Fraud is a pervasive and costly problem that affects every industry and sector. According to a report by PwC, global fraud losses reached $42 billion in 2020, up from $26 billion in 2018. Fraudsters are constantly devising new and sophisticated ways to exploit vulnerabilities and loopholes in systems and processes. To combat this threat, businesses must implement robust and effective fraud detection and prevention strategies.
One of the key solutions to fight fraud is automation. Automated fraud detection systems use advanced technologies such as machine learning, artificial intelligence, and data analytics to identify and prevent fraudulent activities in real-time. Automation can help businesses improve their efficiency, accuracy, scalability, and cost-effectiveness in fraud management. In this article, we will explore the concept of fraud detection, the types and methods of fraud detection, the benefits and challenges of automation, and the future trends and innovations in this field.
Key Takeaways
Key Area | Actionable Insights | Benefits |
---|---|---|
The Threat | Fraud is a $5.1 trillion global threat. | Understand the urgency of implementing robust fraud prevention. |
Automation Advantage | Automate to outpace evolving fraudsters. | Increase efficiency, accuracy, and scalability. – Reduce costs and false positives. |
Fraud Types | Learn about common fraud types like credit card fraud and insurance scams. | Tailor your fraud detection approach to specific vulnerabilities. |
Detection Process | Master the core steps: data collection, analysis, and investigation. | Build a comprehensive framework for fraud prevention. |
Industry Specfics | Explore credit card fraud detection with AI and machine learning. | Implement targeted solutions for insurance and financial fraud. |
Case Studies | Learn from success stories of fraud prevention with automation. | Industry Specifics |
The Future of Fraud | Embrace biometrics, blockchain, and behavioral analysis. | Stay ahead of the curve with cutting-edge technologies. |
Understanding Fraud Detection and Management
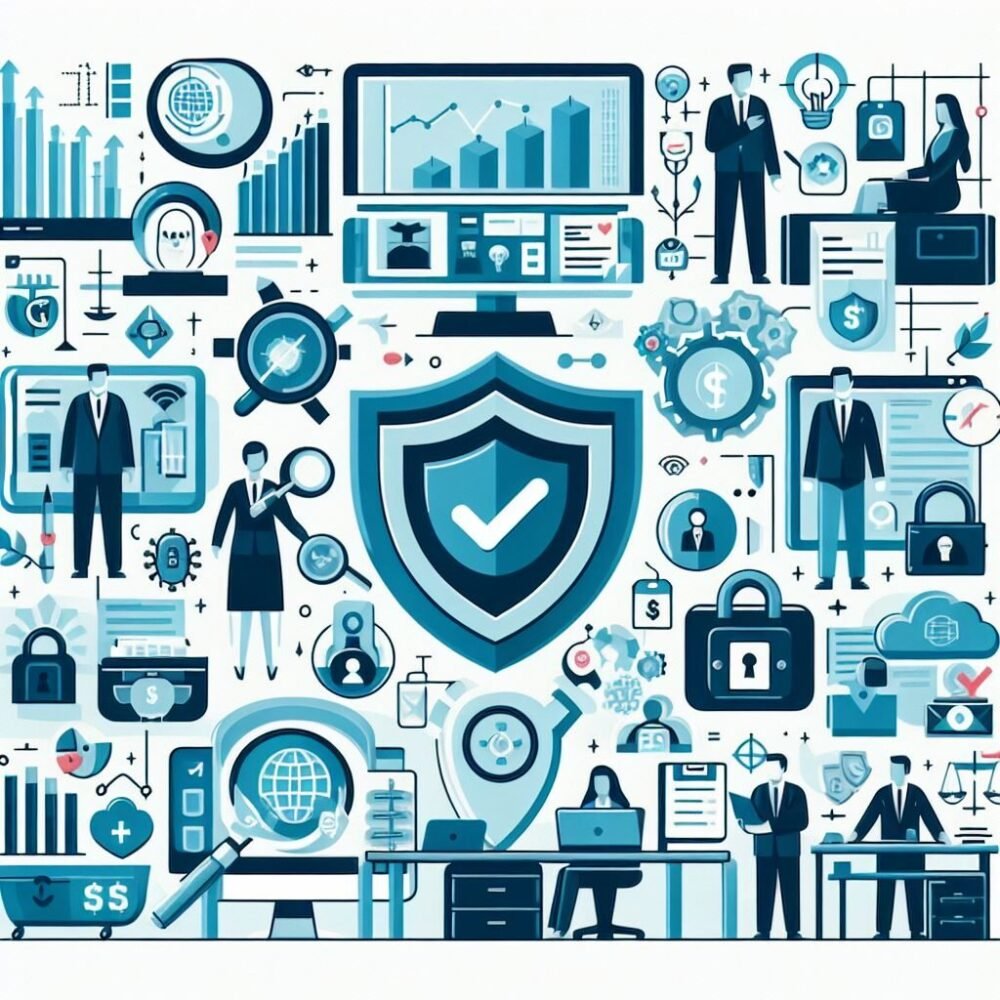
Fraud detection is the process of identifying and preventing fraudulent or suspicious transactions, activities, or behaviors that may cause financial or reputational harm to a business or its customers. Fraud detection is a crucial component of risk management, as it helps businesses mitigate losses, comply with regulations, and protect their brand image and customer trust.
How Does Fraud Affect Different Industries?
Various types of fraud can affect different industries and sectors, such as:
- Credit card fraud occurs when a fraudster uses stolen or counterfeit credit card or card information to make unauthorized purchases or withdrawals. Credit card fraud can result in chargebacks, fees, and penalties for merchants and card issuers, as well as identity theft and financial losses for cardholders.
- Insurance fraud involves making false or exaggerated claims to obtain undeserved benefits or compensation from an insurance company or policyholder. Insurance fraud can include staged accidents, property damage, injury, or death, as well as inflating or fabricating medical bills, receipts, or documents.
- Financial institution fraud refers to any fraudulent activity that targets banks, fintech companies, or other financial institutions or their customers. Financial institution fraud can include identity theft, money laundering, phishing, account takeover, wire fraud, check fraud, and loan fraud.
- Online fraud encompasses any fraudulent activity that occurs on the internet or through digital platforms or devices. Online fraud can include e-commerce fraud, cyberattacks, malware, ransomware, data breaches, and social engineering.
How to Implement a Fraud Detection Process?
The fraud detection process typically involves the following steps:
- Data collection involves gathering and storing relevant data from various sources, such as transactions, applications, accounts, devices, networks, and external databases. Data collection can be done in batch or in real-time, depending on the volume and velocity of the data.
- Data analysis involves applying various techniques and algorithms to analyze and process the data, such as data cleansing, normalization, transformation, aggregation, and segmentation. Data analysis can help identify patterns, trends, anomalies, outliers, and correlations in the data.
- Fraud detection involves using rules, models, scores, or indicators to flag or classify the data as fraudulent or non-fraudulent, based on predefined criteria or thresholds. Fraud detection can be done using supervised, unsupervised, or semi-supervised machine learning methods, depending on the availability and quality of the labeled data.
- Fraud investigation involves verifying and validating the flagged or classified data, using human intervention, feedback, or evidence. Fraud investigation can help confirm or reject the fraud detection results, as well as provide insights and recommendations for further action or improvement.
Fraud Detection Deep Dive
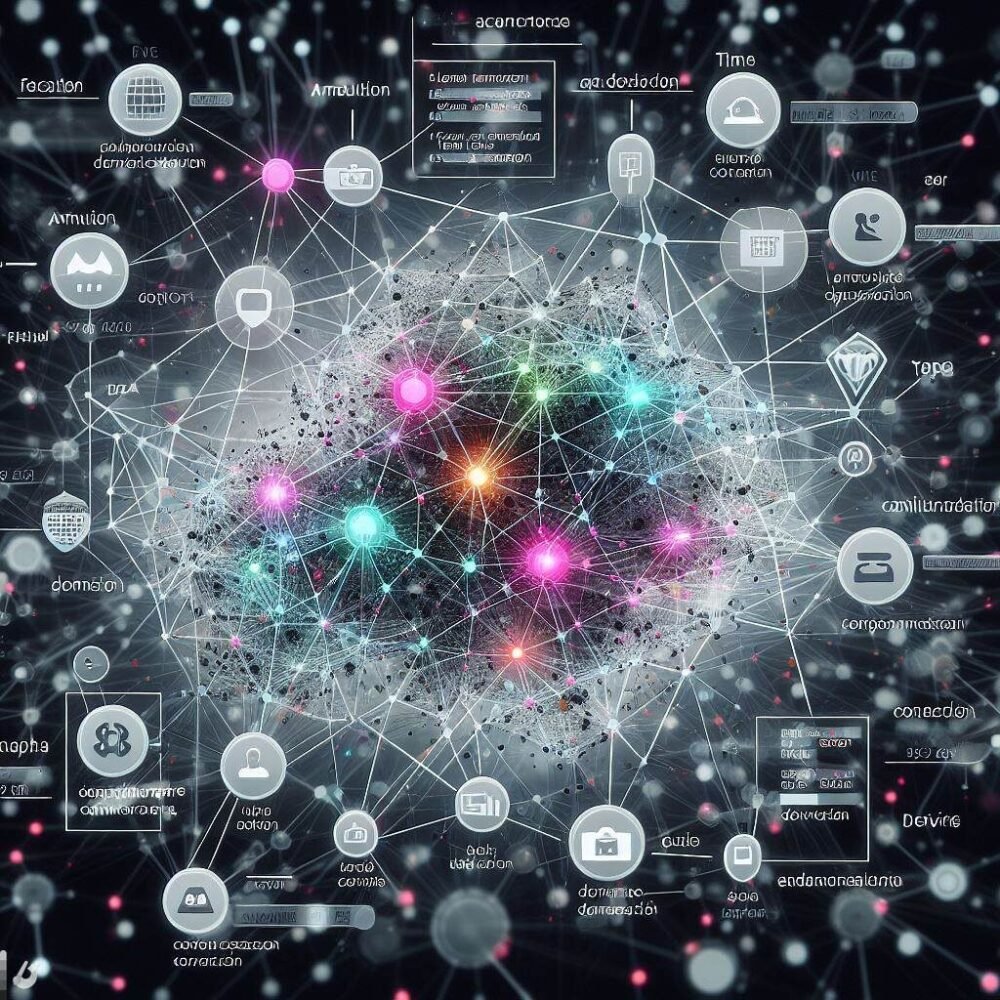
Each type of fraud requires specific methods and tools to detect and prevent it, depending on the nature, complexity, and scale of the fraud. In this section, we will dive deeper into some of the most common and challenging types of fraud and how automation can help address them.
Credit Card Fraud Detection
Credit card fraud is one of the most prevalent and costly types of fraud, especially with the rise of online and mobile payments. According to a report by Juniper Research, global online payment fraud losses are expected to reach $48 billion by 2023, up from $22 billion in 2020. Credit card fraud can take various forms, such as:
- Card-present fraud occurs when a fraudster uses a stolen or counterfeit physical card to make purchases or withdrawals at a point-of-sale (POS) terminal or an automated teller machine (ATM). Card-present fraud can be detected using techniques such as chip and PIN verification, magnetic stripe analysis, and hologram inspection.
- Card-not-present (CNP) fraud occurs when a fraudster uses stolen or counterfeit card information to make purchases or withdrawals online, over the phone, or by mail. CNP fraud can be detected using techniques such as address verification service (AVS), card verification value (CVV), and 3D secure authentication.
- Account takeover (ATO) fraud occurs when a fraudster gains unauthorized access to a cardholder’s account and makes purchases or withdrawals using the card or card information. ATO fraud can be detected using techniques such as behavioral biometrics, device fingerprinting, and geolocation.
AI in Credit Card Fraud Detection
Machine learning and artificial intelligence can play a vital role in enhancing credit card fraud detection, as they can help:
- Detect anomalies: Machine learning algorithms can learn from historical and real-time data to identify normal and abnormal patterns of transactions, activities, or behaviors, and flag any deviations or outliers that may indicate fraud.
- Generate risk scores: Machine learning models can assign a numerical value to each transaction, activity, or behavior, based on the probability or likelihood of fraud, and trigger alerts or actions based on predefined thresholds or rules.
- Adapt to new fraud patterns: Machine learning systems can continuously monitor and update their data, models, and parameters, and adapt to changing fraud trends and tactics, without requiring manual intervention or reprogramming.
You can read more about AI in Finance to learn more about how AI is revolutionizing the industry.
Insurance Fraud Detection
Insurance fraud is another major type of fraud that affects both insurers and policyholders. According to a report by the Coalition Against Insurance Fraud, insurance fraud costs the U.S. economy at least $80 billion per year, and the average U.S. household pays nearly $950 per year in higher premiums due to fraud. Insurance fraud can involve various schemes, such as:
- Claim fraud: This involves making false or exaggerated claims to obtain undeserved benefits or compensation from an insurance company or policyholder. Claim fraud can include staged accidents, property damage, injury, or death, as well as inflating or fabricating medical bills, receipts, or documents.
- Premium fraud: This involves misrepresenting or concealing information to obtain lower premiums or avoid paying premiums from an insurance company or policyholder. Premium fraud can include underreporting income, assets, or employees, as well as falsifying or omitting medical history, driving record, or credit score.
- Policy fraud: This involves obtaining or issuing fraudulent or invalid policies from an insurance company or policyholder. Policy fraud can include selling or buying fake or stolen policies, as well as impersonating or forging identities, signatures, or documents.
Predictive Analytics in Insurance Fraud Detection
Predictive analytics and data mining can help improve insurance fraud detection, as they can help:
- Extract features: Predictive analytics and data mining techniques can help extract relevant and meaningful features or attributes from large and complex data sets, such as claims, policies, customers, and providers, and reduce the dimensionality and noise in the data.
- Build models: Predictive analytics and data mining methods can help build predictive models or classifiers that can estimate the probability or likelihood of fraud for each claim, policy, or customer, based on historical and current data, and generate scores or labels accordingly.
- Evaluate performance: Predictive analytics and data mining tools can help evaluate the performance and accuracy of the models or classifiers, using metrics such as precision, recall, F1-score, and ROC curve, and optimize or refine them accordingly.
Image Recognition in Insurance Fraud Detection
Artificial intelligence and image recognition can also help enhance insurance fraud detection, especially for property and casualty insurance, as they can help:
- Analyze images: Artificial intelligence and image recognition systems can help analyze and process images of vehicles, buildings, or other objects that are involved in insurance claims, and extract relevant information, such as make, model, color, damage, or repair.
- Detect anomalies: Artificial intelligence and image recognition algorithms can help detect anomalies or inconsistencies in the images, such as mismatched parts, altered license plates, or tampered timestamps, and flag them as potential fraud indicators.
- Compare images: Artificial intelligence and image recognition tools can help compare images from different sources, such as claims, policies, databases, or social media, and identify similarities or differences that may suggest fraud, such as duplicate or recycled images, or conflicting or contradictory information.
Financial Institution Fraud Detection
Financial institution fraud is a broad and complex type of fraud that targets banks, fintech companies, or other financial institutions or their customers. According to a report by LexisNexis Risk Solutions, financial institution fraud cost the U.S. financial services industry $16.8 billion in 2020, up from $14.4 billion in 2018. Financial institution fraud can include various activities, such as:
- Identity theft: This involves stealing or misusing personal or financial information of a customer or an institution, such as name, address, social security number, account number, or password, to open or access accounts, make transactions, or obtain benefits or services.
- Money laundering: This involves transferring or concealing the source, ownership, or destination of illegally obtained
why would institutions automate their fraud detection process?
Automating fraud detection can bring significant benefits to businesses, such as:
- Improved efficiency and accuracy: Automated fraud detection systems can process large volumes of data faster and more accurately than human analysts, reducing the time and resources required for fraud detection and investigation.
- 24/7 real-time monitoring: Automated fraud detection systems can monitor transactions, activities, and behaviors in real-time, and detect and prevent fraud as soon as it occurs, minimizing the potential losses and damages.
- Scalability to handle large volumes of data: Automated fraud detection systems can scale up or down to handle fluctuations in data volume and complexity, without compromising the quality and performance of fraud detection.
- Reduced operating costs: Automated fraud detection systems can reduce the operating costs associated with fraud detection and management, such as labor, training, infrastructure, and maintenance costs.
- Proactive identification of new fraud patterns: Automated fraud detection systems can learn from new data and feedback, and adapt to changing fraud trends and tactics, without requiring manual intervention or reprogramming.
What are the challenges of automating the fraud detection process?
However, automation also comes with some challenges and limitations, such as:
- Data quality and availability: Automated fraud detection systems rely on the quality and availability of the data to perform effectively and accurately. Poor, incomplete, or inconsistent data can affect the performance and reliability of the fraud detection models and algorithms.
- Data security and privacy: Automated fraud detection systems need to ensure the security and privacy of the data they collect, store, and process, and comply with the relevant regulations and standards, such as the General Data Protection Regulation (GDPR) and the Payment Card Industry Data Security Standard (PCI DSS).
- Human oversight and intervention: Automated fraud detection systems still need human oversight and intervention to verify, validate, and explain the fraud detection results, as well as to handle complex or ambiguous cases that require human judgment or expertise.
Which tools can be used to automate the fraud detection process?
Choosing the right tools and software for automated fraud detection is crucial for achieving the desired outcomes and overcoming the challenges. There are various fraud detection tools and software available in the market, each with different features, functionalities, and capabilities. Some of the factors to consider when choosing a solution are:
- Industry needs: Different industries and sectors have different fraud detection needs and challenges, depending on the type, scale, and complexity of the fraud they face. For example, credit card fraud detection may require different tools and techniques than insurance fraud detection. Therefore, it is important to choose a solution that is tailored to the specific industry needs and requirements.
- Budget: The cost of implementing and maintaining an automated fraud detection system can vary depending on the features, functionalities, and capabilities of the solution, as well as the data volume and complexity, and the integration and customization needs. Therefore, it is important to choose a solution that fits the budget and provides the best value for money.
- Data security: The security and privacy of the data is a paramount concern for any business that deals with sensitive or confidential information, such as personal or financial data. Therefore, it is important to choose a solution that provides adequate data security and privacy measures, such as encryption, authentication, authorization, and auditing, and complies with the relevant regulations and standards, such as the GDPR and the PCI DSS.
Case Studies and Success Stories
To illustrate the benefits and effectiveness of automated fraud detection, here are some real-world examples of how businesses have used automated fraud detection to prevent losses and protect customers.
Case Study 1: PayPal
PayPal is a global online payment platform that enables users to send and receive money, make purchases, and access financial services. PayPal handles over 1 billion transactions per day, across 200 markets and 25 currencies. With such a large and diverse customer base and transaction volume, PayPal faces a high risk of fraud, such as account takeover, phishing, and money laundering.
To combat fraud, PayPal uses a combination of machine learning, artificial intelligence, and human expertise to detect and prevent fraudulent transactions and activities. PayPal’s fraud detection system consists of three main components:
- Fraud engine: This is the core component that analyzes and scores each transaction, activity, or behavior, based on the probability or likelihood of fraud, using various machine learning models and algorithms, such as neural networks, decision trees, and logistic regression. The fraud engine also learns from new data and feedback and adapts to changing fraud patterns and tactics.
- Fraud cockpit: This is the user interface that allows human analysts to monitor, review, and investigate the fraud detection results, using various tools and dashboards, such as alerts, reports, and graphs. The fraud cockpit also enables human analysts to provide feedback and input to the fraud engine and to escalate or resolve fraud cases.
- Fraud network: This is the network of external data sources and partners that provides additional information and insights to the fraud detection system, such as identity verification, device fingerprinting, and geolocation. The fraud network also helps PayPal collaborate and share best practices with other financial institutions and law enforcement agencies.
What Does PayPal Benefit from Automating Fraud Detection?
By using automated fraud detection, PayPal has been able to:
- Reduce fraud losses: PayPal has reduced its fraud losses to less than 0.3% of its total revenue, which is significantly lower than the industry average of 1.5%.
- Enhance customer experience: PayPal has improved its customer satisfaction and retention, by providing a fast, secure, and convenient payment service, and by resolving fraud issues quickly and effectively.
- Gain competitive advantage: PayPal has gained a competitive edge in the online payment market, by offering a reliable and trustworthy payment platform, and by innovating and expanding its products and services.
Case Study 2: Allstate
Allstate is one of the largest insurance companies in the U.S., offering various types of insurance products, such as auto, home, life, and business insurance. Allstate processes over 40,000 claims per day, across 50 states and 10 countries. With such a large and complex claim volume, Allstate faces a high risk of fraud, such as claim fraud, premium fraud, and policy fraud.
To combat fraud, Allstate uses a combination of predictive analytics, data mining, and artificial intelligence to detect and prevent fraudulent claims and policies. Allstate’s fraud detection system consists of three main components:
- Claim scoring: This is the component that analyzes and scores each claim, based on the probability or likelihood of fraud, using various predictive analytics and data mining techniques and algorithms, such as regression, clustering, and association rules. The claim scoring also uses various data sources and features, such as claim history, policy details, customer profiles, and external databases.
- Image analysis: This is the component that analyzes and processes images of vehicles, buildings, or other objects that are involved in claims, using various artificial intelligence and image recognition tools and techniques, such as deep learning, convolutional neural networks, and object detection. The image analysis also helps extract relevant information, such as make, model, color, damage, or repair, and detect anomalies or inconsistencies, such as mismatched parts, altered license plates, or tampered timestamps.
- Fraud investigation: This is the component that verifies and validates the fraud detection results, using human intervention, feedback, or evidence. The fraud investigation also helps confirm or reject the fraud detection results, as well as provide insights and recommendations for further action or improvement.
What Does Allstate Benefit from Automating Fraud Detection?
By using automated fraud detection, Allstate has been able to:
- Reduce fraud losses: Allstate has reduced its fraud losses by over $100 million per year, which is equivalent to about 10% of its total claim losses.
- Improve claim efficiency: Allstate has improved its claim efficiency and accuracy, by reducing the time and resources required for claim processing and investigation, and by increasing the claim settlement and recovery rates.
- Enhance customer satisfaction: Allstate has enhanced its customer satisfaction and loyalty, by providing a fair, transparent, and timely claim service, and by rewarding honest and loyal customers.
The Future of Fraud Detection
Fraud detection is a dynamic and evolving field that requires constant adaptation and innovation to keep up with the changing fraud trends and tactics. Some of the emerging trends and technologies that are shaping the future of fraud detection are:
- Biometrics: Biometrics are physical or behavioral characteristics that can be used to identify or authenticate a person, such as fingerprints, face, voice, iris, or gait. Biometrics can help enhance fraud detection by providing a more secure and convenient way of verifying the identity of customers or users, and by preventing identity theft, spoofing, or impersonation.
- Blockchain: Blockchain is a distributed ledger technology that records and verifies transactions, activities, or data, without the need for a central authority or intermediary. Blockchain can help improve fraud detection by providing a transparent and immutable record of transactions, activities, or data, and by enabling peer-to-peer verification and validation, and smart contracts.
- Behavioral analysis: Behavioral analysis is the study of the patterns, trends, and anomalies of human behavior, such as emotions, preferences, motivations, or intentions. Behavioral analysis can help augment fraud detection by providing a deeper and richer understanding of the customers or users, and by detecting subtle or hidden signals or indicators of fraud, such as changes in behavior, mood, or tone.
The future of fraud detection is not only about using new technologies but also about using existing technologies in new and innovative ways. For example, combining machine learning with biometrics, blockchain with image recognition, or behavioral analysis with natural language processing. The future of fraud detection is also about collaborating and sharing data, insights, and best practices with other stakeholders, such as customers, partners, regulators, and law enforcement agencies.
Conclusion
Fraud is a serious and costly problem that affects every industry and sector. To combat fraud, businesses need to implement robust and effective fraud detection and prevention strategies. Automation can help businesses achieve this goal, by using advanced technologies such as machine learning, artificial intelligence, data analytics, and image recognition, to identify and prevent fraudulent transactions, activities, or behaviors, in real-time.
However, automation is not a silver bullet, and it comes with its own challenges and limitations, such as data quality and availability, data security and privacy, and human oversight and intervention. Therefore, businesses need to choose the right tools and software for automated fraud detection, based on their industry needs, budget, and data security requirements.
The future of fraud detection is bright and promising, as new and emerging technologies, such as biometrics, blockchain, and behavioral analysis, offer new opportunities and possibilities for enhancing fraud detection. The future of fraud detection is also collaborative and cooperative, as businesses need to work together with other stakeholders, such as customers, partners, regulators, and law enforcement agencies, to fight fraud and protect their data.
We hope you enjoyed reading this article and learned something new and useful about fraud detection. If you have any questions or comments on this topic, please feel free to share them with us. We would love to hear from you and learn from your feedback. Thank you for your time and attention. Stay safe and secure! 😊