and customers. For example, UPS is a logistics company that uses machine learning and deep learning to provide route optimization for its drivers and customers, by using cloud computing and analytics to collect and analyze data from various sources, such as GPS, sensors, and maps, and by using natural language generation and machine learning to provide insights and recommendations for route planning and delivery.
Demand Forecasting
AI can help predict the demand for goods and services, by using machine learning and deep learning to analyze data from various sources, such as sales, inventory, and social media, and by using natural language generation and machine learning to generate forecasts and insights for logistics providers and customers. For example, Walmart is a retail company that uses machine learning and deep learning to provide demand forecasting for its suppliers and customers, by using cloud computing and analytics to collect and analyze data from various sources, such as point-of-sale, online transactions, and customer feedback, and by using natural language generation and machine learning to provide insights and recommendations for inventory management and replenishment.
Warehouse Automation
AI can help automate and optimize warehouse operations, by using computer vision and machine learning to enable robots to perceive and manipulate goods, and by using reinforcement learning and deep learning to enable robots to learn and improve their skills. For example, Amazon is an e-commerce company that uses computer vision and machine learning to provide warehouse automation for its workers and customers, by using cloud computing and robotics to collect and analyze data from images of goods, and by using reinforcement learning and deep learning to provide features such as Kiva, which are robots that can move and sort goods in the warehouse.
Freight Transportation
AI can help improve the efficiency and safety of freight transportation, by using machine learning and deep learning to analyze data from various sources, such as sensors, cameras, and radars, and by using natural language generation and machine learning to generate reports and feedback for freight operators and customers. For example, Convoy is an AI company that uses machine learning and deep learning to provide freight transportation for freight operators and customers, by using cloud computing and IoT to collect and analyze data from various sources, such as trucks, drivers, shippers, and by using natural language generation and machine learning to provide insights and recommendations for freight matching and pricing.
AI in Customer Service
AI can help provide customer service for logistics providers and customers, by using natural language processing and speech recognition to understand and respond to customer queries, and by using natural language generation and speech synthesis to generate natural and engaging responses. For example, FedEx is a logistics company that uses natural language processing and speech recognition to provide customer service for its customers, by using chatbots and voice assistants that can converse with customers in natural language, and by using natural language generation and speech synthesis to provide feedback and guidance for various logistics issues, such as tracking, delivery, and returns.
AI in logistics is a rapidly growing and evolving field, with many opportunities and challenges. AI can help improve the speed and accuracy of logistics operations, and reduce costs and errors. However, AI poses ethical and social issues, such as privacy, security, accountability, bias, and trust. Therefore, it is important to develop and use AI in logistics responsibly, ethically, and sustainably, and to ensure that AI respects human dignity and rights.
Fourteenth AI Applications: AI in Media
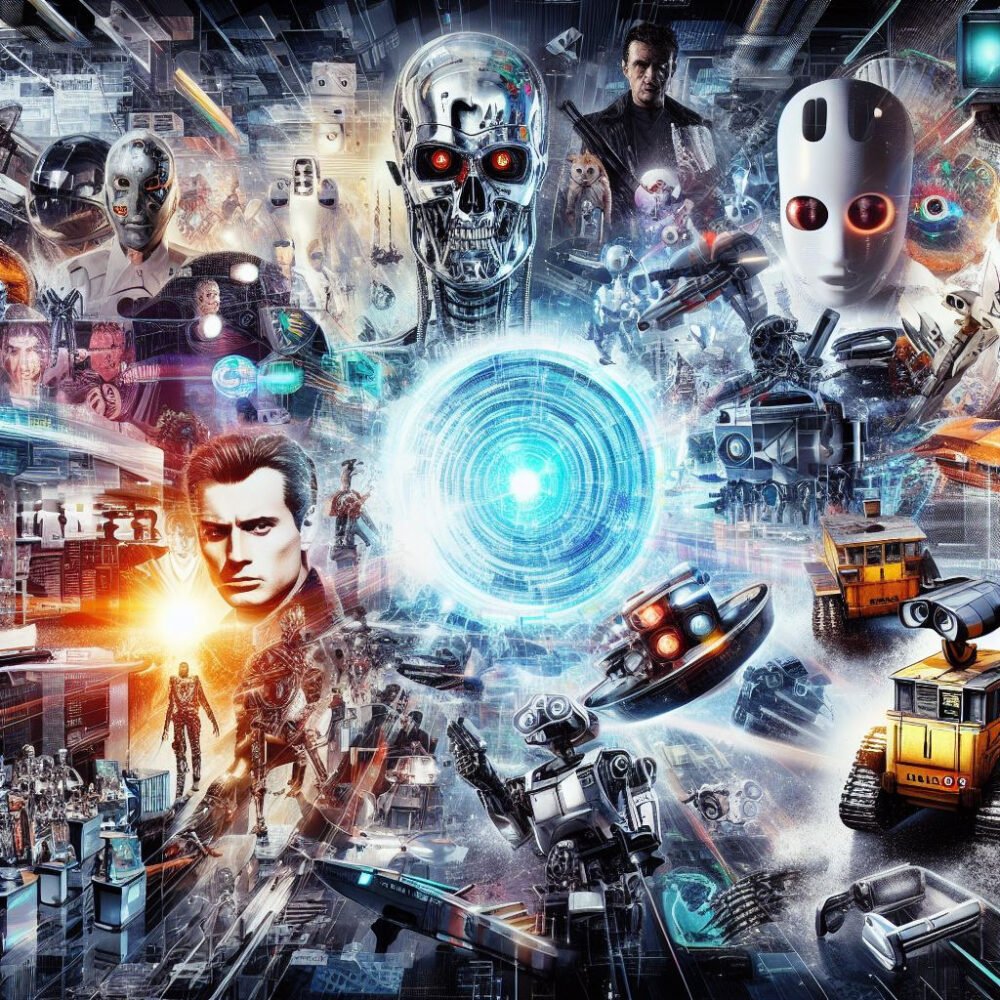
Media is one of the most creative and influential domains for AI applications. AI can help create and distribute various forms of media content, such as news, music, movies, TV, and art. Some of the AI applications in media are:
AI News Generation
AI can help generate news articles, by using natural language processing and natural language generation to analyze and synthesize data from various sources, such as facts, statistics, and quotes, and by using natural language generation and machine learning to generate natural and engaging texts for readers and viewers. For example, Associated Press is a news agency that uses natural language processing and natural language generation to provide news generation for its clients, by using cloud computing and analytics to collect and analyze data from various sources, such as sports scores, financial reports, and election results, and by using natural language generation and machine learning to provide insights and stories for news reporting.
Music Creation
AI can help create music, by using machine learning and deep learning to generate and synthesize music, such as melodies, harmonies, and lyrics, and by using natural language processing and machine learning to analyze and recommend music, such as genres, artists, and songs. For example, Amper Music is an AI company that uses machine learning and deep learning to provide music creation for its users, by using cloud computing and analytics to collect and analyze data from various sources, such as user preferences, mood, and style, and by using natural language processing and machine learning to provide features such as Amper Score, which can compose and produce original music for various purposes, such as videos, podcasts, and games.
AI in Movie Production
AI can help produce movies, by using machine learning and deep learning to generate and edit movie content, such as scripts, dialogues, and scenes, and by using natural language processing and machine learning to analyze and recommend movies, such as genres, directors, and actors. For example, ScriptBook is an AI company that uses machine learning and deep learning to provide movie production for its clients, by using cloud computing and analytics to collect and analyze data from various sources, such as movie scripts, box office data, and audience feedback, and by using natural language processing and machine learning to provide insights and predictions for movie success and quality.
AI in TV Content
AI can help create and distribute TV content, by using machine learning and deep learning to generate and optimize TV content, such as scripts, dialogues, and scenes, and by using natural language processing and machine learning to analyze and recommend TV content, such as genres, producers, and actors. For example, ZoneTV is an AI company that uses machine learning and deep learning to provide TV content for its users, by using cloud computing and analytics to collect and analyze data from various sources, such as video libraries, user behavior, and social media, and by using natural language processing and machine learning to provide features such as ZoneTV Studio, which can create and deliver personalized and dynamic TV channels for various interests, such as sports, comedy, and lifestyle.
AI in Streaming Services
AI can help provide streaming services for movies, TV shows, music, and other content, by using machine learning and deep learning to optimize and personalize the streaming experience, such as quality, speed, and recommendations, and by using natural language processing and machine learning to provide interactive and engaging features, such as voice control, chat, and social media. For example, Netflix is an online streaming service that uses machine learning and deep learning to provide streaming for its users, by using cloud computing and edge computing to collect and analyze data from its videos and users, and by using natural language processing and machine learning to provide features such as personalized recommendations, ratings, and summaries for each video.
AI in Social Media
AI can help develop and operate social media platforms, by using machine learning and deep learning to generate and optimize social media content, such as posts, stories, and filters, and by using natural language processing and machine learning to analyze and recommend social media content, such as friends, groups, and trends. For example, Facebook is a social media platform that uses machine learning and deep learning to provide social media for its users, by using cloud computing and analytics to collect and analyze data from its users and content and using natural language processing and machine learning to provide features such as news feed, messenger, marketplace, and groups.
Creative Writing
AI can help generate creative text formats, such as poems, stories, code, essays, songs, and more, by using machine learning and deep learning to generate and synthesize natural language text, based on the user’s input and preferences. For example, OpenAI is an AI research organization that develops and operates AI-powered tools for creative writing, such as GPT-3, which can generate natural language text for various purposes, such as writing, summarizing, and answering, and DALL-E, which can generate art images for various prompts, such as drawing a cat wearing a hat.
Art Generation
AI can help generate art images, such as paintings, drawings, and cartoons, by using machine learning and deep learning to generate and synthesize visual content, based on the user’s input and preferences. For example, Artbreeder is an online service that uses machine learning and deep learning to provide art generation for its users, by using generative adversarial networks (GANs) that can create and modify images, such as portraits, landscapes, and animals, and by using natural language processing and machine learning to provide features such as tags, ratings, and comments.
AI in media is a rapidly growing and evolving field, with many opportunities and challenges. AI can help create and distribute various forms of media content, such as news, music, movies, TV, and art. However, AI poses ethical and social issues, such as privacy, security, accountability, bias, and trust. Therefore, it is important to develop and use AI in media responsibly, ethically, and sustainably, and to ensure that AI respects human dignity and rights.
Fifteenth AI Applications: AI in Military
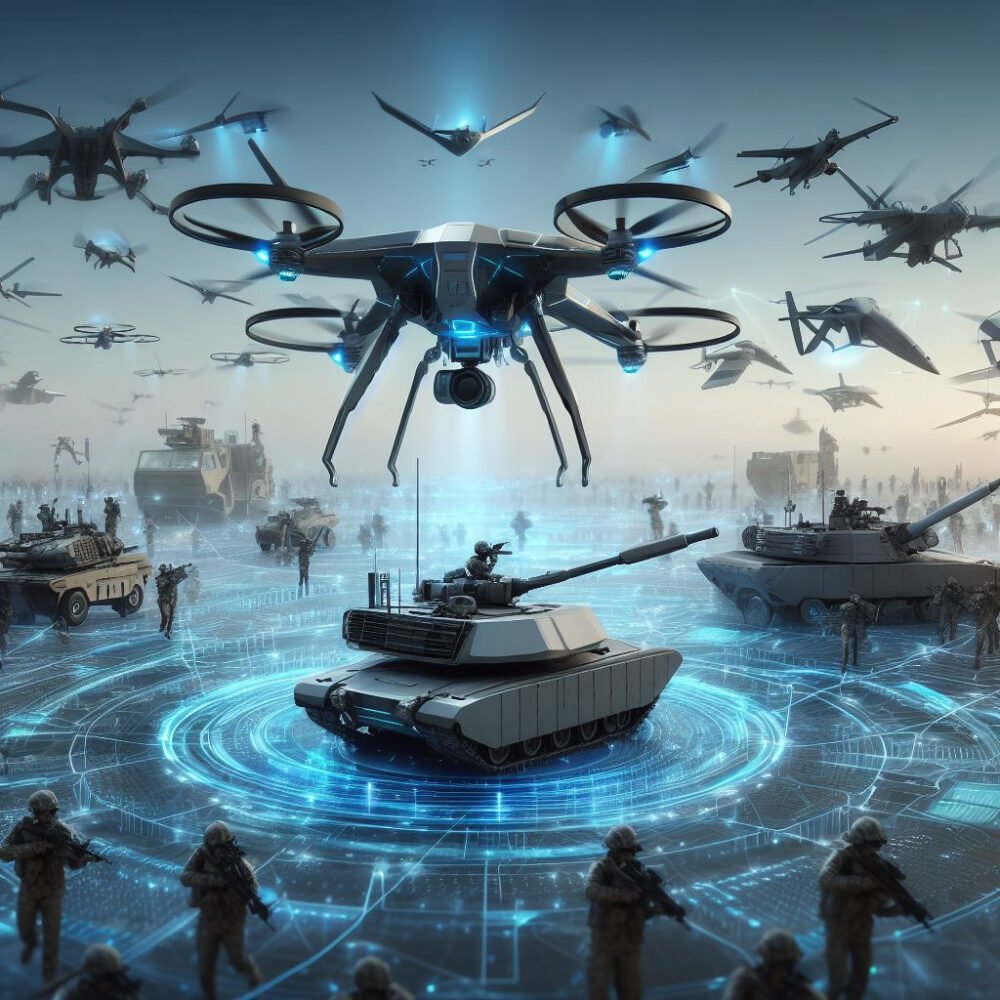
Military is one of the most strategic and controversial domains for AI applications. AI can help improve the security and effectiveness of military operations, and reduce casualties and costs. Some of the AI applications in the military are:
AI in Intelligence Gathering
AI can help gather intelligence about adversaries and threats, by using machine learning and deep learning to analyze data from various sources, such as satellites, drones, sensors, and social media, and by using natural language generation and machine learning to generate reports and insights for military commanders and analysts. For example, Palantir is an AI company that uses machine learning and deep learning to provide intelligence gathering for military and intelligence agencies, by using cloud computing and analytics to collect and analyze data from various sources, such as battlefield data, enemy movements, and terrorist activities, and by using natural language generation and machine learning to provide insights and predictions for intelligence analysis and decision making.
AI in Target Recognition
AI can help identify targets for military attacks, by using computer vision and machine learning to analyze images and videos of potential targets, such as buildings, vehicles, and weapons, and by using natural language generation and machine learning to generate reports and feedback for military commanders and operators. For example, Project Maven is a military project that uses computer vision and machine learning to provide target recognition for military and intelligence agencies, by using cloud computing and edge computing to collect and analyze data from various sources, such as drones, cameras, and radars, and by using natural language generation and machine learning to provide insights and recommendations for target identification and classification.
Autonomous Weapons
AI can help develop and operate autonomous weapons systems, such as missiles, drones, and robots, that can perform military tasks without human intervention, by using computer vision and machine learning to enable weapons systems to perceive and navigate their environment, and by using reinforcement learning and deep learning to enable weapons systems to learn and improve their skills. For example, DARPA is a military research agency that develops and operates autonomous weapons systems, such as the X-47B, which is an unmanned combat air vehicle that can take off and land on aircraft carriers, and the Sea Hunter, which is an unmanned surface vessel that can track and engage enemy submarines.
Cyber Warfare
AI can help engage in cyber warfare against adversaries, by using machine learning and deep learning to analyze and manipulate data from various sources, such as networks, systems, and devices, and by using natural language generation and machine learning to generate attacks and defenses for cyber operations. For example, Cyber Grand Challenge is a military competition that uses machine learning and deep learning to provide cyber warfare for military and intelligence agencies, by using cloud computing and analytics to collect and analyze data from various sources, such as software, hardware, and malware, and by using natural language generation and machine learning to provide autonomous cyber systems that can attack and defend against cyber threats.
AI in Logistics
AI can help manage military logistics more efficiently, by using machine learning and deep learning to analyze data from various sources, such as supply, demand, delivery, and maintenance, and by using natural language generation and machine learning to generate plans and actions for military logistics. For example, IBM Watson is an AI platform that uses machine learning and deep learning to provide logistics for military and intelligence agencies, by using cloud computing and analytics to collect and analyze data from various sources, such as inventory, orders, vehicles, and weather, and by using natural language generation and machine learning to provide insights and recommendations for logistics optimization and management. You can read more about AI in Logistics.
AI-Powered Training
AI can help train soldiers more effectively, by using machine learning and deep learning to create and optimize training content, such as simulations, scenarios, and feedback, and by using natural language processing and speech recognition to understand and respond to soldier queries, and by using natural language generation and speech synthesis to generate natural and engaging responses. For example, Bohemia Interactive Simulations is an AI company that uses machine learning and deep learning to provide training for military and defense agencies, by using cloud computing and VR to create and deliver realistic and immersive training simulations, such as virtual battlespaces, and by using natural language processing and speech recognition to provide voice-based interaction and communication for trainees and instructors.
AI-Powered Simulation
AI can help simulate military situations and outcomes, by using machine learning and deep learning to model and predict various factors, such as enemy behavior, terrain, and weather, and by using natural language generation and machine learning to generate reports and insights for military commanders and analysts. For example, One World Terrain is a military project that uses machine learning and deep learning to provide simulation for military and intelligence agencies, by using cloud computing and IoT to collect and analyze data from various sources, such as satellites, drones, and sensors, and by using natural language generation and machine learning to provide realistic and accurate 3D models of the terrain for simulation and analysis.
AI in military is a rapidly growing and evolving field, with many opportunities and challenges. AI can help improve the security and effectiveness of military operations, and reduce casualties and costs. However, AI poses ethical and social issues, such as privacy, security, accountability, bias, and trust. Therefore, it is important to develop and use AI in military responsibly, ethically, and sustainably, and to ensure that AI respects human dignity and rights.
Sixteenth AI Applications: AI in Public Safety
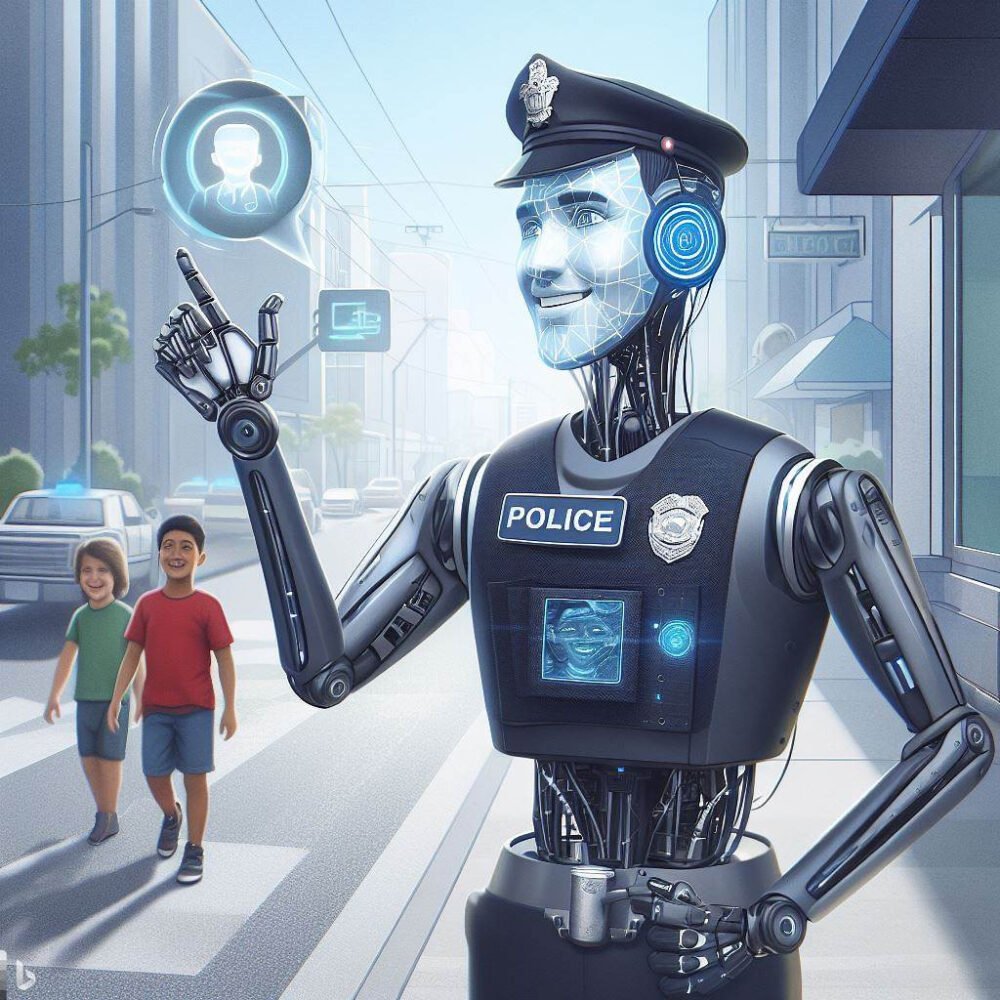
Public safety is one of AI applications’ most important and challenging domains. AI can help prevent and respond to various threats and emergencies, such as crime, terrorism, natural disasters, and accidents. Some of the AI applications in public safety are:
AI-Powered Crime Prevention
AI can help prevent crime, by using machine learning and deep learning to analyze data from various sources, such as cameras, sensors, and social media, and by using natural language generation and machine learning to generate alerts and actions for public safety officials and citizens. For example, SoundThinking is an AI company that uses machine learning and deep learning to provide gunshot detection and location for public safety officials and citizens, by using cloud computing and IoT to collect and analyze data from acoustic sensors, and by using natural language generation and machine learning to provide insights and notifications for public safety response.
AI-Powered Crime Investigation
AI can help investigate crime, by using computer vision and machine learning to analyze images and videos of crime scenes and suspects, and by using natural language processing and machine learning to analyze texts and speech of witnesses and victims. For example, Veritone is an AI company that uses computer vision and machine learning to provide facial recognition and object detection for crime investigation, by using cloud computing and edge computing to collect and analyze data from images and videos of crime scenes and suspects, and by using natural language processing and machine learning to provide insights and recommendations for crime identification and solving.
AI-Powered Terrorism Detection
AI can help detect terrorism, by using machine learning and deep learning to analyze data from various sources, such as networks, systems, and devices, and by using natural language generation and machine learning to generate alerts and actions for public safety officials and citizens. For example, Darktrace is an AI company that uses machine learning and deep learning to provide cyber defense and threat detection for public safety officials and citizens, by using cloud computing and analytics to collect and analyze data from various sources, such as networks, systems, and devices, and by using natural language generation and machine learning to provide insights and recommendations for cyber security and resilience.
AI-Powered Disaster Management
AI can help manage disasters, by using machine learning and deep learning to analyze data from various sources, such as satellites, sensors, and social media, and by using natural language generation and machine learning to generate reports and feedback for public safety officials and citizens. For example, One Concern is an AI company that uses machine learning and deep learning to provide disaster management for public safety officials and citizens, by using cloud computing and analytics to collect and analyze data from various sources, such as weather, terrain, and population, and by using natural language generation and machine learning to provide insights and predictions for disaster impacts and actions4.
AI-Powered Accident Prevention
AI can help prevent accidents, by using machine learning and deep learning to analyze data from various sources, such as sensors, cameras, and radars, and by using natural language generation and machine learning to generate alerts and actions for public safety officials and citizens. For example, Mobileye is an AI company that uses machine learning and deep learning to provide driver assistance and collision avoidance for public safety officials and citizens, by using cloud computing and edge computing to collect and analyze data from various sources, such as cameras, radars, and ultrasonic sensors, and by using natural language generation and machine learning to provide insights and recommendations for driving safety and efficiency5.
AI-Powered Emergency Response
AI can help respond to emergencies, by using natural language processing and speech recognition to understand and respond to emergency calls, and by using natural language generation and speech synthesis to generate natural and engaging responses. For example, RapidSOS is an online emergency service that uses natural language processing and speech recognition to provide emergency response for public safety officials and citizens, by using cloud computing and IoT to collect and analyze data from various sources, such as phones, wearables, and vehicles, and by using natural language generation and speech synthesis to provide features such as RapidSOS Haven, which can connect users with emergency services in natural language, and RapidSOS Clearinghouse, which can share users’ location and health data with emergency services.
AI in public safety is a rapidly growing and evolving field, with many opportunities and challenges. AI can help prevent and respond to various threats and emergencies, such as crime, terrorism, natural disasters, and accidents. However, AI poses some ethical and social issues, such as privacy, security, accountability, bias, and trust. Therefore, it is important to develop and use AI in public safety responsibly, ethically, and sustainably, and to ensure that AI respects human dignity and rights.
Seventeenth AI Applications: AI in Scientific Research
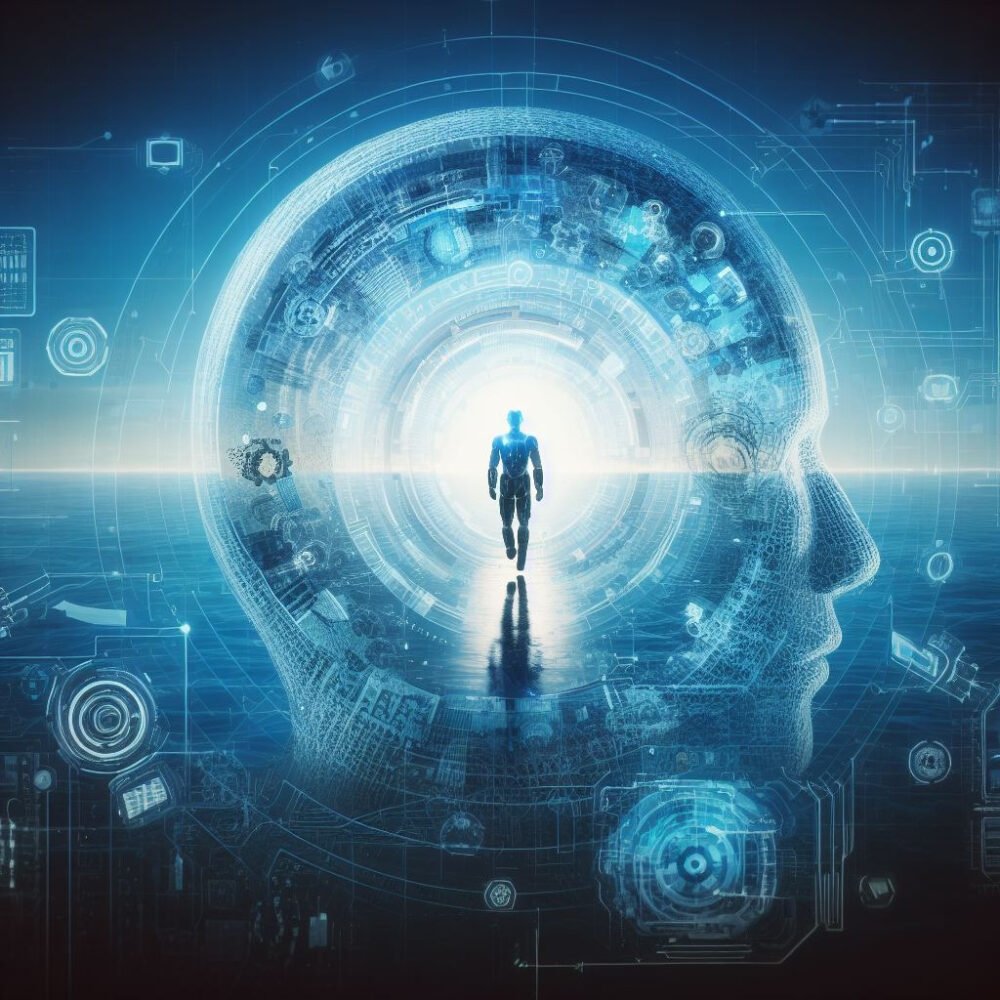
Research is one of the most fundamental and innovative domains for AI applications. AI can help enhance the quality and efficiency of research processes, and discover new knowledge and insights. Some of the AI applications in research are:
AI-Powered Literature Review
AI can help review the existing literature on a given topic, by using natural language processing and natural language generation to analyze and synthesize data from various sources, such as academic papers, books, and reports, and by using natural language generation and machine learning to generate summaries and insights for researchers and readers. For example, Semantic Scholar is an AI platform that uses natural language processing and natural language generation to provide literature review for researchers and readers, by using cloud computing and analytics to collect and analyze data from various sources, such as scientific literature, citations, and figures, and by using natural language generation and machine learning to provide features such as paper summaries, key citations, and related papers.
AI-Powered Data Analysis
AI can help analyze data from various sources, such as experiments, surveys, and simulations, by using machine learning and deep learning to identify and extract relevant information, such as patterns, trends, and outliers, and by using natural language generation and machine learning to generate reports and feedback for researchers and readers. For example, DataRobot is an AI company that uses machine learning and deep learning to provide data analysis for researchers and readers, by using cloud computing and analytics to collect and analyze data from various sources, such as spreadsheets, databases, and APIs, and by using natural language generation and machine learning to provide features such as automated machine learning, model deployment, and data visualization.
AI-Powered Hypothesis Generation
AI can help generate hypotheses for research questions, by using machine learning and deep learning to analyze data from various sources, such as literature, data, and models, and by using natural language generation and machine learning to generate hypotheses and insights for researchers and readers. For example, IBM Watson Discovery is an AI platform that uses machine learning and deep learning to provide hypothesis generation for researchers and readers, by using cloud computing and analytics to collect and analyze data from various sources, such as scientific literature, patents, and news, and by using natural language generation and machine learning to provide features such as natural language queries, semantic search, and knowledge graphs.
AI-Powered Experiment Design
AI can help design experiments for testing hypotheses, by using machine learning and deep learning to analyze data from various sources, such as literature, data, and models, and by using natural language generation and machine learning to generate plans and actions for researchers and readers. For example, LabTwin is an AI company that uses machine learning and deep learning to provide experiment design for researchers and readers, by using cloud computing and IoT to collect and analyze data from various sources, such as lab equipment, protocols, and notes, and by using natural language generation and machine learning to provide features such as voice assistants, smart timers, and protocol reminders.
AI-Powered Research Collaboration
AI can help facilitate research collaboration among researchers, by using natural language processing and speech recognition to understand and respond to researcher queries, and by using natural language generation and speech synthesis to generate natural and engaging responses. For example, Slack is an online communication platform that uses natural language processing and speech recognition to provide research collaboration for researchers, by using cloud computing and chatbots to collect and analyze data from various sources, such as messages, files, and apps, and by using natural language generation and speech synthesis to provide features such as channels, threads, and calls.
AI in research is a rapidly growing and evolving field, with many opportunities and challenges. AI can help enhance the quality and efficiency of research processes, and discover new knowledge and insights. However, AI poses some ethical and social issues, such as privacy, security, accountability, bias, and trust. Therefore, it is important to develop and use AI in research responsibly, ethically, and sustainably, and to ensure that AI respects human dignity and rights.
Eighteenth AI Applications: AI in Space
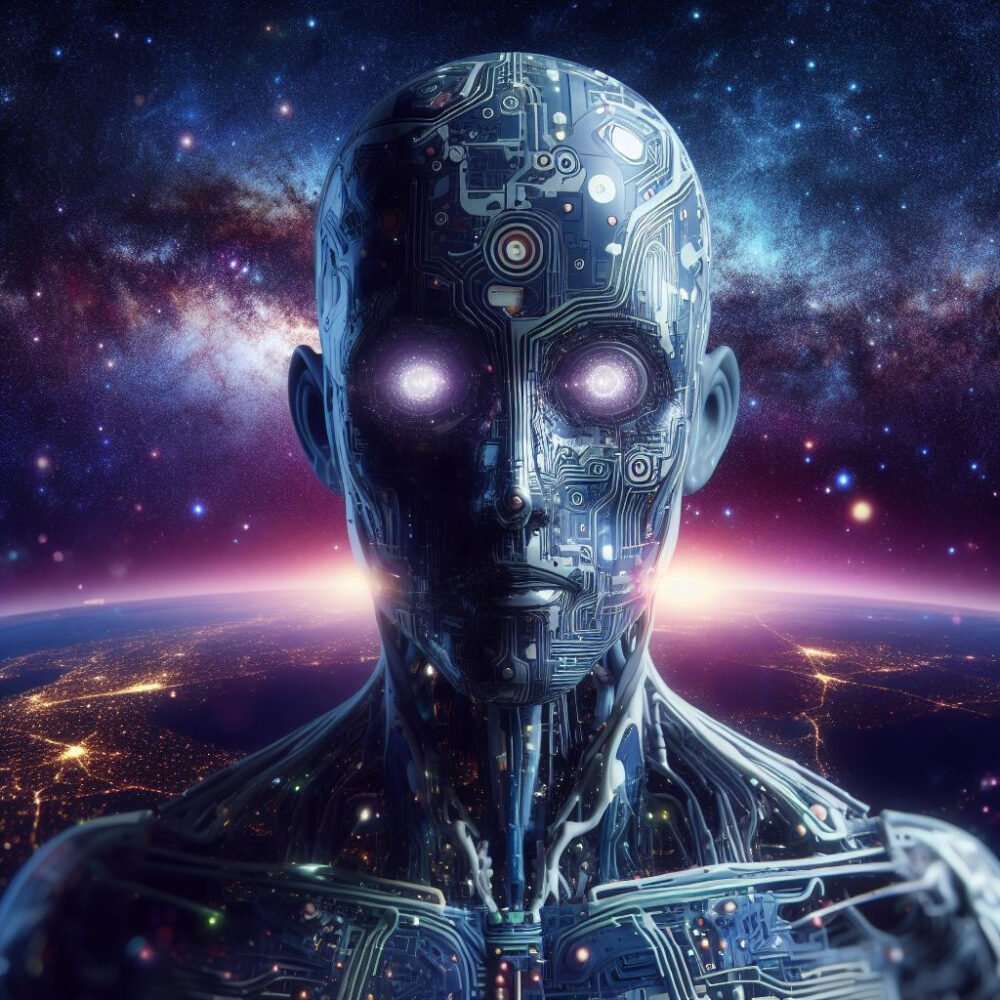
Space is one of the most fascinating and challenging domains for AI applications. AI can help explore and understand the vast and mysterious universe and support human and robotic missions in space. Some of the AI applications in space are:
AI-Powered Space Exploration
AI can help explore the solar system and beyond, by using machine learning and deep learning to analyze data from various sources, such as satellites, rovers, and telescopes, and by using natural language generation and machine learning to generate reports and insights for space scientists and enthusiasts. For example, NASA is a space agency that uses machine learning and deep learning to provide space exploration for space scientists and enthusiasts, by using cloud computing and analytics to collect and analyze data from various sources, such as the Mars rovers, the Juno spacecraft, and the Hubble Space Telescope, and by using natural language generation and machine learning to provide insights and discoveries for planetary science, astrophysics, and heliophysics.
AI-Powered Space Navigation
AI can help navigate and maneuver in space, by using computer vision and machine learning to enable spacecraft and satellites to perceive and avoid obstacles, such as asteroids, debris, and other spacecraft, and by using reinforcement learning and deep learning to enable spacecraft and satellites to learn and improve their skills. For example, SpaceX is a space company that uses computer vision and machine learning to provide space navigation for its spacecraft and satellites, by using cloud computing and edge computing to collect and analyze data from various sources, such as cameras, radars, and ultrasonic sensors, and by using reinforcement learning and deep learning to provide features such as Starlink, which is a constellation of satellites that can autonomously adjust their orbits, and Starship, which is a reusable launch vehicle that can land and take off vertically.
AI-Powered Space Communication
AI can help communicate in space, by using natural language processing and speech recognition to understand and respond to astronaut queries, and by using natural language generation and speech synthesis to generate natural and engaging responses. For example, CIMON is an AI assistant that uses natural language processing and speech recognition to provide space communication for astronauts, by using cloud computing and IoT to collect and analyze data from various sources, such as voice, gesture, and facial expression, and by using natural language generation and speech synthesis to provide features such as voice-based interaction, emotion recognition, and task support.
AI-Powered Space Data
AI can help process and utilize the massive amounts of data collected from space, by using machine learning and deep learning to identify and extract relevant information, such as images, signals, and patterns, and by using natural language generation and machine learning to generate reports and feedback for space scientists and enthusiasts. For example, ESA is a space agency that uses machine learning and deep learning to provide space data for space scientists and enthusiasts, by using cloud computing and analytics to collect and analyze data from various sources, such as the Copernicus satellites, the Gaia spacecraft, and the Herschel Space Observatory, and by using natural language generation and machine learning to provide insights and solutions for Earth observation, galaxy mapping, and cosmic phenomena.
AI-Powered Space Education
AI can help educate and inspire the public about space, by using machine learning and deep learning to create and optimize educational content, such as courses, games, and quizzes, and by using natural language processing and speech recognition to understand and respond to user queries, and by using natural language generation and speech synthesis to generate natural and engaging responses. For example, NASA Jet Propulsion Laboratory is a space research center that uses machine learning and deep learning to provide space education for the public, by using cloud computing and VR to create and deliver interactive and immersive educational content, such as Eyes on the Solar System, which is a 3D simulation of the solar system, and by using natural language processing and speech recognition to provide features such as NASA Selfies, which is an app that lets users take selfies in space.
AI in space is a rapidly growing and evolving field, with many opportunities and challenges. AI can help explore and understand the vast and mysterious universe and support human and robotic missions in space. However, AI also poses some ethical and social issues, such as privacy, security, accountability, bias, and trust. Therefore, it is important to develop and use AI in space responsibly, ethically, and sustainably, and to ensure that AI respects human dignity and rights.
Nineteenth AI Applications: AI in Sports
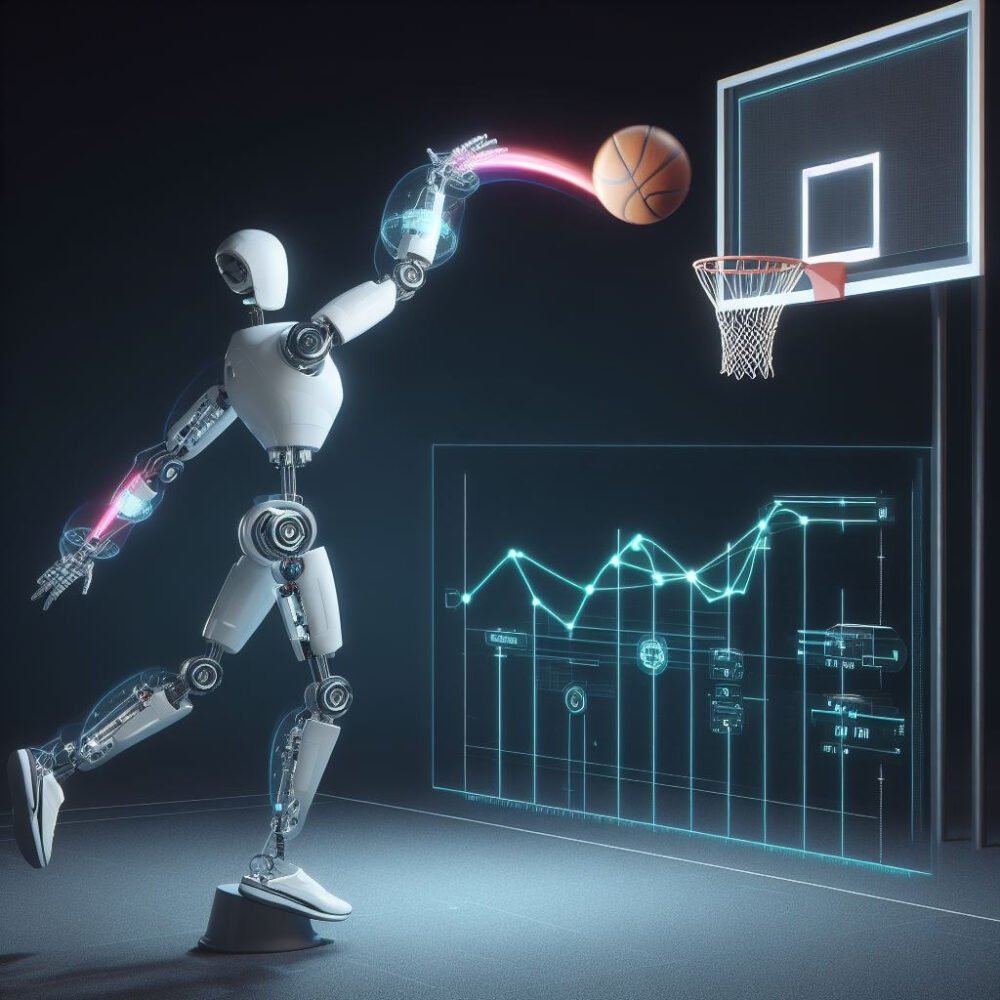
Sports is one of the most exciting and competitive domains for AI applications. AI can help enhance the performance and experience of athletes, coaches, fans, and media. Some of the AI applications in sports are:
Performance Analysis
AI can help analyze the performance of athletes and teams, by using machine learning and deep learning to analyze data from various sources, such as video feeds, wearables, and biometrics, and by using natural language generation and machine learning to generate reports and feedback for athletes and coaches. For example, STATS is an AI company that uses machine learning and deep learning to provide performance analysis for athletes and coaches, by using cloud computing and analytics to collect and analyze data from various sources, such as game footage, player tracking, and event data, and by using natural language generation and machine learning to provide insights and recommendations for player evaluation, game strategy, and injury prevention1.
AI-Powered Fan Engagement
AI can help engage and entertain fans, by using machine learning and deep learning to create and optimize fan content, such as highlights, stories, and games, and by using natural language processing and machine learning to analyze and recommend fan content, such as social media, news, and podcasts. For example, WSC Sports is an AI company that uses machine learning and deep learning to provide fan engagement for sports leagues and broadcasters, by using cloud computing and edge computing to collect and analyze data from various sources, such as live video streams, scoreboards, and commentary, and by using natural language processing and machine learning to provide features such as automated highlights, personalized stories, and interactive games.
AI-Powered Media production
AI can help produce and distribute media content, by using machine learning and deep learning to generate and optimize media content, such as graphics, captions, and summaries, and by using natural language processing and machine learning to analyze and recommend media content, such as genres, channels, and ads. For example, IBM Watson Media is an AI platform that uses machine learning and deep learning to provide media production for sports leagues and broadcasters, by using cloud computing and analytics to collect and analyze data from various sources, such as video feeds, audio feeds, and metadata, and by using natural language processing and machine learning to provide features such as automated graphics, live captions, and video summaries.
AI-Powered Sports Betting
AI can help facilitate and improve sports betting, by using machine learning and deep learning to analyze data from various sources, such as historical data, current data, and social data, and by using natural language generation and machine learning to generate predictions and insights for bettors and bookmakers. For example, Betegy is an AI company that uses machine learning and deep learning to provide sports betting for bettors and bookmakers, by using cloud computing and analytics to collect and analyze data from various sources, such as game results, team statistics, and player ratings, and by using natural language generation and machine learning to provide insights and predictions for betting outcomes and odds.
AI-Powered Sports Journalism
AI can help create and deliver sports journalism, by using natural language processing and natural language generation to analyze and synthesize data from various sources, such as game data, player data, and fan data, and by using natural language generation and machine learning to generate natural and engaging texts for readers and viewers. For example, The Washington Post is a news outlet that uses natural language processing and natural language generation to provide sports journalism for its readers and viewers, by using cloud computing and analytics to collect and analyze data from various sources, such as game scores, player statistics, and fan reactions, and by using natural language generation and machine learning to provide features such as Heliograf, which can write automated stories and updates for sports events5.
AI in sports is a rapidly growing and evolving field, with many opportunities and challenges. AI can help enhance the performance and experience of athletes, coaches, fans, and media. However, AI also poses some ethical and social issues, such as privacy, security, accountability, bias, and trust. Therefore, it is important to develop and use AI in sports responsibly, ethically, and sustainably, and to ensure that AI respects human dignity and rights.
Conclusion
- AI is transforming the retail industry in many ways, from demand forecasting to personalized recommendations, cashierless technology, automated inventory management, customer sentiment analysis, visual search, voice assistants, fraud detection, and product development.
- AI can help retailers improve their efficiency, accuracy, speed, and quality of service, as well as enhance their customer experience, loyalty, and satisfaction.
- However, AI also poses some challenges and risks for retailers, such as privacy violations, bias and discrimination, job displacement, and ethical dilemmas.
- Free AI tools can be a great way for retailers to experiment with AI without investing a lot of money or time, and to solve specific problems or tasks more efficiently and accurately than manual or traditional methods.
- Free AI tools can also help retailers improve their skills and knowledge with AI, and to have fun and explore new possibilities with AI.
- However, free AI tools also have some limitations and risks that retailers should be aware of, such as reliability, security, user-friendliness, and responsibility.
- Therefore, retailers should be careful and smart when choosing, comparing, evaluating, improving, and having fun with free AI tools, and to use them responsibly, ethically, and sustainably, and to ensure that they respect human dignity and rights.
This is the end of the article. I hope you enjoyed reading it and learned something new about AI applications in retail and how to use free AI tools. If you have any questions or feedback, please let me know. Thank you for your attention. 😊