Artificial intelligence, commonly known as AI, refers to the ability of machines to perform tasks that typically require human intelligence, such as learning, reasoning, and decision-making. Although not a new concept, AI has gained a lot of attention and momentum in recent years, primarily due to advances in computing power, data availability, and algorithm development. AI is now being utilized across various domains and industries, including healthcare, education, finance, and manufacturing.
Manufacturing is one of the oldest and most important industries in the world, as it produces the goods and services that we use every day. Manufacturing is also one of the most competitive and dynamic industries, as it constantly strives to improve efficiency, quality, innovation, and customer satisfaction. However, manufacturing also faces many challenges, such as rising costs, environmental regulations, labor shortages, global competition, and changing customer demands.
This is where AI comes in. AI boosts manufacturing performance and productivity. It also enables new products, services, and markets. This article covers AI in manufacturing. You will learn about AI types, benefits, challenges, and cases. You will also discover the future of AI in manufacturing and how to get started today.
Key Takeaways
Section | Key Takeaways |
---|---|
Introduction | AI in manufacturing is the use of AI techniques and technologies to enhance and automate various aspects of the manufacturing process, such as design, production, quality control, and maintenance. |
Benefits of AI in Manufacturing | AI in manufacturing can provide many benefits and advantages, such as improved efficiency, quality, innovation, and customer satisfaction, and can create many opportunities and possibilities, such as new products and services, and new markets and segments. |
Challenges of implementing AI in Manufacturing | AI in manufacturing also comes with some challenges and risks, that need to be addressed and managed, such as the lack of skilled workers, data security and privacy concerns, cost of implementation, ethical implications, and social impact. |
Case studies of successful AI implementations in Manufacturing | AI in manufacturing is not a hypothetical or futuristic scenario, but a reality and a necessity for many manufacturers around the world, who have successfully implemented and leveraged AI solutions and systems to achieve their goals and objectives, and to gain a competitive edge in the market. |
The future of AI in Manufacturing | AI in manufacturing is not a static or stagnant phenomenon, but a dynamic and evolving one, that will continue to transform and revolutionize the manufacturing industry, and that will have a significant impact on the society and the environment. |
How to get started with AI in Manufacturing | AI in manufacturing is not a distant or unreachable goal, but a present and attainable one, that can be achieved and leveraged by any manufacturer, regardless of their size, sector, or region, as long as they have the vision, the strategy, the commitment, and the action to do so. |
What is AI in Manufacturing?
AI in manufacturing is the use of artificial intelligence techniques and technologies to enhance and automate various aspects of the manufacturing process, such as design, production, quality control, maintenance, logistics, and customer service. Artificial intelligence in manufacturing can also refer to creating intelligent products and systems that can interact with humans and the environment, such as smart robots, sensors, and devices.
AI in manufacturing is not a single technology, but a combination of different methods and tools that can work together to achieve specific goals and tasks. Some of the most common types of AI used in manufacturing are:
Machine Learning
Machine learning is machines learning from data and experience, without explicit programming. It analyzes large and complex data sets. Thus, it discovers patterns, trends, and insights that can help improve decision-making and optimization. Machine learning helps in creating predictive models. Those models can forecast future outcomes and events, such as demand, inventory, quality, and failures.
Deep Learning
Deep learning is a subset of machine learning that uses artificial neural networks to learn from data and perform complex tasks, such as image recognition, natural language processing, and speech recognition. It processes and interprets unstructured and multimodal data, such as images, videos, text, and audio. Deep learning extracts useful information and features that can help improve quality, safety, and efficiency.
Natural Language Processing
Natural language processing, or NLP, is the ability of machines to understand and generate natural language, such as speech and text. NLP enables communication and interaction between humans and machines. Machines like voice assistants, chatbots, and translators. NLP analyzes and extracts information from textual data, such as documents, reports, reviews, and feedback. NLP provides insights and recommendations that can help improve customer service, marketing, and innovation.
Robotic Process Automation
Robotic process automation, or RPA, is the use of software robots to automate repetitive and rule-based tasks, such as data entry, invoicing, and order processing. RPA can help reduce human errors, save time and costs, and increase accuracy and compliance. RPA combines with other AI techniques, such as machine learning and natural language processing. It creates intelligent automation that can handle more complex and dynamic tasks, such as data analysis, decision-making, and problem-solving.
Computer Vision
Computer vision is the ability of machines to see and understand visual data, such as images and videos. It performs tasks such as object detection, face recognition, scene understanding, and gesture recognition. Computer vision can help improve quality, safety, and efficiency, by enabling tasks such as defect detection, product inspection, inventory management, and worker monitoring.
Generative adversarial networks
Generative adversarial networks, or GANs, are a type of deep learning that uses two competing neural networks to generate new and realistic data, such as images, videos, and text. GANs create synthetic data that can augment and enhance existing data. They help generate new and novel designs and products that can inspire innovation and creativity.
Reinforcement Learning
Reinforcement learning is a type of machine learning that uses trial and error to learn from its own actions and feedback and to optimize its behavior and performance. It creates autonomous and adaptive systems that can learn from their own experience and environment. They achieve complex and dynamic goals, such as navigation, control, and coordination.
What are AI Solutions for Manufacturing?
AI applications span various stages and aspects of the manufacturing process, from design to delivery, and from production to customer service. Some of the most common and impactful applications of AI in manufacturing are:
Predictive Maintenance
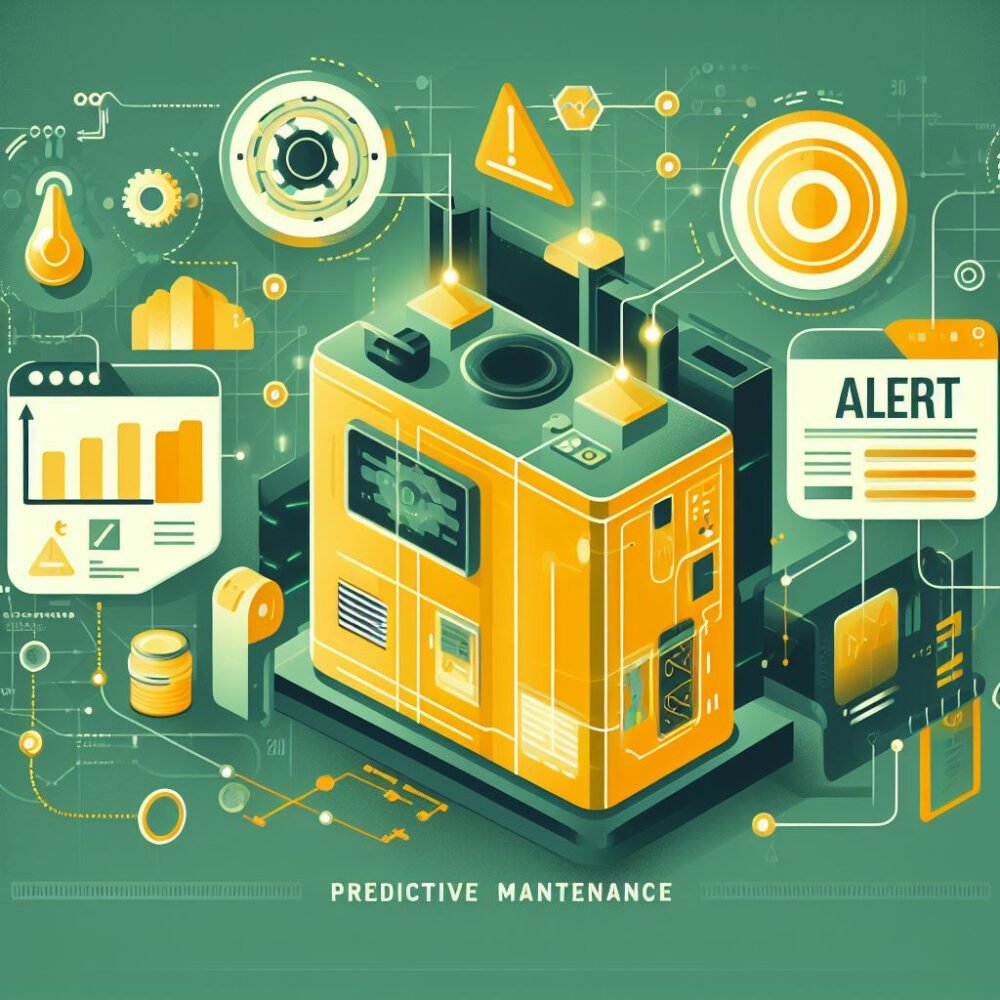
What is Predictive Maintenance?
Predictive maintenance is the use of AI to monitor and analyze the condition and performance of machines and equipment and to predict and prevent failures and breakdowns before they occur. It can help reduce downtime, save costs, extend the lifespan of assets, and improve reliability and safety. Predictive maintenance leverages sensors, IoT devices, and machine learning models. They collect and process data from machines and equipment, that can detect anomalies, faults, and degradation patterns, and that can provide alerts, recommendations, and actions to optimize maintenance schedules and procedures.
Benefits of Predictive Maintenance in Manufacturing
- Reduced downtime and increased production: By catching potential issues early, PdM allows for scheduled maintenance, minimizing unplanned downtime and disruptions to production schedules.
- Lower maintenance costs: PdM eliminates unnecessary preventive maintenance and focuses repairs on when they’re truly needed, saving resources and avoiding unnecessary expenditures.
- Extended equipment lifespan: By identifying and addressing early wear and tear, PdM helps optimize equipment performance and prolong its lifespan.
- Improved safety: Early detection of potential safety hazards like overheating or vibration anomalies helps prevent accidents and ensure worker safety.
- Data-driven decision making: PdM provides valuable insights into the health and performance of manufacturing assets, enabling data-driven decisions to optimize production processes and resource allocation.
Challenges of implementing Predictive Maintenance
- Data quality and infrastructure: PdM relies on accurate and consistent data, which requires investment in sensor technology, data storage, and analytics infrastructure.
- Skill gap: Implementing and maintaining a PdM system requires personnel with expertise in data analytics, AI, and machine learning, which can be a challenge for some companies.
- Integration with existing systems: Integrating PdM with existing IT infrastructure and maintenance systems can be complex and require careful planning.
AI-Powered Quality Control
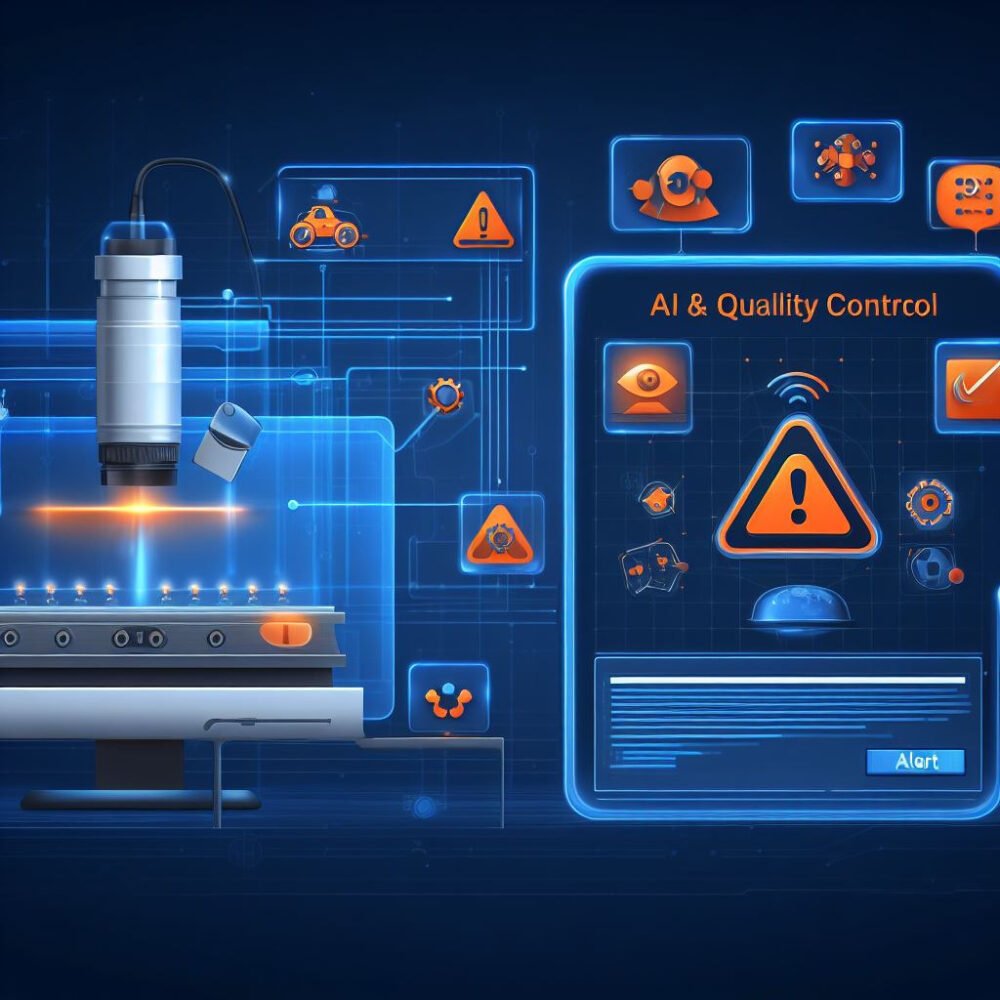
What is AI-Powered Quality Control?
Quality control is the use of AI to ensure and improve the quality and consistency of products and processes and to detect and eliminate defects and errors. It uses computer vision, deep learning, and machine learning models. Those models inspect and verify products and processes, and identify and classify defects and errors. Additionally, they provide feedback, corrections, and improvements to enhance quality standards and customer satisfaction. Moreover, quality control can use natural language processing and machine learning models. Those models analyze and extract information from textual data, such as documents, reports, reviews, and feedback. Then, they provide insights and recommendations to improve quality and compliance.
Benefits of AI-powered Quality Control:
- Improved product quality: AI systems can detect defects with greater accuracy and consistency, leading to fewer defective products and higher customer satisfaction.
- Increased production efficiency: Faster inspections and proactive maintenance reduce downtime and bottlenecks, boosting overall production throughput.
- Reduced costs: Fewer defects and less waste translate to lower production costs and improved profitability.
- Enhanced data-driven decision-making: AI-generated insights provide valuable data for optimizing production processes, improving quality control strategies, and making informed business decisions.
AI in Production Planning and Scheduling
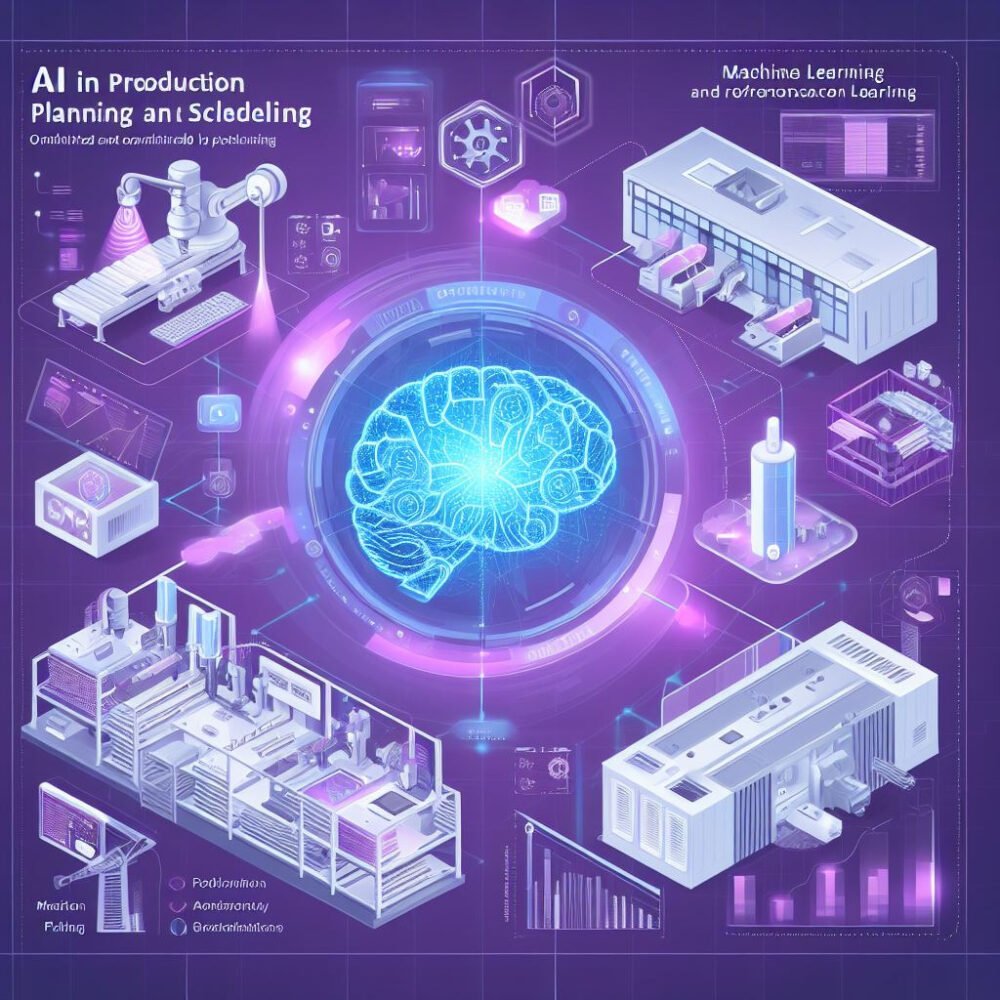
How Artificial Intelligence is Used in Production Planning and Scheduling?
Production planning and scheduling is the use of AI to optimize and coordinate the production process and to balance the demand and supply of resources, such as materials, machines, labor, and energy. It uses machine learning, deep learning, and reinforcement learning models that can forecast and simulate the demand and supply of resources. Production planning and scheduling provide optimal and flexible production plans and schedules that can maximize efficiency, productivity, and profitability. They adapt to changing conditions and constraints, such as customer orders, inventory levels, market trends, and environmental factors.
Benefits of AI-Powered Production Planning and Scheduling
- Reduced production costs: AI-optimized schedules lead to less waste, fewer disruptions, and efficient resource utilization, ultimately reducing production costs.
- Improved on-time delivery: Agile scheduling and accurate forecasts ensure products are delivered to customers on time, boosting customer satisfaction and brand reputation.
- Increased production capacity: AI-driven optimization can maximize resource utilization and identify previously unseen production efficiencies, leading to increased production capacity.
- Enhanced decision-making: Data-driven insights empower manufacturers to make informed decisions about resource allocation, inventory levels, and production schedules, fostering a culture of data-driven manufacturing.
AI-Powered Supply Chain Management
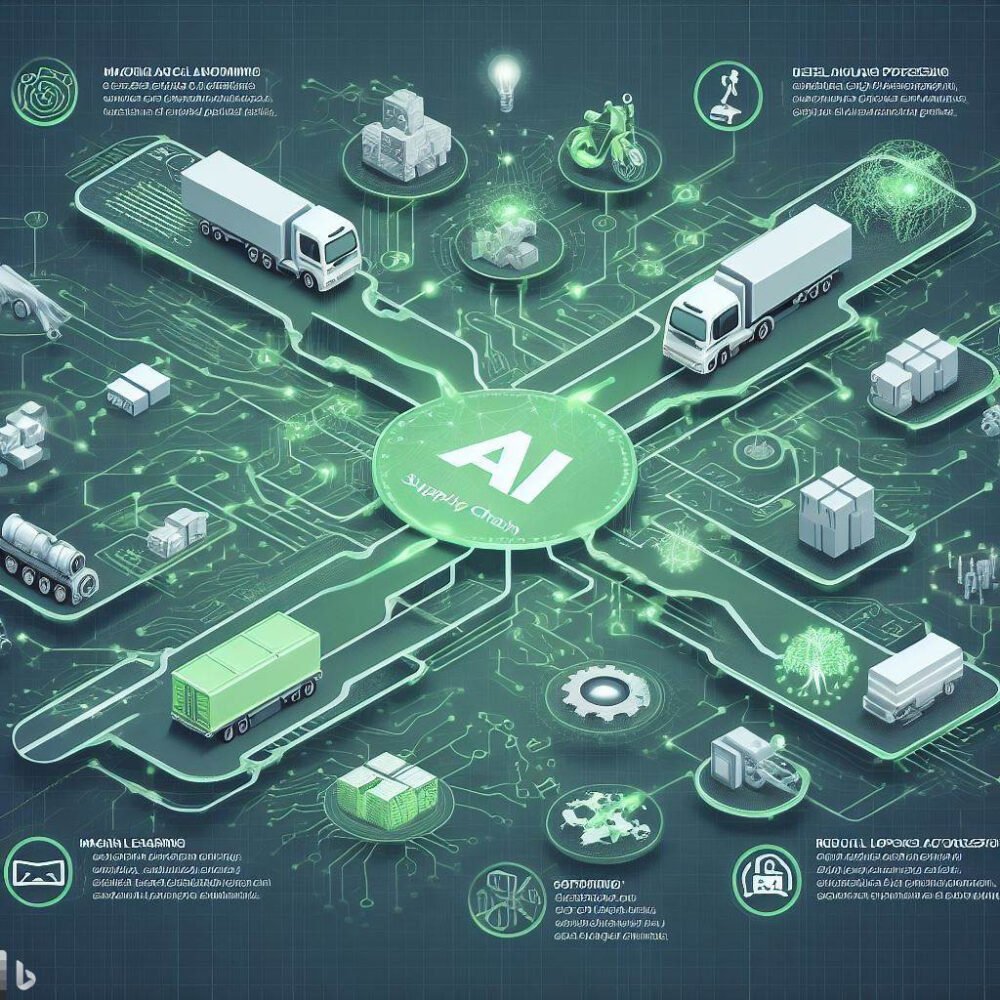
What is AI in Supply Chain Management?
Supply chain management is the use of AI to manage and optimize the flow of goods and services, from the source to the customer, and to enhance the visibility and transparency of the supply chain network. To achieve efficient supply chain management, you can use machine learning, deep learning, and natural language processing models. These models can analyze and process data from multiple sources, like suppliers, distributors, retailers, and customers, and provide insights and recommendations to enhance supply chain performance, reduce risks, and cut costs. Additionally, robotic process automation and intelligent automation can automate and streamline tasks like ordering, invoicing, tracking, and delivery. This can help improve accuracy and compliance, ultimately leading to a more efficient supply chain management system. Read more about AI in Logistics for more details.
Benefits of AI-Powered Supply Chain Management
- Increased profitability: AI optimizes costs, reduces waste, and improves efficiency, leading to higher profitability for all stakeholders in the supply chain.
- Enhanced customer satisfaction: Accurate demand forecasting and efficient logistics ensure timely delivery and product availability, boosting customer satisfaction and loyalty.
- Improved environmental sustainability: Optimizing operations and reducing waste through AI contributes to a more sustainable supply chain with a smaller environmental footprint.
- Greater agility and resilience: AI empowers companies to adapt quickly to changing market conditions and unforeseen disruptions, ensuring operational resilience.
AI in Manufacturing: Product Design and Development
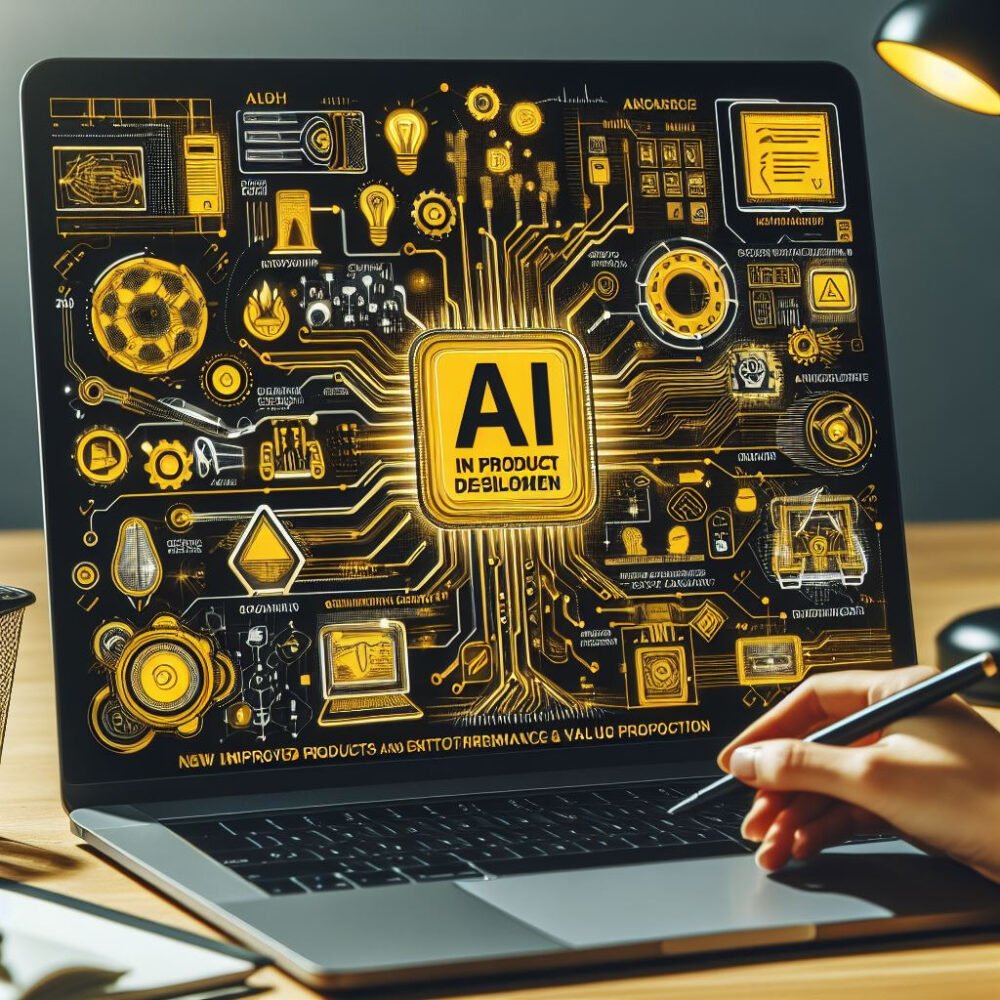
What is AI in Product Design and Development?
Product design and development is the use of AI to create and innovate new and improved products and services and to enhance the customer experience and value proposition. To achieve product design and development goals, you can use generative adversarial networks, deep learning, and natural language processing models. These models can generate and evaluate new designs and products, inspire creativity and innovation, and help improve functionality, usability, and aesthetics of products and services. Additionally, natural language processing and machine learning models can analyze textual data, such as documents, reports, reviews, and feedback, to provide insights and recommendations that meet the needs and preferences of customers.
Benefits of AI in Product Design and Development
- Shorter design cycles: AI can rapidly generate a plethora of design options, accelerating the iterative process and speeding up time-to-market.
- Enhanced innovation: By exploring unconventional design paths, AI can lead to breakthrough solutions that might have been missed by human designers.
- Improved performance and functionality: AI algorithms can optimize designs for specific criteria, such as weight, strength, or efficiency, resulting in superior products.
Process Optimization
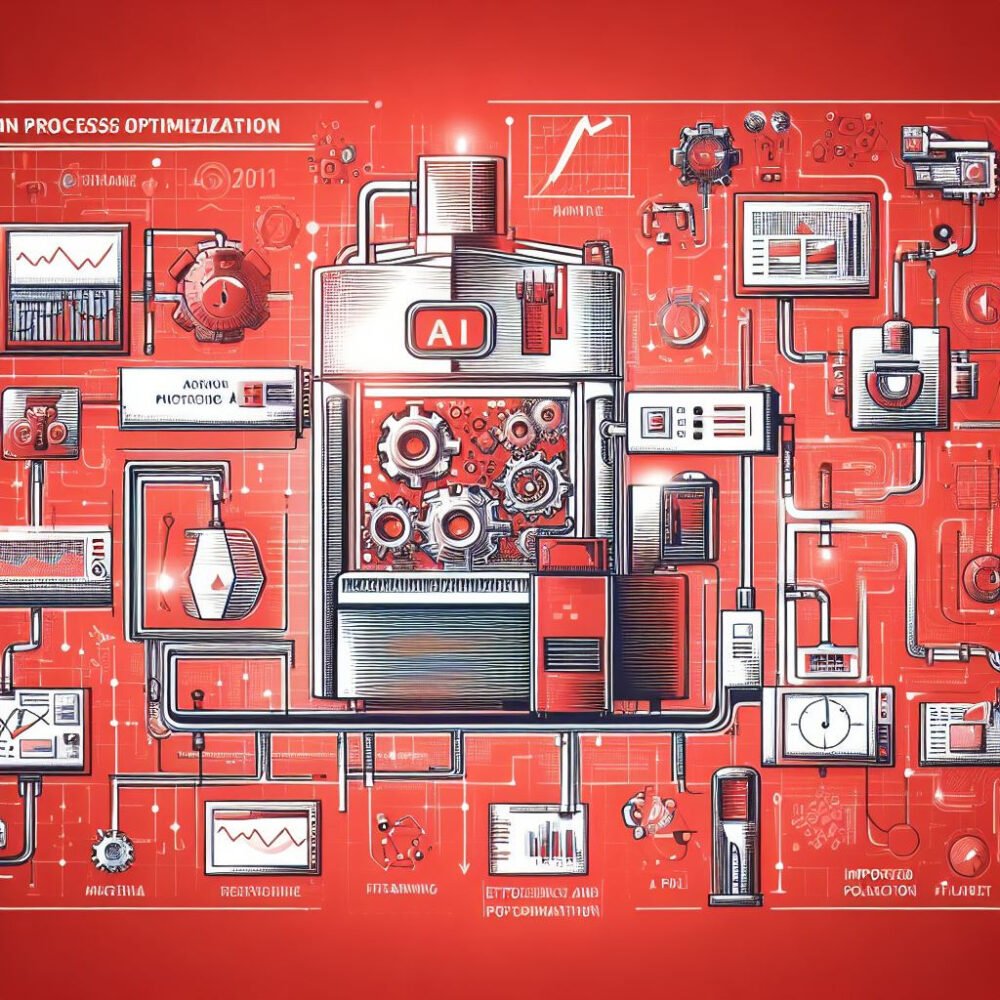
What is Process Optimization?
Process optimization is the use of AI to improve and enhance the efficiency and effectiveness of various processes and operations, and to reduce waste and variability. You can achieve this by utilizing machine learning, deep learning, and reinforcement learning models. These models can analyze and optimize different parameters and variables, including temperature, pressure, speed, and time. The result is that you can get optimal and robust solutions and actions that can improve the quality and productivity of your processes and operations, and that can handle uncertainties and disturbances.
Benefits of Process Optimization
The benefits of process optimization can be significant, including:
- Increased efficiency and productivity
- Reduced costs and waste
- Improved quality and customer satisfaction
- Enhanced competitiveness and agility
AI in Manufacturing: Safety and Security
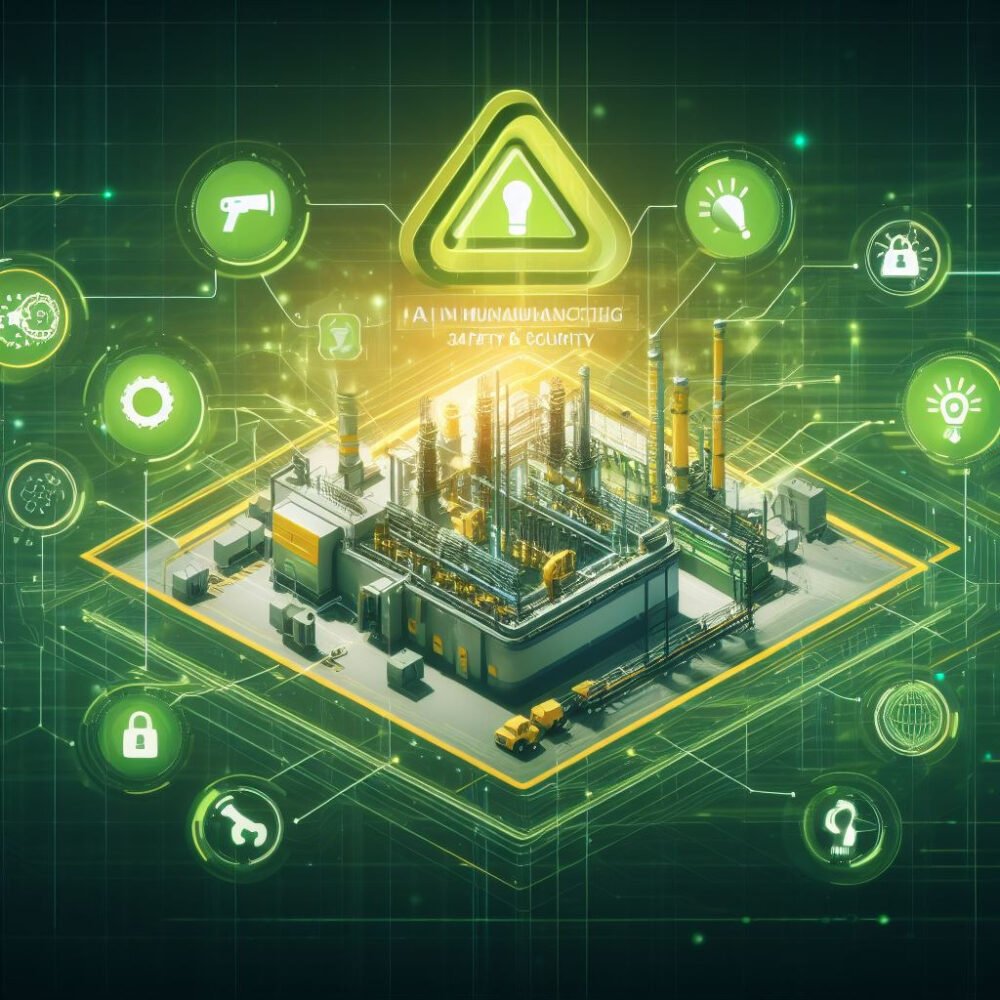
What is AI in Manufacturing Safety and Security?
Safety and security are the use of AI to protect and prevent the health and well-being of workers, machines, and the environment, and to detect and respond to threats and incidents. To achieve safety and security, we can use computer vision, deep learning, and natural language processing models to monitor and analyze the behavior and condition of workers, machines, and the environment. These models can identify and alert potential hazards, accidents, and intrusions, and provide guidance and assistance to mitigate and resolve them. Additionally, machine learning, deep learning, and reinforcement learning models can learn and enforce safety and security policies and protocols, and adapt and improve them over time.
Benefits of AI in Manufacturing: Safety and Security
- Real-time risk assessment and anomaly detection: AI systems can monitor work environments in real-time, identifying potential hazards such as fatigue, unsafe practices, or environmental anomalies before they lead to accidents.
- Enhanced worker safety equipment: AI-powered wearables can monitor vital signs, detect unsafe posture, and trigger alerts in case of emergencies, protecting workers from harm.
- Autonomous safety robots: Robots equipped with AI can perform dangerous tasks in hazardous environments, minimizing human exposure to risks.
- Cybersecurity defense: AI can analyze network activity and identify suspicious patterns, preventing cyberattacks that could disrupt production or compromise sensitive data.
- Physical security monitoring: AI-powered cameras and sensors can automatically detect intruders, unauthorized access, and potential security threats, deterring criminal activity and protecting facilities.
- Inventory control and tracking: AI systems can track inventory in real-time, preventing theft and ensuring product authenticity, especially for valuable or high-demand items.
- Supply chain security: AI can analyze data from various points in the supply chain, identifying potential vulnerabilities and mitigating risks of fraud, counterfeiting, or disruptions.
AI in Manufacturing: Customer Service
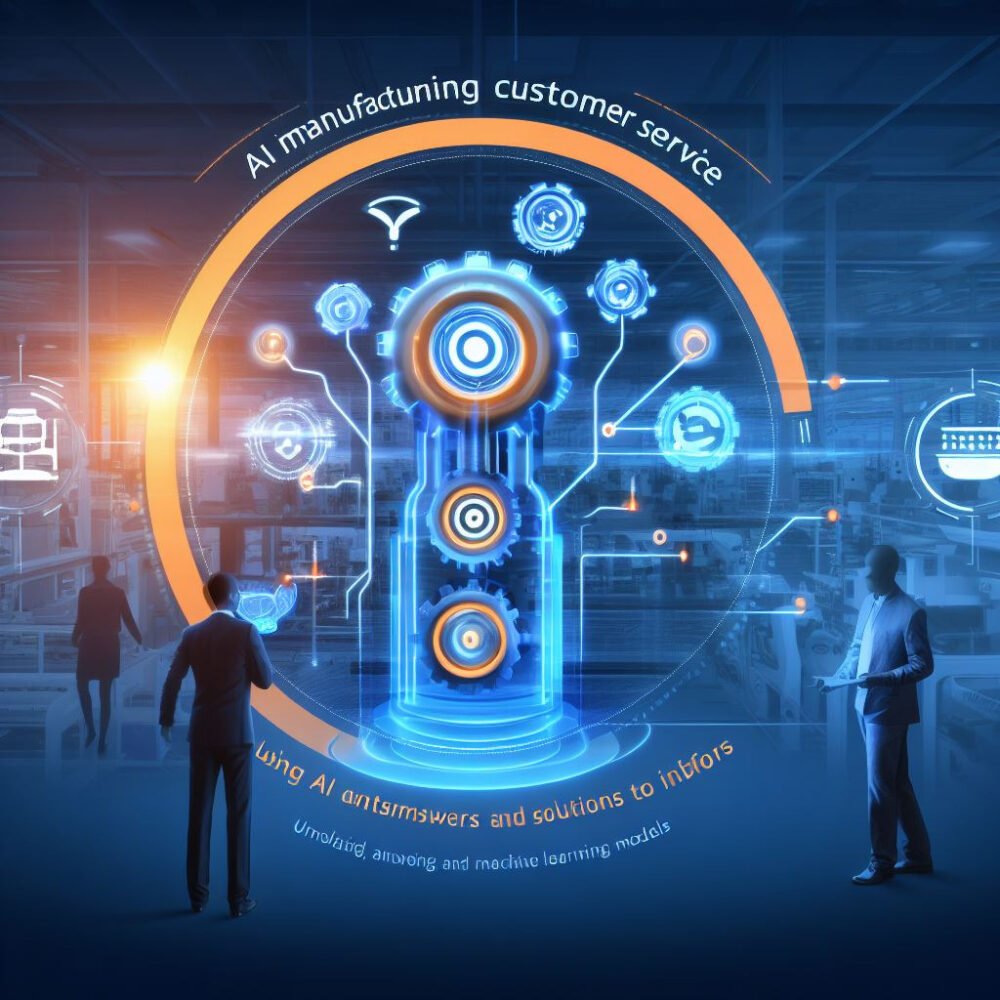
What is AI in Manufacturing Customer Service?
Customer service is the use of AI to provide and improve the service and support to customers, and to enhance customer loyalty and retention. To improve customer service, businesses can use natural language processing, machine learning, and deep learning models. These models can communicate with customers via voice assistants, chatbots, and translators, providing them with information, answers, and solutions. Additionally, businesses can use natural language processing and machine learning models to analyze and extract information from textual data such as documents, reports, reviews, and feedback. This process can provide insights and recommendations to improve the customer experience, satisfaction, and personalization of products and services to meet customer needs and preferences.
Benefits of AI in Manufacturing Customer Service
- 24/7 Support: AI-powered chatbots and virtual assistants provide instant assistance to customers anytime, anywhere, eliminating the need for human agents during off-hours or peak periods.
- Self-service Portals: AI-powered knowledge bases and FAQs empower customers to find answers to their questions on their own, freeing up human agents for more complex issues.
- Streamlined Communication: AI can automate routine tasks like order confirmations, shipping updates, and warranty registration, minimizing turnaround times and improving customer satisfaction.
Challenges of implementing AI in Manufacturing
AI in manufacturing offers many advantages and opportunities, but it also poses some challenges and risks that need to be considered and addressed. Some of the most common and significant challenges and risks of implementing AI in manufacturing are:
Lack / Shortage of Data
One of the challenges of implementing AI in manufacturing is the lack or shortage of data that can feed and fuel the AI solutions and systems. Data is essential for AI to learn from and perform various tasks and functions, such as sensing, learning, reasoning, and decision-making. Manufacturing data can be biased, outdated, and error-prone. Harsh operating conditions and weak data governance contribute to this. Proprietary systems also play a role. Manufacturing data is often localized and specific to a company’s operations. This limits the transferability of AI models and insights. Generalization is also hindered by domain-specific manufacturing data.
Manufacturers must collect and process diverse data. This includes images, videos, text, and audio. Data quality must be ensured. Data cleaning, enrichment, and augmentation techniques are used for this. A single source of information must be created and shared across the organization. Collaboration and data exchange with other manufacturers and stakeholders is necessary.
The aim is to create a universal and standardized industrial data set. This will help overcome the challenge posed by the increasing complexity of manufacturing processes. Multimodal data collection and processing is key to achieving this goal. Data quality is critical for accurate analysis and decision-making. Data-cleaning and enrichment techniques can help improve data quality. Data augmentation can help increase the amount of available data. A single source of information can ensure consistency across the organization. Collaborating with other manufacturers and stakeholders can lead to a universal industrial data set. Standardization of data is important for successful data exchange. A universal industrial data set can help manufacturers make better decisions. It can also improve production efficiency and reduce costs.
Lack of Skilled Workers
AI in manufacturing requires a workforce that has the skills and knowledge to design, develop, implement, and manage AI solutions and systems, and to work alongside them. However, there is a shortage of skilled workers in the field of AI, especially in manufacturing, as it is a relatively new and complex domain that requires a combination of technical, analytical, and soft skills. According to a report by Deloitte and The Manufacturing Institute, the US manufacturing industry could face a gap of up to 2.4 million workers by 2028, due to the lack of talent in emerging technologies, such as AI.
To overcome this challenge, manufacturers need to invest in training and education programs, both internally and externally, to upskill and reskill their existing and potential workers, and to attract and retain talent in the field of AI. Manufacturers also need to collaborate with academic institutions, research organizations, and industry associations, to create and promote curricula and certifications that can prepare and qualify workers for AI-related roles and tasks.
Data Security and Privacy Concerns
AI in manufacturing relies on large and diverse data sets, both structured and unstructured. The data comes from various sources, such as machines, equipment, sensors, IoT devices, suppliers, distributors, retailers, and customers. This raises concerns about security and privacy. The data may contain sensitive information, such as intellectual property and personal data. It may be vulnerable to cyberattacks, theft, loss, corruption, or misuse by malicious actors.
To address this challenge, strict data security and privacy policies and protocols are necessary. These policies can protect the data and comply with relevant laws and regulations. Manufacturers should use encryption, authentication, and authorization techniques. This will ensure that only authorized and legitimate parties can access and use the data. They should monitor and audit data activities and transactions to detect and prevent any anomalies or breaches.
Cost of Implementation
AI in manufacturing requires a significant amount of investment and resources, both financial and technical, to implement and maintain. It involves the acquisition and integration of various hardware and software components. For example, it requires the acquisition of sensors, IoT devices, computers, servers, cloud services, networks, platforms, applications, and tools. These tools enable and support AI solutions and systems. AI in manufacturing also involves the collection and processing of large and complex data sets. This requires a lot of storage, bandwidth, and computing power, to perform the AI tasks and operations. AI in manufacturing also involves the testing and evaluation of AI solutions and systems. Thus, it requires a lot of time and effort to ensure the quality, accuracy, and reliability of the AI outcomes.
Manufacturers must assess ROI and value proposition of AI solutions. Compare costs and benefits of implementation. Leverage existing resources and infrastructure. Utilize external sources of funding and support. Seek grants, subsidies, partnerships, and collaborations to share the cost and risk.
Ethical Implications
AI in manufacturing has the potential to create and improve products and services that can benefit society and the environment. It has the potential to cause and exacerbate the problems and issues that can harm society and the environment. AI in manufacturing can raise some ethical questions and dilemmas, such as:
- Who is responsible and accountable for the AI outcomes and outputs, and for the AI errors and failures, and how can they be measured and evaluated?
- How can the AI solutions and systems be transparent and explainable, and how can they be verified and validated, to ensure the trust and confidence of the stakeholders and the users?
- How can the AI solutions and systems be fair and unbiased, and how can they be inclusive and diverse, to ensure the respect and dignity of the stakeholders and the users?
- How can the AI solutions and systems be aligned and compatible with human values and norms, and how can they be ethical and moral, to ensure the well-being and welfare of the stakeholders and the users?
To address this challenge, manufacturers need to adopt and adhere to ethical principles and guidelines. Manufacturers also need to engage and consult with relevant and diverse stakeholders and experts. Customers, workers, regulators, ethicists, and civil society will help identify and understand the ethical implications. Additionally, they help identify expectations of the AI solutions and incorporate them in the AI design and implementation. Manufacturers also need to monitor and evaluate the ethical impact and performance of AI solutions. Moreover, they need to revise and improve them accordingly, to ensure the ethical and sustainable use of AI in manufacturing.
Case studies of successful AI implementations in Manufacturing
AI in manufacturing is not a hypothetical or futuristic scenario. It is a reality and a necessity for many manufacturers around the world. Many of them have successfully implemented and leveraged AI solutions and systems to achieve their goals and objectives, and to gain a competitive edge in the market. Here are some of the examples and case studies of successful AI implementations in manufacturing:
AI in Manufacturing Use Case 1: Tesla
Tesla is one of the leading and most innovative companies. Tesla is in the field of electric vehicles, battery energy storage, solar panels, and solar roofs. It is also one of the pioneers and adopters of AI in manufacturing. It uses AI to design, produce, and improve its products and services. Additionally, it enhances its customer experience and value proposition with AI. Tesla uses AI to:
- Create and optimize its battery cells, using machine learning and deep learning models. Those models analyze and process data from various sources, such as materials, chemistry, physics, and engineering. They provide optimal and robust solutions and designs that can improve the performance, efficiency, and cost of the battery cells.
- Develop and deploy its self-driving cars, using computer vision, deep learning, and reinforcement learning models. The models collect and process data from various sensors and cameras. They then enable the cars to perceive and understand their environment and to navigate and control themselves autonomously and safely.
- Enhance and personalize its customer service, using natural language processing, machine learning, and deep learning models. They can communicate and interact with customers, such as voice assistants, chatbots, and translators. Additionally, they can provide information, answers, and solutions to customer queries and issues. Moreover, they can also provide feedback, suggestions, and updates to customers, based on their needs and preferences.
AI in Manufacturing Use Case 2: Siemens
Siemens is one of the largest and most diversified companies in the field of engineering and technology, with products and services in various domains and industries, such as energy, healthcare, transportation, and manufacturing. It is also one of the leaders and innovators of AI in manufacturing, as it uses AI to enhance and automate various aspects of its manufacturing process, such as design, production, quality control, maintenance, and logistics. Siemens uses AI to:
- Design and optimize its gas turbines, using generative adversarial networks and machine learning models that can generate and evaluate new and novel designs and configurations, and that can provide optimal and efficient solutions and designs that can improve the performance, reliability, and sustainability of the gas turbines.
- Produce and inspect its circuit boards, using computer vision and machine learning models that can inspect and verify the quality and consistency of the circuit boards, identify and classify defects and errors, and provide feedback, corrections, and improvements to enhance the quality standards and customer satisfaction.
- Maintain and monitor its machines and equipment, using predictive maintenance and machine learning models that can monitor and analyze the condition and performance of the machines and equipment, that can predict and prevent failures and breakdowns before they occur, and provide alerts, recommendations, and actions to optimize the maintenance schedules and procedures.
AI in Manufacturing Use Case 3: Boeing
Boeing is one of the largest and most renowned companies in the field of aerospace and aviation, with products and services in various domains and industries, such as commercial airplanes, defense, space, and security. It is also one of the early adopters and users of AI in manufacturing, as it uses AI to improve and enhance various aspects of its manufacturing process, such as design, production, quality control, and safety. Boeing uses AI to:
- Design and test its airplanes, using machine learning and deep learning models that can analyze and process data from various sources, such as aerodynamics, physics, and engineering, and that can provide optimal and robust solutions and designs that can improve the performance, efficiency, and safety of the airplanes.
- Produce and assemble airplanes, using robotic process automation and intelligent automation that can automate and streamline various tasks and processes, such as drilling, riveting, painting, and wiring, and that can improve the accuracy, speed, and quality of the production and assembly.
- Inspect and verify its airplanes, using computer vision and machine learning models that can inspect and verify the quality and consistency of the airplanes, identify and classify defects and errors, and provide feedback, corrections, and improvements to enhance the quality standards and customer satisfaction.
AI in Manufacturing Use Case 5: General Electric
General Electric is one of the largest and most diversified companies in the field of engineering and technology, with products and services in various domains and industries, such as power, aviation, healthcare, and renewable energy. It is also one of the early adopters and users of AI in manufacturing, as it uses AI to enhance and automate various aspects of its manufacturing process, such as design, production, quality control, and maintenance. General Electric uses AI to:
- Design and optimize its jet engines, using machine learning and deep learning models that can analyze and process data from various sources, such as aerodynamics, physics, and engineering, and that can provide optimal and robust solutions and designs that can improve the performance, efficiency, and safety of the jet engines.
- Produce and inspect its wind turbines, using computer vision and machine learning models that can inspect and verify the quality and consistency of the wind turbine blades, that can identify and classify defects and errors, and provide feedback, corrections, and improvements to enhance the quality standards and customer satisfaction.
- Maintain and monitor its power plants, using predictive maintenance and machine learning models that can monitor and analyze the condition and performance of the power plant equipment, that can predict and prevent failures and breakdowns before they occur, and provide alerts, recommendations, and actions to optimize the maintenance schedules and procedures
AI in Manufacturing Use Case 6: Ford Motor Company
Ford Motor Company is one of the leading and most innovative companies in the field of automotive and mobility, with products and services in various domains and industries, such as cars, trucks, SUVs, electric vehicles, and self-driving vehicles. It is also one of the pioneers and adopters of AI in manufacturing, as it uses AI to design, produce, and improve its products and services, and to enhance its customer experience and value proposition. Ford Motor Company uses AI to:
- Create and optimize its battery cells, using machine learning and deep learning models that can analyze and process data from various sources, such as materials, chemistry, physics, and engineering, and that can provide optimal and robust solutions and designs that can improve the performance, efficiency, and cost of the battery cells.
- Develop and deploy its self-driving cars, using computer vision, deep learning, and reinforcement learning models that can collect and process data from various sensors and cameras, and that can enable the cars to perceive and understand their environment and to navigate and control themselves autonomously and safely.
- Enhance and personalize its customer service, using natural language processing, machine learning, and deep learning models that can communicate and interact with customers, such as voice assistants, chatbots, and translators, and that can provide information, answers, and solutions to customer queries and issues, and that can also provide feedback, suggestions, and updates to customers, based on their needs and preferences.
AI in Manufacturing Use Case 7: NVIDIA
NVIDIA is one of the largest and most influential companies in the field of computer graphics, gaming, and artificial intelligence, with products and services in various domains and industries, such as GPUs, chipsets, software, platforms, and cloud services. It is also one of the leaders and innovators of AI in manufacturing, as it uses AI to enhance and automate various aspects of its manufacturing process, such as design, production, quality control, and logistics. NVIDIA uses AI to:
- Design and optimize its GPUs, using machine learning and deep learning models that can analyze and process data from various sources, such as architecture, performance, and power, and that can provide optimal and efficient solutions and designs that can improve the performance, efficiency, and quality of the GPUs.
- Produce and inspect its chipsets, using computer vision and machine learning models that can inspect and verify the quality and consistency of the chipsets, that can identify and classify defects and errors, and provide feedback, corrections, and improvements to enhance the quality standards and customer satisfaction.
- Manage and optimize its supply chain, using machine learning, deep learning, and natural language processing models that can analyze and process data from various sources, such as suppliers, distributors, retailers, and customers, and that can provide insights and recommendations to improve the performance and efficiency of the supply chain and to reduce risks and costs.
AI in Manufacturing Use Case 8: IBM
IBM is one of the oldest and most reputable companies in the field of information technology, with products and services in various domains and industries, such as software, hardware, cloud, consulting, and research. It is also one of the pioneers and providers of AI in manufacturing, as it uses AI to enhance and automate various aspects of its own manufacturing process, such as design, production, quality control, and maintenance, and as it offers AI solutions and systems to other manufacturers, such as platforms, applications, and tools. IBM uses AI to:
- Design and optimize its microprocessors, using machine learning and deep learning models that can analyze and process data from various sources, such as architecture, performance, and power, and that can provide optimal and efficient solutions and designs that can improve the performance, efficiency, and quality of the microprocessors.
- Produce and inspect its circuit boards, using computer vision and machine learning models that can inspect and verify the quality and consistency of the circuit boards, identify and classify defects and errors, and provide feedback, corrections, and improvements to enhance the quality standards and customer satisfaction.
- Maintain and monitor its machines and equipment, using predictive maintenance and machine learning models that can monitor and analyze the condition and performance of the machines and equipment, that can predict and prevent failures and breakdowns before they occur, and provide alerts, recommendations, and actions to optimize the maintenance schedules and procedures.
AI in Manufacturing Use Case 9: PTC
PTC is one of the leading and most specialized companies in the field of industrial software, with products and services in various domains and industries, such as computer-aided design, product lifecycle management, internet of things, augmented reality, and artificial intelligence. It is also one of the leaders and providers of AI in manufacturing, as it uses AI to enhance and automate various aspects of its own manufacturing process, such as design, production, quality control, and maintenance, and as it offers AI solutions and systems to other manufacturers, such as platforms, applications, and tools. PTC uses AI to:
- Design and optimize its products, using generative design and machine learning models that can generate and evaluate new and novel designs and products, and that can provide optimal and efficient solutions and designs that can improve the performance, functionality, and usability of the products.
- Produce and assemble its products, using robotic process automation and intelligent automation that can automate and streamline various tasks and processes, such as drilling, riveting, painting, and wiring, and that can improve the accuracy, speed, and quality of the production and assembly.
- Inspect and verify its products, using computer vision and machine learning models that can inspect and verify the quality and consistency of the products, that can identify and classify defects and errors, and that can provide feedback, corrections, and improvements to enhance the quality standards and customer satisfaction.
AI in Manufacturing Use Case 10: Dassault Systèmes
Dassault Systèmes is one of the largest and most diversified companies in the field of software and technology, with products and services in various domains and industries, such as 3D design, simulation, engineering, and manufacturing. It is also one of the leaders and providers of AI in manufacturing, as it uses AI to enhance and automate various aspects of its own manufacturing process, such as design, production, quality control, and maintenance, and as it offers AI solutions and systems to other manufacturers, such as platforms, applications, and tools. Dassault Systèmes uses AI to:
- Design and optimize its products, using generative design and machine learning models that can generate and evaluate new and novel designs and products, and that can provide optimal and efficient solutions and designs that can improve the performance, functionality, and usability of the products.
- Produce and inspect its products, using computer vision and machine learning models that can inspect and verify the quality and consistency of the products, that can identify and classify defects and errors, and can provide feedback, corrections, and improvements to enhance the quality standards and customer satisfaction.
- Maintain and monitor its machines and equipment, using predictive maintenance and machine learning models that can monitor and analyze the condition and performance of the machines and equipment, that can predict and prevent failures and breakdowns before they occur, and provide alerts, recommendations, and actions to optimize the maintenance schedules and procedures.
AI in Manufacturing Use Case 11: Rockwell Automation
Rockwell Automation is one of the leading and most specialized companies in the field of industrial automation and information, with products and services in various domains and industries, such as control systems, software, sensors, and services. It is also one of the leaders and providers of AI in manufacturing, as it uses AI to enhance and automate various aspects of its own manufacturing process, such as design, production, quality control, and maintenance, and as it offers AI solutions and systems to other manufacturers, such as platforms, applications, and tools. Rockwell Automation uses AI to:
- Design and optimize its control systems, using machine learning and deep learning models that can analyze and process data from various sources, such as architecture, performance, and power, and that can provide optimal and efficient solutions and designs that can improve the performance, efficiency, and quality of the control systems.
- Produce and inspect its sensors, using computer vision and machine learning models that can inspect and verify the quality and consistency of the sensors, that can identify and classify defects and errors, and provide feedback, corrections, and improvements to enhance the quality standards and customer satisfaction.
- Maintain and monitor its machines and equipment, using predictive maintenance and machine learning models that can monitor and analyze the condition and performance of the machines and equipment, that can predict and prevent failures and breakdowns before they occur, and provide alerts, recommendations, and actions to optimize the maintenance schedules and procedures.
AI in Manufacturing Use Case 12: ABB
ABB is one of the largest and most diversified companies in the field of engineering and technology, with products and services in various domains and industries, such as power, robotics, automation, and electrification. It is also one of the leaders and innovators of AI in manufacturing, as it uses AI to enhance and automate various aspects of its manufacturing process, such as design, production, quality control, and maintenance. ABB uses AI to:
- Design and optimize its robots. ABB uses machine learning and deep learning models to analyze and process data from various sources. Data such as kinematics, dynamics, and control. Additionally, AI can provide optimal and efficient solutions and designs to improve the performance, efficiency, and quality of the robots.
- Produce and assemble its products, using robotic process automation and intelligent automation. AI can automate and streamline various tasks and processes, such as welding, painting, picking, and placing. Moreover, It can improve the accuracy, speed, and quality of the production and assembly.
- Inspect and verify its products, using computer vision and machine learning models. AI inspects and verifies the quality and consistency of the products. It identifies and classifies defects and errors, and provides feedback, corrections, and improvements to enhance quality standards and customer satisfaction.
These are some of the examples and case studies of successful AI implementations in manufacturing. These case studies can serve as inspiration and motivation for other manufacturers who want to implement and leverage AI in their manufacturing process to gain a competitive edge in the market.
In the next section, we will discuss the future of AI in manufacturing. How it will continue to transform and revolutionize the manufacturing industry. And, how it will have a significant impact on society and the environment.
The Future of AI in Manufacturing
AI in manufacturing is not a static or stagnant phenomenon. It is a dynamic and evolving one. AI will continue to transform and revolutionize the manufacturing industry. Additionally, it will have a significant impact on society and the environment. AI in manufacturing will create new opportunities and challenges. AI will require new skills and competencies. Additionally, it will raise new questions and dilemmas, that will need to be addressed and managed.
Trends and Predictions for the Future of AI in Manufacturing
- AI will become more pervasive and ubiquitous in manufacturing. It will be integrated and embedded in various products and systems. AI will integrate with smart robots, sensors, and devices. They can interact and collaborate with humans and the environment. Additionally, they can perform various tasks and functions, such as sensing, learning, reasoning, and decision-making.
- AI will become more advanced and sophisticated in manufacturing. It will use more complex and powerful techniques and technologies. AI will use deep learning, reinforcement learning, generative adversarial networks, and natural language processing to interpret more diverse and multimodal data. For example, It will process images, videos, text, and audio. It can perform more complex and dynamic tasks, such as creativity, innovation, and problem-solving.
- AI is also becoming more autonomous and adaptive in manufacturing. They use self-learning and self-improving techniques and technologies. For example, they will use reinforcement learning to learn from their own actions and feedback, optimize their behavior and performance, cope with uncertainties and disturbances, and achieve complex and dynamic goals. AI is being used to develop autonomous robots, predictive maintenance, and quality control systems in manufacturing.
- AI in manufacturing is becoming more human-centric and ethical, as it is using more human-aware and human-compatible techniques and technologies. These technologies, such as natural language processing, can communicate and interact with humans in a more natural way. They can also understand and respect human values and norms, and be transparent and explainable. This makes AI more accessible and understandable to humans and builds trust between humans and AI systems.
These are some of the trends and predictions for the future of AI in manufacturing. External and internal factors and forces that can influence and shape the future of AI in manufacturing.
How to get started with AI in Manufacturing
AI in manufacturing is a present and attainable goal for manufacturers of all sizes, sectors, and regions. It can provide many benefits and advantages, such as improved efficiency, quality, innovation, and customer satisfaction. It can also create many opportunities and possibilities, such as new products and services, and new markets and segments.
However, AI in manufacturing also requires some investment and resources, both financial and technical, and some preparation and planning, both strategic and operational. Here are some steps and tips on how to get started:
- Assess your needs and opportunities. What are your pain points? Where can AI make the biggest impact?
- Develop a strategy. What are your goals for AI? How will you measure success?
- Start small. Don’t try to do too much too soon. Focus on one or two areas where AI can have the biggest impact.
- Partner with experts. There are many companies that can help you implement AI in manufacturing.
- Be patient and persistent. AI takes time to implement and realize the benefits.
Assess Your Needs
How to Assess Needs and Objectives for AI in Manufacturing
- Analyze your current situation and performance. What are your strengths and weaknesses? What are your opportunities and threats? What are your key performance indicators (KPIs) and metrics?
- Analyze your customers and competitors. What are their needs and expectations? What are their strengths and weaknesses? What are their opportunities and threats? What is your value proposition and competitive advantage?
- Identify and prioritize the problems and issues that you want to solve and the goals and outcomes that you want to achieve, using AI. What are your biggest pain points? Where can AI make the biggest impact? What are your desired outcomes for AI?
Examples of Needs and Objectives for AI in Manufacturing
- Improve efficiency: Reduce production costs, cycle times, and waste.
- Improve quality: Reduce defects and improve product quality.
- Improve innovation: Accelerate product development and launch new products faster.
- Improve customer satisfaction: Deliver products on time and to customers’ specifications.
- Reduce costs: Reduce energy consumption, maintenance costs, and other expenses.
Once you have assessed your needs and objectives, you can start to develop a strategy for implementing AI in manufacturing.
Tips for Assessing the Needs and Objectives for AI in Manufacturing
- Be specific and measurable. What specific problems do you want to solve with AI? What specific goals do you want to achieve?
- Be realistic. AI is a powerful tool, but it can’t solve every problem. Consider your budget, resources, and capabilities when setting your goals.
- Be flexible. The field of AI is rapidly evolving, so be prepared to adjust your plans as needed.
- Get input from stakeholders. Talk to your employees, customers, and other stakeholders to get their input on your needs and objectives.
By following these tips, you can assess your needs and objectives for AI in manufacturing and develop a plan to achieve your goals.
Choose the Right AI Solutions
How to Choose the Right AI Solution for Manufacturing
- Research and explore the various types of AI techniques and technologies that are available and applicable. What are the strengths and weaknesses of each technology? How can each technology be used to solve your specific problems and achieve your goals?
- Select and combine the AI techniques and technologies that can best suit and serve your purpose and function. Consider your needs, objectives, budget, and resources when making your selection.
- Research and explore the various sources and providers of AI solutions and systems. What are the benefits and drawbacks of each vendor, platform, application, and tool? How well do each of them meet your requirements and specifications?
- Select and use the AI solutions and systems that can best fit and support your budget and resources. Consider the cost of the solutions and systems, as well as the cost of implementation and maintenance.
Tips for Choosing the Right AI Solution for Manufacturing
- Start by understanding your needs and objectives. What problems do you want to solve with AI? What goals do you want to achieve?
- Consider your budget and resources. How much money do you have available for AI? What skills and expertise do you have in-house?
- Evaluate different AI solutions and systems. Compare the features, pricing, and support options of different vendors and platforms.
- Choose a solution that is scalable. Your AI needs may grow over time, so choose a solution that can scale to meet your needs.
- Get buy-in from stakeholders. Talk to your employees, customers, and other stakeholders to get their input on the AI solution or system that you are considering.
By following these tips, you can choose the right AI solution and system for your manufacturing business.
Implement and Manage your AI Solution
How to Implement and Manage an AI Solution in Manufacturing
- Acquire and integrate the various hardware and software components. This may include sensors, IoT devices, computers, servers, cloud services, networks, platforms, applications, and tools. It is important to choose components that are compatible with each other and that can support the requirements of the AI solution and system.
- Collect and process the various data sets. AI solutions and systems need to be trained on data in order to learn and perform tasks. The data sets should be clean, organized, and relevant to the specific tasks that the AI solution and system will be performing.
- Test and evaluate the AI solution and system. This is important to ensure that the AI solution and system are working as expected and that it is producing accurate and reliable results.
- Monitor and manage the AI solution and system. Once the AI solution and system are deployed, it is important to monitor its performance and make adjustments as needed. This may involve retraining the AI solution and system on new data or updating the hardware and software components.
Tips for Implementing and Managing AI Solutions in Manufacturing:
- Start by developing a clear plan. This should include identifying the specific goals of the AI solution and system, the data sets that will be used, and the hardware and software components that will be required.
- Get buy-in from stakeholders. It is important to have the support of employees, managers, and customers before implementing an AI solution and system.
- Start small. It is better to start with a small, pilot project than to try to implement a large-scale AI solution and system all at once.
- Be patient. It takes time to implement and manage an AI solution and system effectively.
By following these tips, manufacturers can implement and manage AI solutions and systems that can help them improve their efficiency, quality, innovation, and customer satisfaction.
Measure the Results of your AI Solution
How to Measure the Results and Impact of an AI Solution in Manufacturing:
- Define and measure your key performance indicators (KPIs) and metrics. This may include things like production efficiency, product quality, customer satisfaction, and return on investment (ROI). It is important to choose KPIs and metrics that are relevant to your specific business goals. Those metrics need to be measured accurately and consistently.
- Collect data on your AI solution and system. This may include data on the accuracy of the AI system, the time it takes to complete tasks and the resources it consumes. It is important to collect data over a period of time to get a good understanding of the performance and impact of the AI solution and system.
- Analyze the data and identify trends. Once you have collected data, you can analyze it to identify trends and patterns. This can help you to understand how the AI solution and system is performing and how it is impacting your business.
- Compare the results with and without AI. It is helpful to compare the results of your AI solution and system to the results that you were achieving before you implemented AI. This can help you to quantify the impact of the AI solution and system on your business.
- Use the insights to improve your AI solution and system. The insights that you gain from measuring the results and impact of your AI solution and system can be used to improve the system and make it more effective.
Examples of KPIs and Metrics Used to Measure Results and Impact of AI in Manufacturing:
- Production efficiency: Increase in throughput, reduction in cycle time, reduction in waste
- Product quality: Reduction in defects, increase in customer satisfaction
- Customer satisfaction: Increase in customer satisfaction scores, reduction in customer complaints
- Return on investment (ROI): The ratio of the benefits of the AI solution and system to the costs of the AI solution.
- Cost savings: The amount of money saved by using the AI solution and system
By measuring the results and impact of AI in manufacturing, manufacturers can ensure that they are getting the most value from their AI investments.
Additional tips for measuring the results and impact of AI in manufacturing:
- Get buy-in from stakeholders. It is important to get buy-in from all stakeholders, including employees, managers, and customers, before implementing AI. This will help to ensure that the AI solution and system are used effectively. More importantly, the results are measured and reported accurately.
- Use a variety of data sources. The more data that you have, the better understanding you will have of the performance and impact of your AI solutions. Use a variety of data sources, including internal data, customer data, and industry data.
- Be patient. It takes time to measure the results and impact of AI. Don’t expect to see results overnight. Be patient and continue to collect data and analyze the results over time.
By following these tips, manufacturers can measure the results and impact of AI in manufacturing and ensure that they are getting the most value from their AI investments.
Conclusion
AI in manufacturing is a powerful and promising technology that can transform the industry. It can help manufacturers overcome challenges, achieve objectives, and gain a competitive edge in the market. However, it is important to be aware of the challenges and risks associated with AI. Some of these risks are the lack of skilled workers, data security and privacy concerns, and ethical implications.
To implement and leverage AI in manufacturing successfully and sustainably, manufacturers need to follow some steps and tips. They need to assess their needs, choose the right AI solution, implement and manage their AI solution, and measure the results of their AI solution.
AI in manufacturing can help manufacturers improve their efficiency, quality, innovation, and customer satisfaction. It can also help them create new products and services and explore new markets and opportunities.
Overall, AI in manufacturing is a powerful and promising technology with the potential to transform the industry. Manufacturers who are able to successfully implement and leverage AI will be well-positioned to succeed in the future.
We hope you enjoyed this article. If you have any questions or comments, please feel free to contact us, or to leave a comment below. We would love to hear from you and to learn from you. Thank you for reading, and happy AI-ing! 😊